Deep Learning for Continuous-time Leader Synchronization in Graphical Games Using Sampling and Deep Neural Networks
ASME letters in dynamic systems and control(2023)
摘要
Abstract We propose a novel deep learning-based approach for the problem of continuous-time leader synchronization in graphical games on large networks. The problem setup is to deploy a distributed and coordinated swarm to track the trajectory of a leader while minimizing local neighborhood tracking error and control cost for each agent. The goal of our work is to develop optimal control poli- cies for continuous-time leader synchronization in graphical games using deep neural networks. We discretize the agents model using sampling to facilitate the modification of gradient descent methods for learning optimal control policies. The distributed swarm is deployed for a certain amount of time while keeping the control input of each agent constant during each sampling period. After collecting state and input data at each sampling time during one iteration, we update the weights of a deep neural network for each agent using collected data to minimize a loss function that characterizes the agents local neighborhood tracking error and the control cost. A modified gradient descent method is presented to overcome existing limitations. The performance of the proposed method is compared with two reinforcement learning-based methods in terms of robustness to initial neural network weights and initial local neighbor- hood tracking errors, and the scalability to networks with a large number of agents. Our approach has been shown to achieve superior performance compared with the other two methods.
更多查看译文
关键词
graphical games,networks,sampling,deep learning,continuous-time
AI 理解论文
溯源树
样例
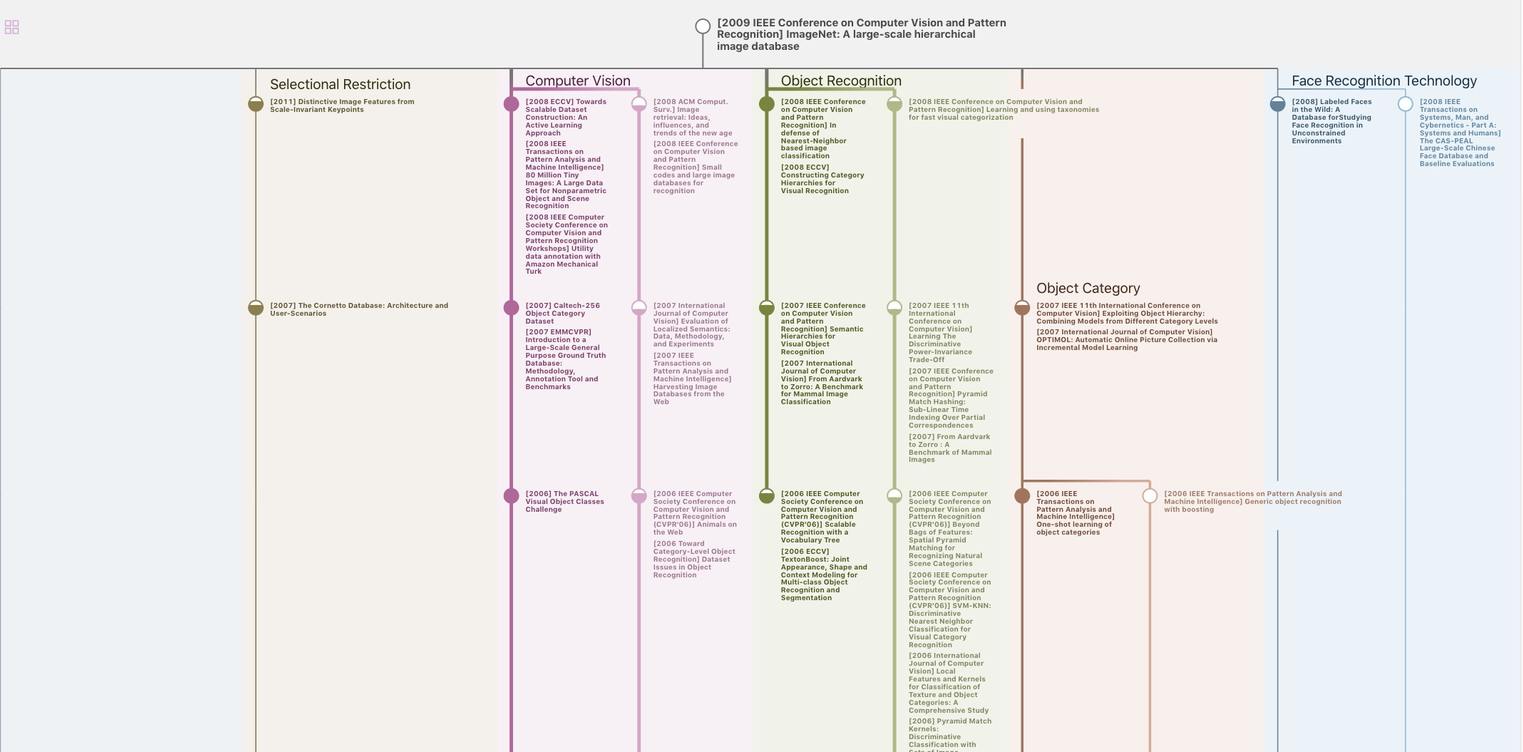
生成溯源树,研究论文发展脉络
Chat Paper
正在生成论文摘要