Light Field Super-Resolution Using Decoupled Selective Matching
IEEE Transactions on Circuits and Systems for Video Technology(2023)
摘要
Non-local self-similarity has been well exploited in the single image super-resolution task as an effective prior. However, due to the difficulty of modeling the 4D correspondence globally, the potential of the non-local prior is less revealed for light field (LF) super-resolution. Meanwhile, existing non-local models only utilize the global spatial correspondence, but largely neglect the global geometric correspondence. To address the aforementioned problems, we propose a Decoupled Selective Matching Network (DSMNet) for LF super-resolution, by designing a novel selective matching mechanism to flexibly extract non-local information from specific 4D positions in an LF. Such a mechanism matches the reference patch with several auxiliary patches dynamically searched from predefined windows, which promotes efficiency while improving performance compared to the existing non-local models. Specifically, our DSMNet decouples the whole LF into Sub-Aperture Images (SAIs) and Epipolar Plane Images (EPIs). For each SAI patch, we separately perform the selective matching inside the current SAI and cross different SAIs to exploit the global spatial correspondence efficiently. For each EPI patch, we separately perform the selective matching in EPIs of different orientations to embed robust LF geometric information into features by enhancing EPI textures, which exploits the global geometric correspondence in an efficient manner. Comprehensive experiments validate that DSMNet outperforms state-of-the-art LF super-resolution methods both quantitatively and qualitatively.
更多查看译文
关键词
Light field,Super-resolution,Decoupling,Selective matching
AI 理解论文
溯源树
样例
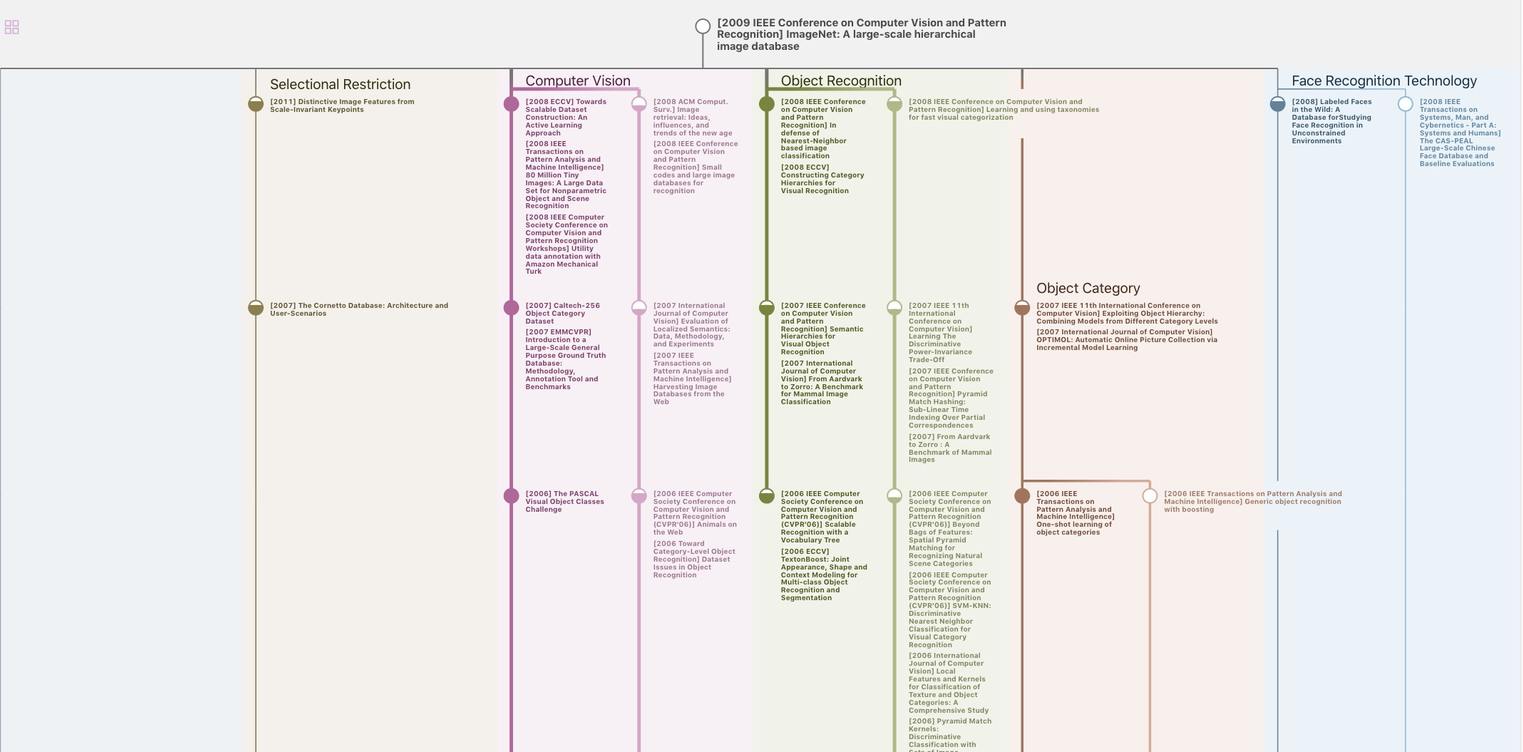
生成溯源树,研究论文发展脉络
Chat Paper
正在生成论文摘要