Multi-Grained Attention Network With Mutual Exclusion for Composed Query-Based Image Retrieval
IEEE TRANSACTIONS ON CIRCUITS AND SYSTEMS FOR VIDEO TECHNOLOGY(2024)
摘要
The Composed Query-Based Image Retrieval (CQBIR) task aims to precisely obtain the preserved and modified parts, based on the multi-grained semantics learned from the composed query. Since the composed query includes a reference image and the modification text, not just a single modality, this task is more challenging than the general image retrieval tasks. Most previous methods attempt to learn preserved and modified parts via different attention modules and fuse them as a unified representation. However, these methods have two intrinsic drawbacks: 1) The different granular semantic information of the composed query is neglected, which results in the fact that learned preserved and modified parts are irrelevant to correct semantics. 2) The preserved and modified parts learned by previous methods have obvious overlaps, which may lead the model to obtain sub-optimal preserved and modified regions. To this end, we propose a novel method termed Multi-Grained Attention Network with Mutual Exclusion (MANME) to address the above problems. Our MANME method mainly consists of two components: 1) A multi-grained semantic construction for obtaining various textual and visual semantic information. 2) An attention with mutual exclusion constraint for reducing the degree of overlap between preserved and modified parts. It adequately utilizes the various granular semantic information and effectively refines the learned preserved and modified parts. Extensive experiments and further analyses on three widely used CQBIR datasets demonstrate that our proposed MANME method achieves new state-of-the-art performance on the CQBIR task.
更多查看译文
关键词
Semantics,Image retrieval,Task analysis,Feature extraction,Visualization,Fuses,Electronic mail,Composed query-based image retrieval,multi-grained semantic construction,mutual exclusion,preserved and modified attentions
AI 理解论文
溯源树
样例
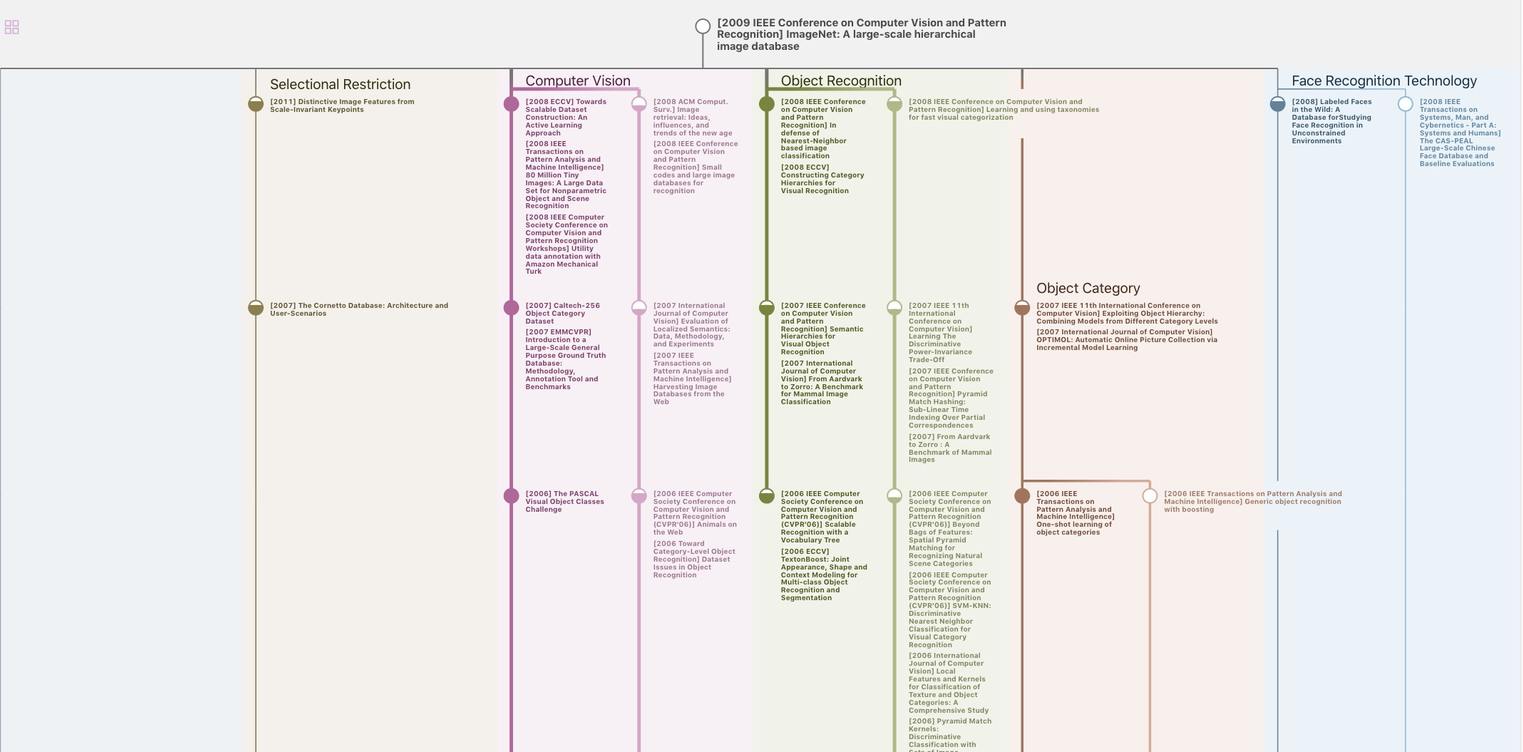
生成溯源树,研究论文发展脉络
Chat Paper
正在生成论文摘要