NASHDetection: A Natural Language Processing Method for Identifying Patients With Non-alcoholic Steatohepatitis Using Clinical Notes
AMERICAN JOURNAL OF GASTROENTEROLOGY(2023)
摘要
Introduction: Electronic Health Records (EHR) data are growing in importance as a platform to study non-alcoholic steatohepatitis (NASH). International Classification of Diseases (ICD) codes are commonly used to define EHR-based cohorts with NASH, but may result in study bias due to miscoding. Prior studies have used natural language processing (NLP) with clinical notes to identify NASH patients, but have been limited by the lack of comparisons to ICD-based methods and their unavailability as open-source algorithms to enable multicenter generalization. We sought to develop, benchmark, and share an algorithm for identifying patients with NASH using NLP. Methods: We queried EHR data at UCSF (2012-2022) to detect all patients with ≥ 1 ambulatory hepatology encounter and ≥ 1 additional clinical encounter at UCSF prior to their first hepatology encounter. We developed NASHDetection: an open-source algorithm that uses named entity recognition and regular expressions to detect assertions of NASH diagnoses from the assessment and plan (A&P) section of hepatology clinical notes. We sampled and manually reviewed 60 algorithm-predicted NASH cases and 60 non-cases to establish a test set and quantify accuracy. Our gold-standard for defining cases was ≥ 1 hepatology notes asserting a NASH diagnosis. We used this test set to compare our approach with other commonly used methods: ≥1 NASH ICD code by any provider, ≥1 NASH ICD code by a hepatologist, ≥1 NASH ICD code and mention of “NASH” in a hepatology note. Results: We identified 82,055 hepatology notes with an A&P section. After applying the inclusion criteria and using NASHDetection, we isolated 1,677 patients with algorithm-predicted NASH diagnoses (cases). Predicted non-cases were the 14,756 patients with hepatology notes who met inclusion but did not have an algorithm-predicted NASH diagnosis. Our method was 93% accurate relative to the results of manual review, with a sensitivity and specificity of 93% and 92% respectively (Table 1). Comparing our approach against simpler methods for defining a NASH cohort, we found that notes-based methods were significantly more accurate than ICD-based methods (P = 0.01). Conclusion: NLP can improve the accuracy of identifying NASH patients within the EHR and reduce potential bias in retrospective studies. NASHDetection is available at github.com/rwelab/NASHDetection. With minor modifications, our algorithm allows for potential generalizability to other centers, and may support future efforts to risk stratify these patients. Table 1. - Comparison of various cohort identification approaches Method Accuracy PPV NPV Sensitivity Specificity ICD codes 73 [64-81] 71 [59-82] 76 [62-87] 78 [66-88] 68 [55-80] ICD codes a/w hepatology encounters 75 [66-82] 73 [61-84] 77 [64-87] 78 [66-88] 72 [59-83] ICD codes + hepatology encounters + keyword match 86 [78-92] 92 [81-98] 81 [70-90] 78 [66-88] 92 [81-98] Text classifier (our approach) 93 [86-97] 92 [83-96] 93 [84-97] 93 [84-98] 92 [82-97] The best performing model (by metric) is underlined, 95% confidence intervals are in brackets. All numbers correspond to percentages. PPV = positive predictive value. NPV = negative predictive value.
更多查看译文
AI 理解论文
溯源树
样例
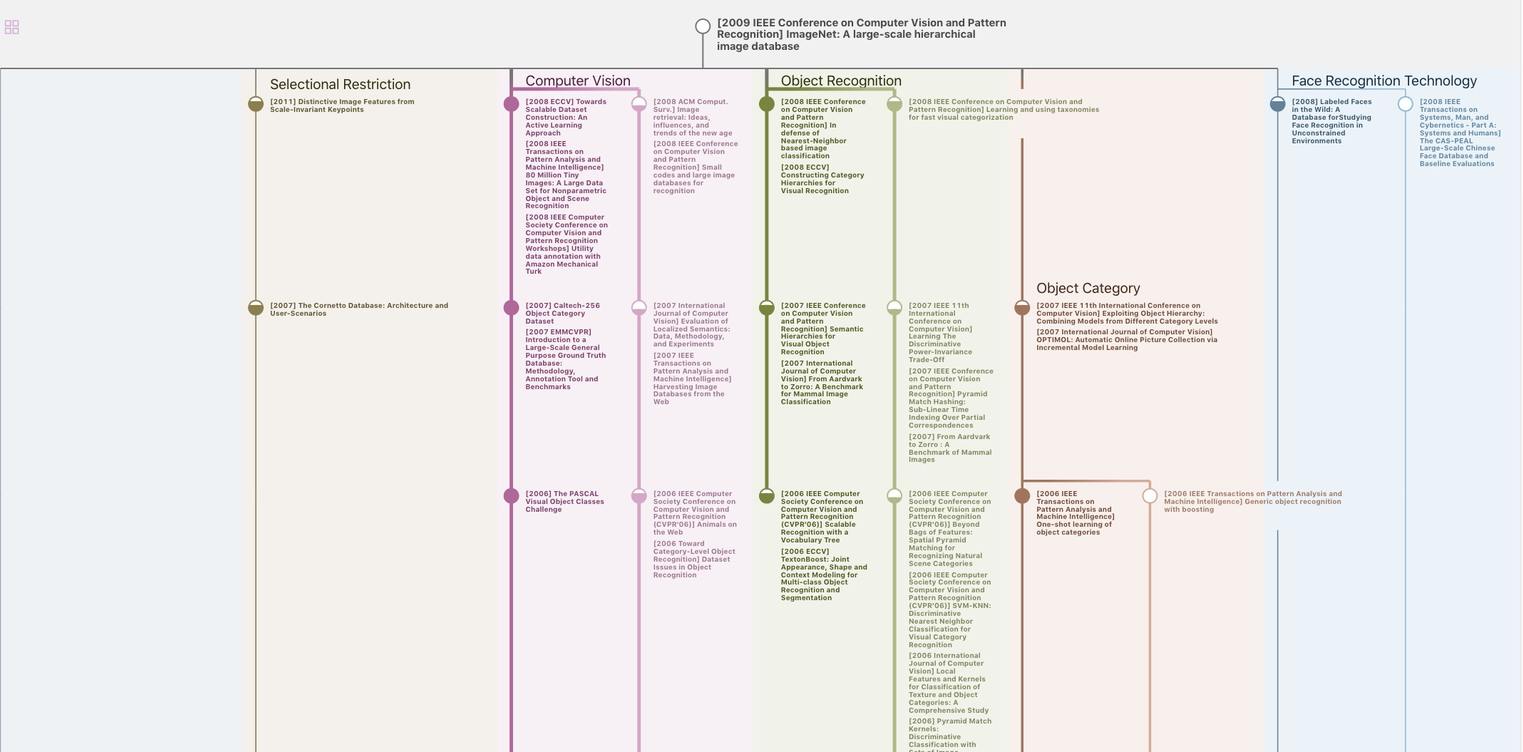
生成溯源树,研究论文发展脉络
Chat Paper
正在生成论文摘要