Predicting Nash Equilibria in Bimatrix Games using a Robust Bi-channel Convolutional Neural Network
IEEE transactions on artificial intelligence(2023)
摘要
In this paper, we consider the problem of finding a Nash equilibrium in bimatrix games. Traditional solution methods, including the Lemke-Howson (LH) algorithm and enumeration methods, rely on iterative approaches, which leads to computational inefficiency, especially when dealing with multiple instances. To address this problem, we introduce a Bi-channel Convolutional Neural Network (BiCNN) that receives a bimatrix game as an input and generates two mixed strategies as a predicted Nash equilibrium. To improve the robustness of the BiCNN model, we propose a novel training algorithm that uses bimatrix games generated from different game sizes and probability distributions. Finally, our experimental results show that the proposed approach offers a significant computational advantage while maintaining acceptable prediction accuracy Bimatrix games play an important role in fields such as finance, economics, and engineering. Traditional methods for finding the Nash equilibrium, such as the LH algorithm, suffer from computational inefficiency. This paper introduces the BiCNN approach, which overcomes this limitation. BiCNN is 5x faster than the LH algorithm when solving one instance and over 5,000x faster when solving 10,000 instances. This advance significantly improves the efficiency of solving bimatrix games, paving the way for innovations in various disciplines and industries
更多查看译文
关键词
Bimatrix game,Nash equilibrium,Convolutional neural network,Lemke-Howson algorithm
AI 理解论文
溯源树
样例
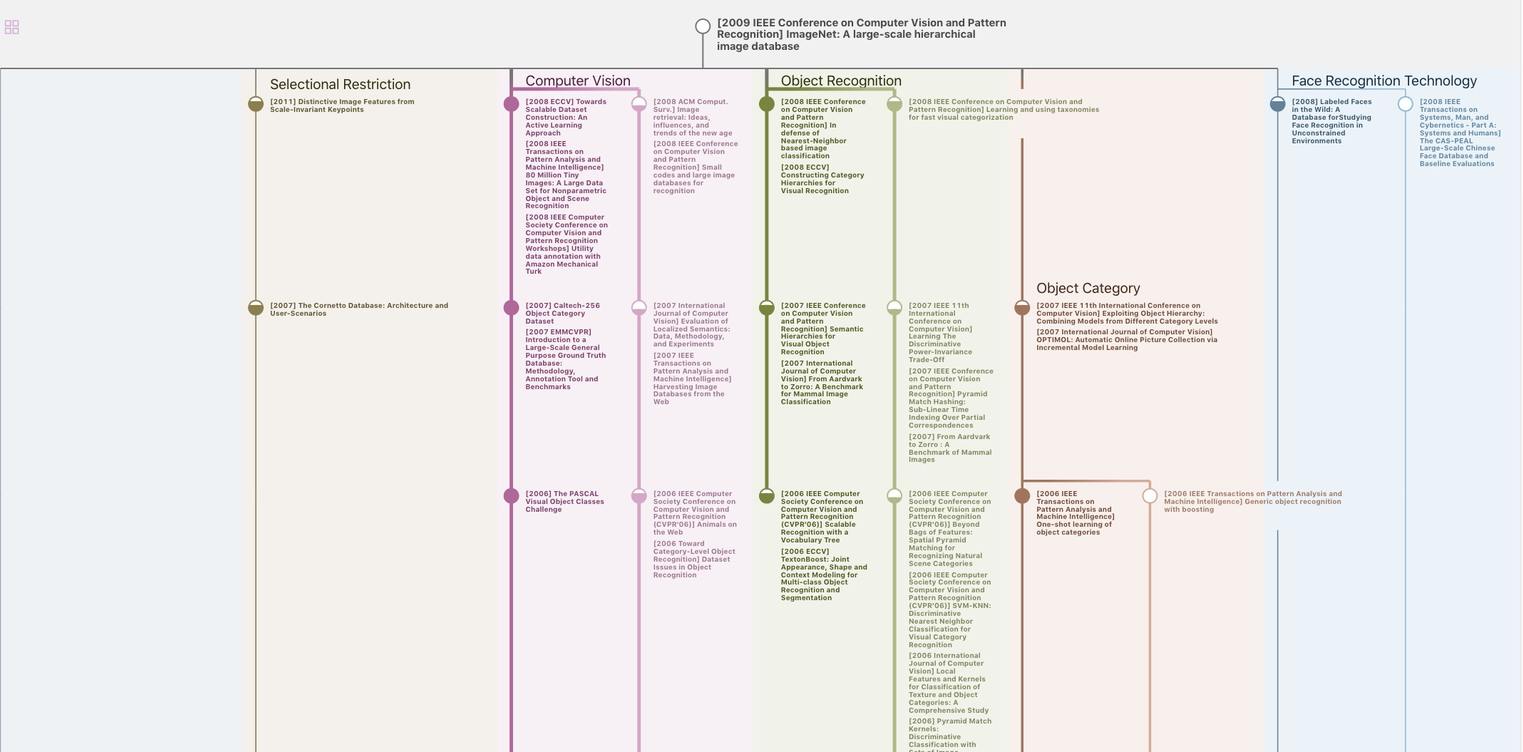
生成溯源树,研究论文发展脉络
Chat Paper
正在生成论文摘要