Sequential Learning Network With Residual Blocks: Incorporating Temporal Convolutional Information Into Recurrent Neural Networks
IEEE TRANSACTIONS ON COGNITIVE AND DEVELOPMENTAL SYSTEMS(2024)
摘要
Temporal convolutional networks (TCNs) have shown remarkable performance in sequence modeling and surpassed recurrent neural networks (RNNs) in a number of tasks. However, performing exceptionally on extremely long sequences remains an obstacle due to the restrained receptive field of temporal convolutions and a lack of forgetting mechanism. Although RNNs can carry state transmission down the full sequence length and latch information by forgetting mechanism, the issues of information saturation and gradient vanishing or exploding still persist, which usually occur in back-propagation due to the effect of multiplicative accumulation. To benefit from both temporal convolutions and RNNs, we propose a neural architecture that merge temporal convolutional data into recurrent networks. The temporal convolutions are employed intermittently and fused into the hidden states of RNNs with the assistance of attention for providing long-term information. With this architecture, it is not needed for convolutional networks to cover the total length of the sequence, thus gradient and saturation issues in RNNs are ameliorated since convolutions are integrated into its cells and the state is updated with convolutional information. Extensive experiments illustrate the superiority of our network against other competitive counterparts, such as TCNs and the different variants of RNNs.
更多查看译文
关键词
Recurrent neural networks,Computer architecture,Microprocessors,Logic gates,Convolutional neural networks,Task analysis,Kernel,Attention,long-term memory,recurrent neural network (RNN),temporal convolution,vanishing gradients
AI 理解论文
溯源树
样例
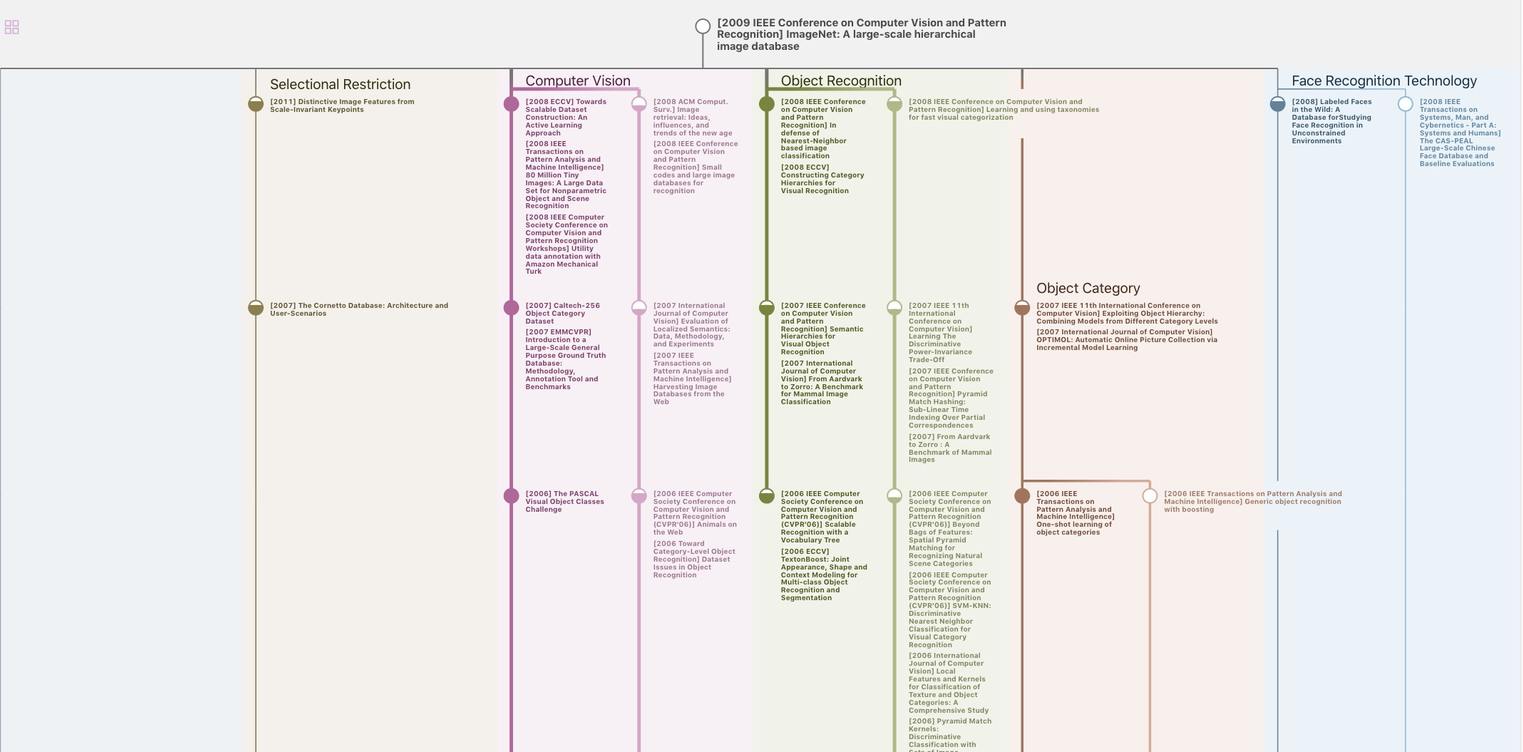
生成溯源树,研究论文发展脉络
Chat Paper
正在生成论文摘要