Graph Fuzzy System for the Whole Graph Prediction: Concepts, Models, and Algorithms
IEEE TRANSACTIONS ON FUZZY SYSTEMS(2024)
摘要
Fuzzy systems (FSs) have been widely utilized in diverse domains, such as pattern recognition, intelligent control, data mining, and bioinformatics due to their strong interpretation and learning abilities. Traditionally, FSs have mainly been applied to model Euclidean data. However, with the emergence of scenarios involving graph data, such as social networks and traffic route maps, which inherently possess non-Euclidean structures, there is a need to develop FS modeling methods suitable for graph data while retaining the advantages of traditional FSs. This article presents a novel FS called graph fuzzy system (GFS) specifically designed for modeling whole graph data. The concepts, modeling framework, and construction algorithms are systematically developed. First, the article defines GFS-related concepts, including the graph fuzzy rule base, graph fuzzy sets, and graph consequent processing unit (GCPU). Second, the learning framework for GFS is proposed. It includes a novel K-Means with graph similarity measure clustering approach (KM-GSM) for generating antecedents in GFS and a new consequent parameters learning algorithm based on graph neural network (GNN). Moreover, three different versions of the GFS implementation algorithm are developed and thoroughly evaluated through experiments on various graph prediction datasets. The results demonstrate that the proposed GFS inherits the advantages of mainstream GNNs methods and conventional FSs methods while achieving superior performance in whole graph prediction compared to existing approaches.
更多查看译文
关键词
Data models,Kernel,Fuzzy systems,Fuzzy sets,Task analysis,Graph neural networks,Uncertainty,Graph consequent processing unit (GCPU),graph fuzzy rule base,graph fuzzy system (GFS),k-means with graph similarity measure clustering (KM-GSM),whole graph prediction
AI 理解论文
溯源树
样例
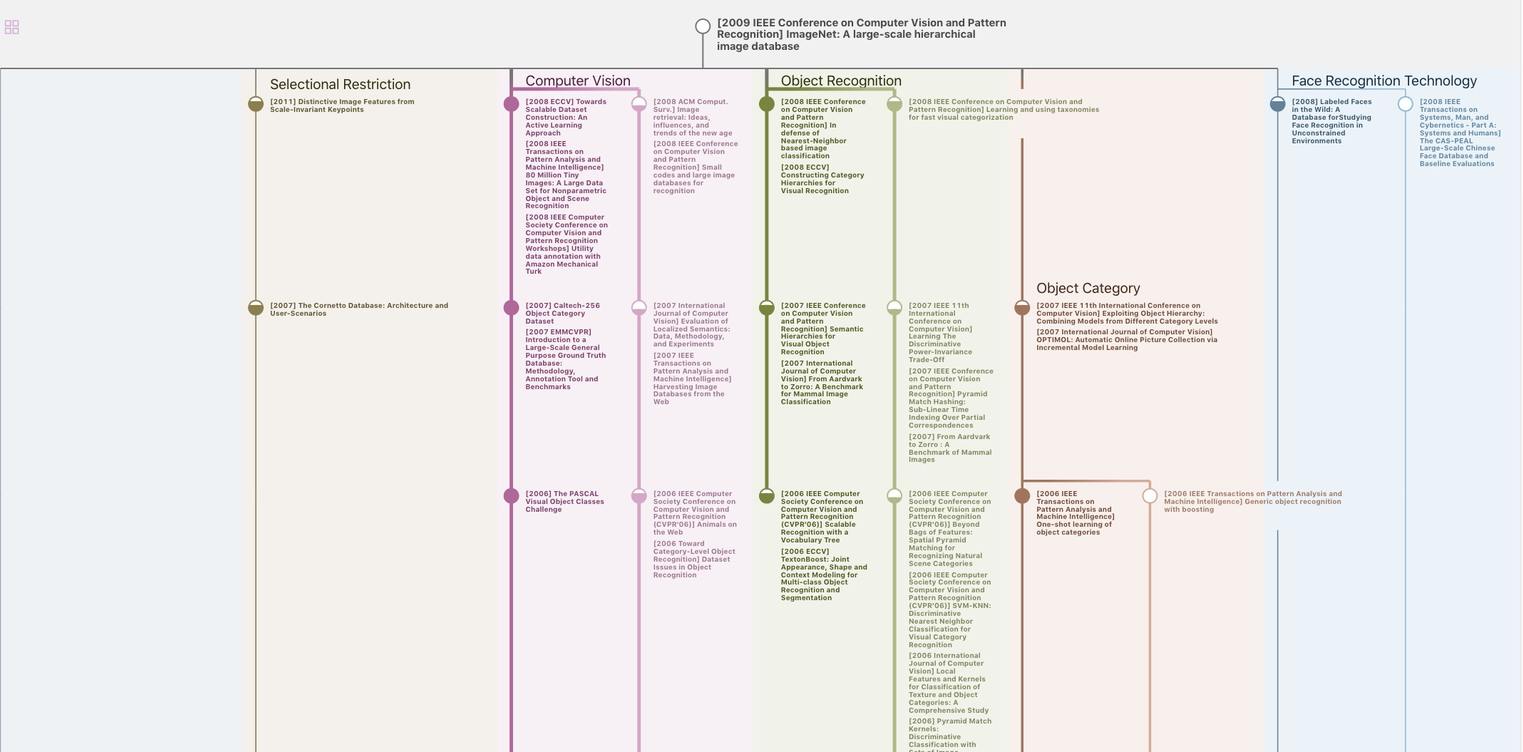
生成溯源树,研究论文发展脉络
Chat Paper
正在生成论文摘要