Channel Pruning Method for Signal Modulation Recognition Deep Learning Models.
IEEE Trans. Cogn. Commun. Netw.(2024)
摘要
Automatic modulation recognition (AMR) plays an important role in communication system. With the expansion of data volume and the development of computing power, deep learning framework shows great potential in AMR. However, deep learning models suffer from the heavy resource consumption problem caused by the huge amount of parameters and high computational complexity, which limit their performance in scenarios that require fast response. Therefore, the deep learning models must be compressed and accelerated, where channel pruning is an effective method to reduce the amount of computation and can speed up models inference. In this paper, we propose a new channel pruning method suitable for AMR deep learning models. We consider both the channel redundancy of the convolutional layer and the channel importance measured by the γ scale factor of the batch normalization (BN) layer. Our proposed method jointly evaluates the model channels from the perspectives of structural similarity and numerical value, and generates evaluation indicators for selecting channels. This method can prevent cutting out important convolutional layer channels. And combined with other strategies such as one-shot pruning strategy and local pruning strategy, the model classification performance can be guaranteed further. We demonstrate the effectiveness of our approach on a variety of different AMR models. Compared with other classical pruning methods, the proposed method can not only better maintain the classification accuracy, but also achieve a higher compression ratio. Finally, we deploy the pruned network model to edge devices, validating the significant acceleration effect of our method.
更多查看译文
关键词
automatic modulation recognition,deep learning,neural network model pruning,edge devices
AI 理解论文
溯源树
样例
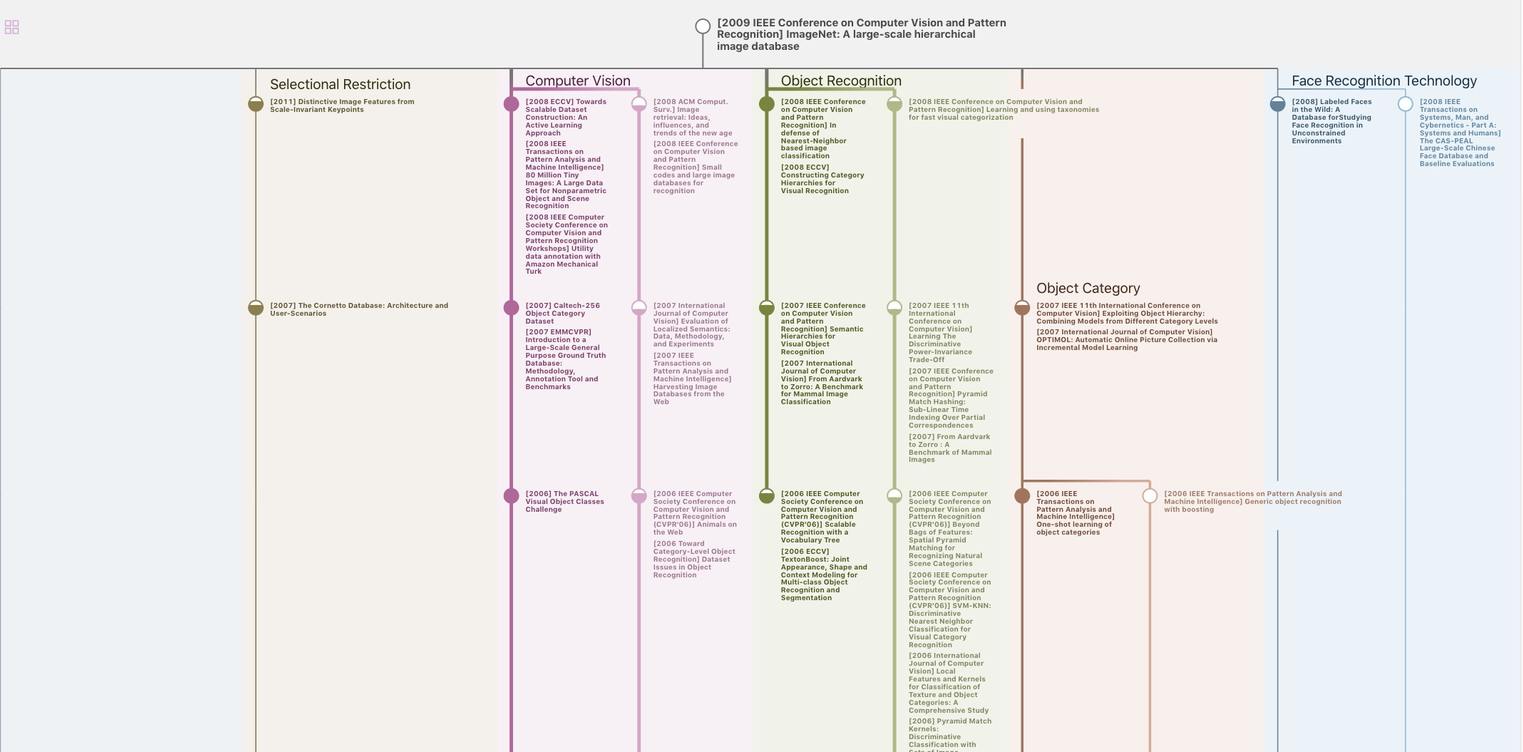
生成溯源树,研究论文发展脉络
Chat Paper
正在生成论文摘要