Fractures Extraction from Core SEM Images Based on Double-Side Inspection and Morphological Completion
All Days(2023)
摘要
ABSTRACT Accurate identification and extraction of fractures in core scanning electron microscope (SEM) images are particularly important for understanding and evaluating fractures in rocks. At present, most of the fracture extraction methods do not take into account the characteristics of fractures extension trend and edge change, resulting in many noise points and breakpoints in the extracted fractures. In view of the above problems, this paper proposes a new method of core SEM image fracture extraction based on double-side inspection and morphological completion. Double-side inspection fractures extraction grids the image and judges the micro-element containing fracture elements. There are many discontinuities in the preliminary extracted fracture image. The study presents a fractures completion algorithm based on the combination of long-short-term memory neural network (LSTM) and Similarity-matching to achieve rapid completion of the fracture discontinuities. Compared with threshold segmentation, the recall rate and precision rate of the proposed method are greatly improved. This method is the application of digital image processing and machine learning algorithm in rock fracture image processing and has important application value in rock fracture quantitative characterization and digital core establishment. INTRODUCTION Among the proven oil and gas reserves in the world, the reserves of fractured oil and gas reservoirs account for more than 60%. At present, there are many research methods for fractures reservoirs. It is an important direction of current research to extract fractures through core image recognition for analysis. The SEM scan can achieve continuous non-destructive imaging of the core, so the current core fracture extraction is mainly based on the SEM core scan image to extract fractures through image segmentation technology (Zou et al, 2016; Ma et al, 2014). At present, fracture segmentation is mainly based on threshold segmentation to binarize the core image, where the fracture area and non-fracture area show two different colors (Wang et al, 2022; Purswani et al, 2020; Zhang et al, 2017). The level set segmentation algorithm can obtain closed contour curves, so this method is also a common algorithm in image segmentation. Through this algorithm, the approximate contour curves of fractures can be obtained from core SEM images. In view of the shortcomings of the level-set algorithm, many scholars have improved it. The improvement is mainly to improve the accuracy of the segmented contour by combining other algorithms with the algorithm (Yang et al, 2012; Xu and Peng, 2012). The improvement of the image binarization algorithm can also effectively extract fractures. Pixels are divided based on the maximum inter-class variance method so that the maximum variance of different categories can effectively reduce the noise points in the image after fractures extraction (Wang, 2006). Through the Beamlet transform, the core image after threshold segmentation can effectively extract the linear features of fractures from the two-dimensional image, thus improving the accuracy of fractures extraction (Gao and Wang, 2010). With the rapid development of artificial intelligence technology, the accuracy of core image segmentation can also be improved with the help of a machine learning algorithm. Effective image segmentation can also be achieved based on BP neural network, but the convergence speed of the BP neural network is slow, so some scholars have made a series of improvements to the BP neural network through genetic algorithm, fuzzy set theory, and other methods (Zhou, 2014; Xiong et al,1999; Yang et al, 2007). The binarization of an image can also be understood as the clustering of image pixels. The pixels in the image are grouped into two categories according to their characteristics. The clustering algorithms such as support vector machine (SVM) and centerless fuzzy clustering have also been used for the binary segmentation of images (Wei et al, 2007; Ma et al, 2013). Combining clustering algorithms with threshold segmentation can effectively improve the extraction accuracy of natural fractures (Fan et al, 2022).
更多查看译文
关键词
core sem images,extraction,morphological completion,double-side
AI 理解论文
溯源树
样例
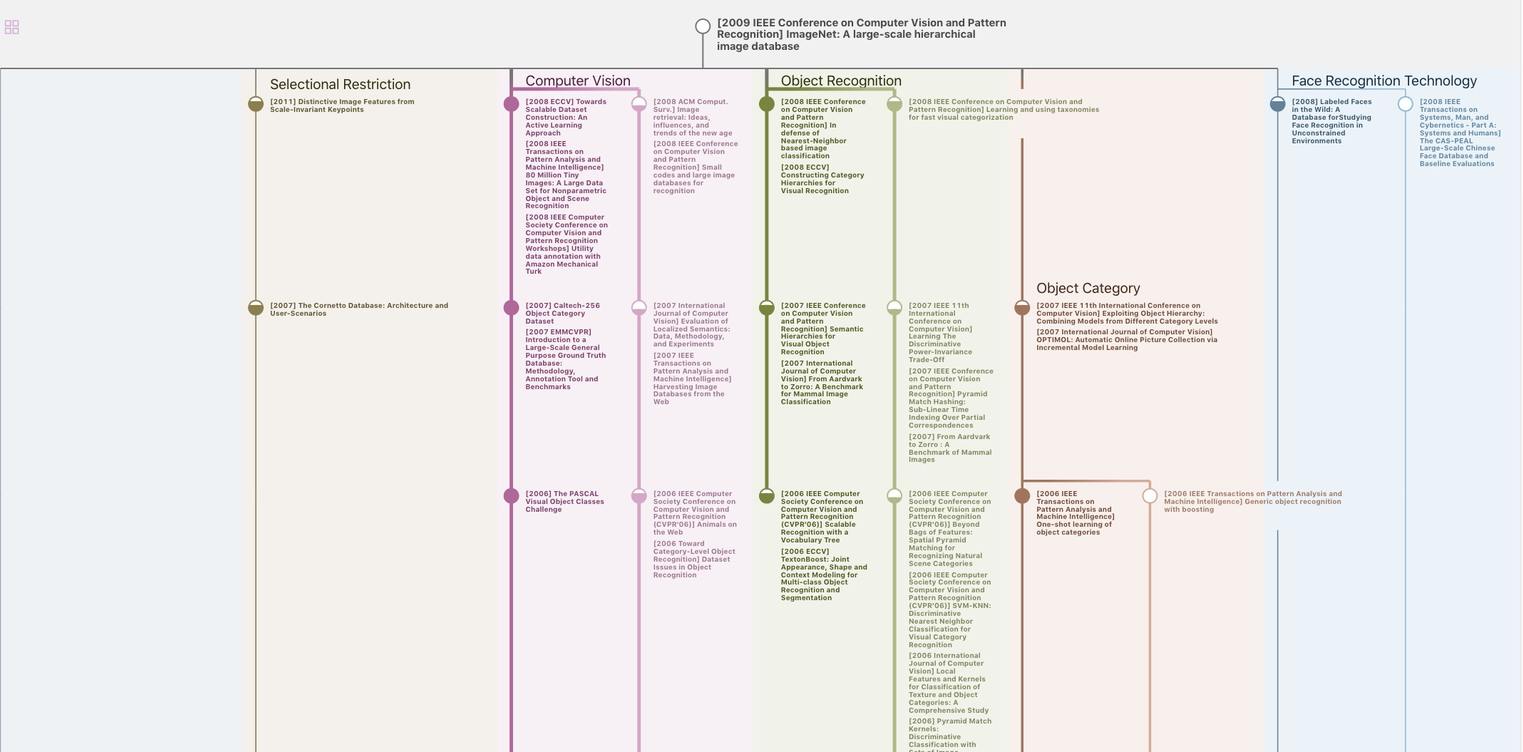
生成溯源树,研究论文发展脉络
Chat Paper
正在生成论文摘要