A Federated Deep Learning Framework for Privacy-Preserving Consumer Electronics Recommendations
IEEE Transactions on Consumer Electronics(2023)
摘要
Recommender systems (RSs) have proven to be highly effective in guiding consumers towards well-informed purchase decisions for electronics. These systems can provide personalised recommendations that consider individual preferences, past purchases and current market trends by collecting and analysing massive amounts of consumer data. However, RSs have traditionally employed centralised storage of users consumption records and item interactions, which may potentially lead to privacy concerns. In particular, centralised data storage may prove unworkable in the future with the advent of regulations such as the General Data Protection Regulation. In turn, this can lead to an urgent need for decentralised recommendation frameworks for consumer electronics. In this study, we propose a federated learning recommender system (FRS) for the recommendation task in the consumer electronics industry. However, this is rather challenging due to its privacy protection, model scalability and personalisation requirements. First, the federated recommender system for consumer electronics (FRS-CE) adopts an outer product and two proposed feature fusion operations to construct an interaction map between users and items. Second, the FRS-CE uses a lightweight convolution operation to extract high-order features from the interaction map. Finally, the proposed model employs an adaptive aggregation mechanism to update the global model, which enhances the scalability of the system. Extensive experiments conducted on two real-world datasets have demonstrated the effectiveness of the FRS-CE in generating consumer electronics recommendations with privacy protection.
更多查看译文
关键词
Data privacy,federated learning,convolutional neural network,consumer electronics recommendation
AI 理解论文
溯源树
样例
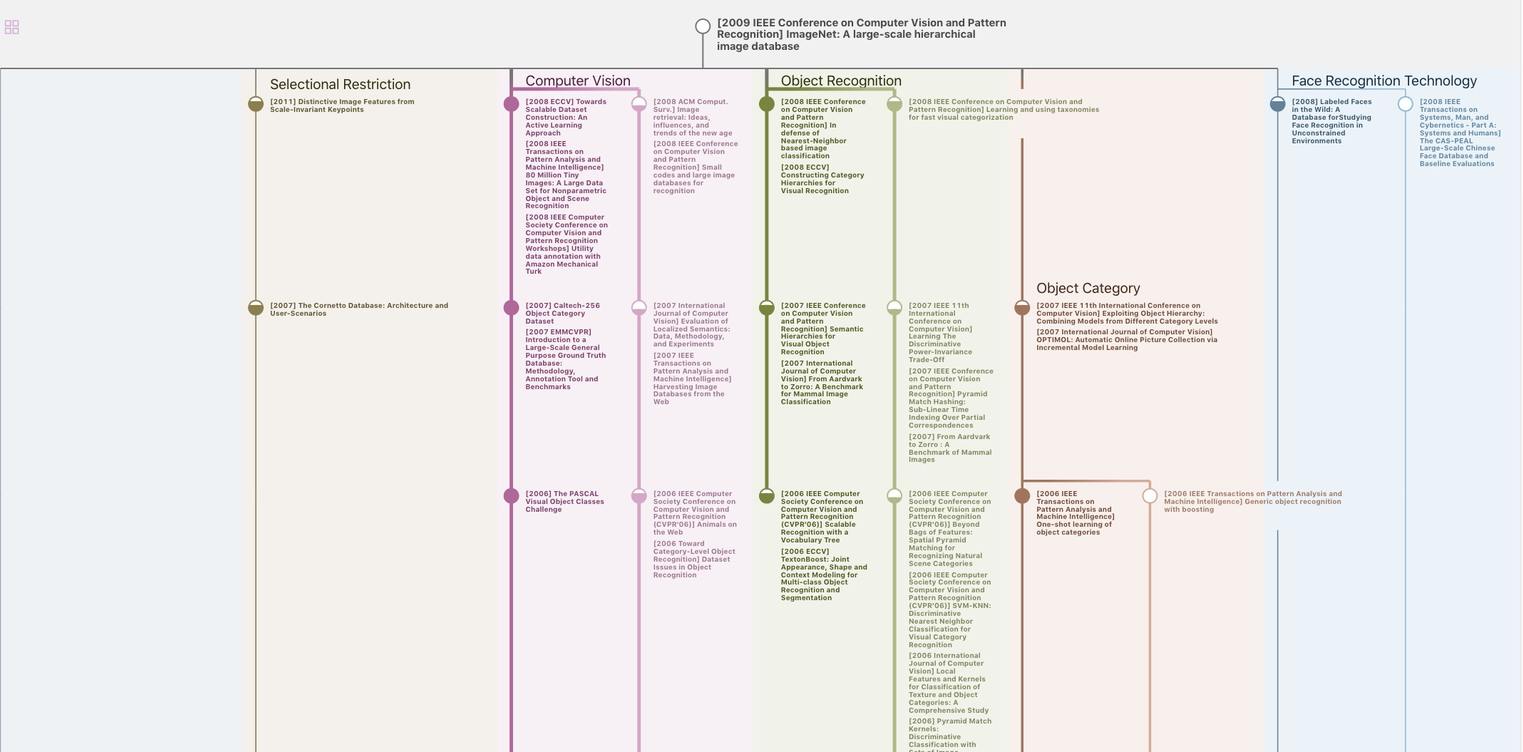
生成溯源树,研究论文发展脉络
Chat Paper
正在生成论文摘要