Towards Robust and Generalizable Training: An Empirical Study of Noisy Slot Filling for Input Perturbations
arXiv (Cornell University)(2023)
摘要
In real dialogue scenarios, as there are unknown input noises in the utterances, existing supervised slot filling models often perform poorly in practical applications. Even though there are some studies on noise-robust models, these works are only evaluated on rule-based synthetic datasets, which is limiting, making it difficult to promote the research of noise-robust methods. In this paper, we introduce a noise robustness evaluation dataset named Noise-SF for slot filling task. The proposed dataset contains five types of human-annotated noise, and all those noises are exactly existed in real extensive robust-training methods of slot filling into the proposed framework. By conducting exhaustive empirical evaluation experiments on Noise-SF, we find that baseline models have poor performance in robustness evaluation, and the proposed framework can effectively improve the robustness of models. Based on the empirical experimental results, we make some forward-looking suggestions to fuel the research in this direction. Our dataset Noise-SF will be released at https://github.com/dongguanting/Noise-SF.
更多查看译文
关键词
input perturbations,generalizable training,slot filling,noisy
AI 理解论文
溯源树
样例
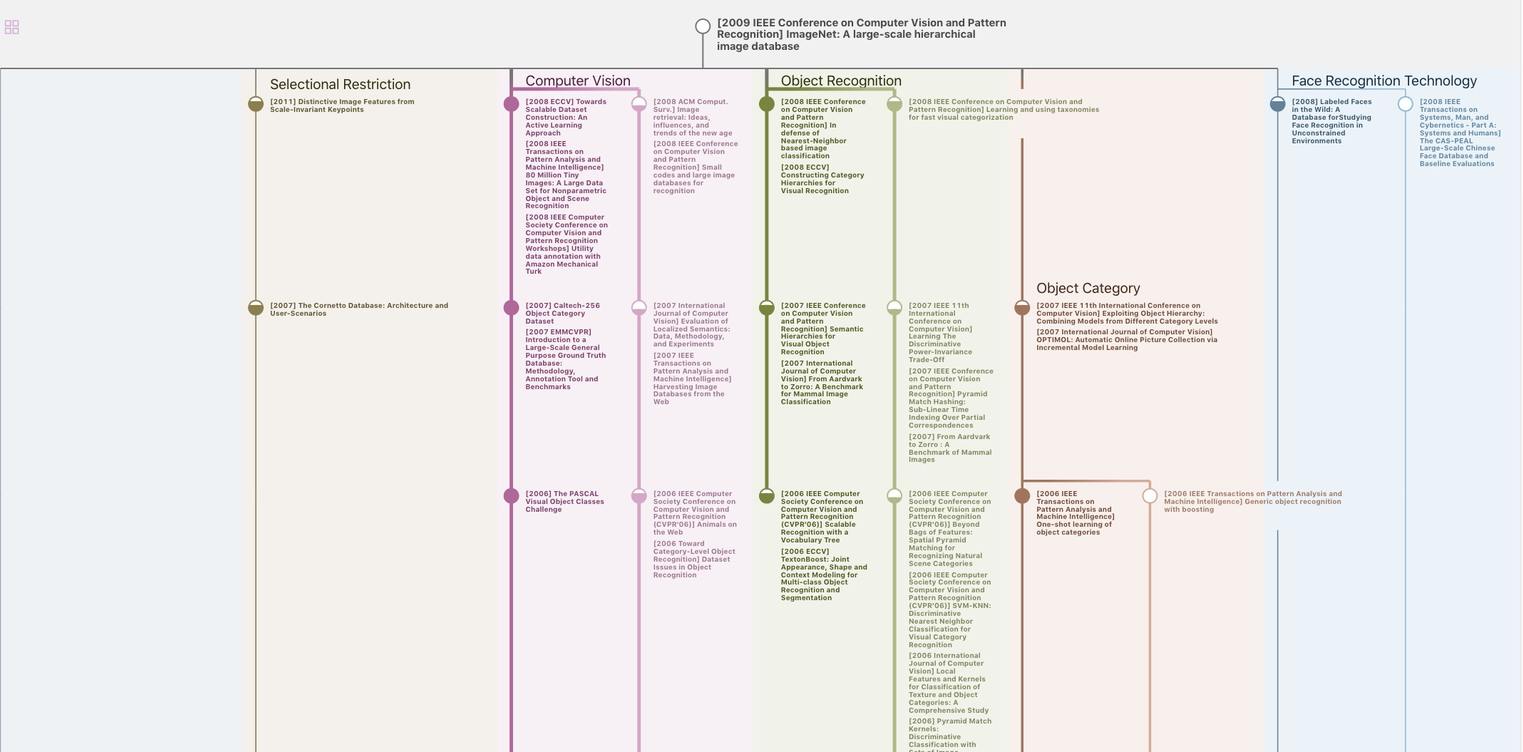
生成溯源树,研究论文发展脉络
Chat Paper
正在生成论文摘要