Evolutionary Pareto Set Learning with Structure Constraints
arXiv (Cornell University)(2023)
摘要
The multiobjective evolutionary optimization algorithm (MOEA) is a powerful approach for tackling multiobjective optimization problems (MOPs), which can find a finite set of approximate Pareto solutions in a single run. However, under mild regularity conditions, the Pareto optimal set of a continuous MOP could be a low dimensional continuous manifold that contains infinite solutions. In addition, structure constraints on the whole optimal solution set, which characterize the patterns shared among all solutions, could be required in many real-life applications. It is very challenging for existing finite population based MOEAs to handle these structure constraints properly. In this work, we propose the first model-based algorithmic framework to learn the whole solution set with structure constraints for multiobjective optimization. In our approach, the Pareto optimality can be traded off with a preferred structure among the whole solution set, which could be crucial for many real-world problems. We also develop an efficient evolutionary learning method to train the set model with structure constraints. Experimental studies on benchmark test suites and real-world application problems demonstrate the promising performance of our proposed framework.
更多查看译文
关键词
constraints,set,learning,structure
AI 理解论文
溯源树
样例
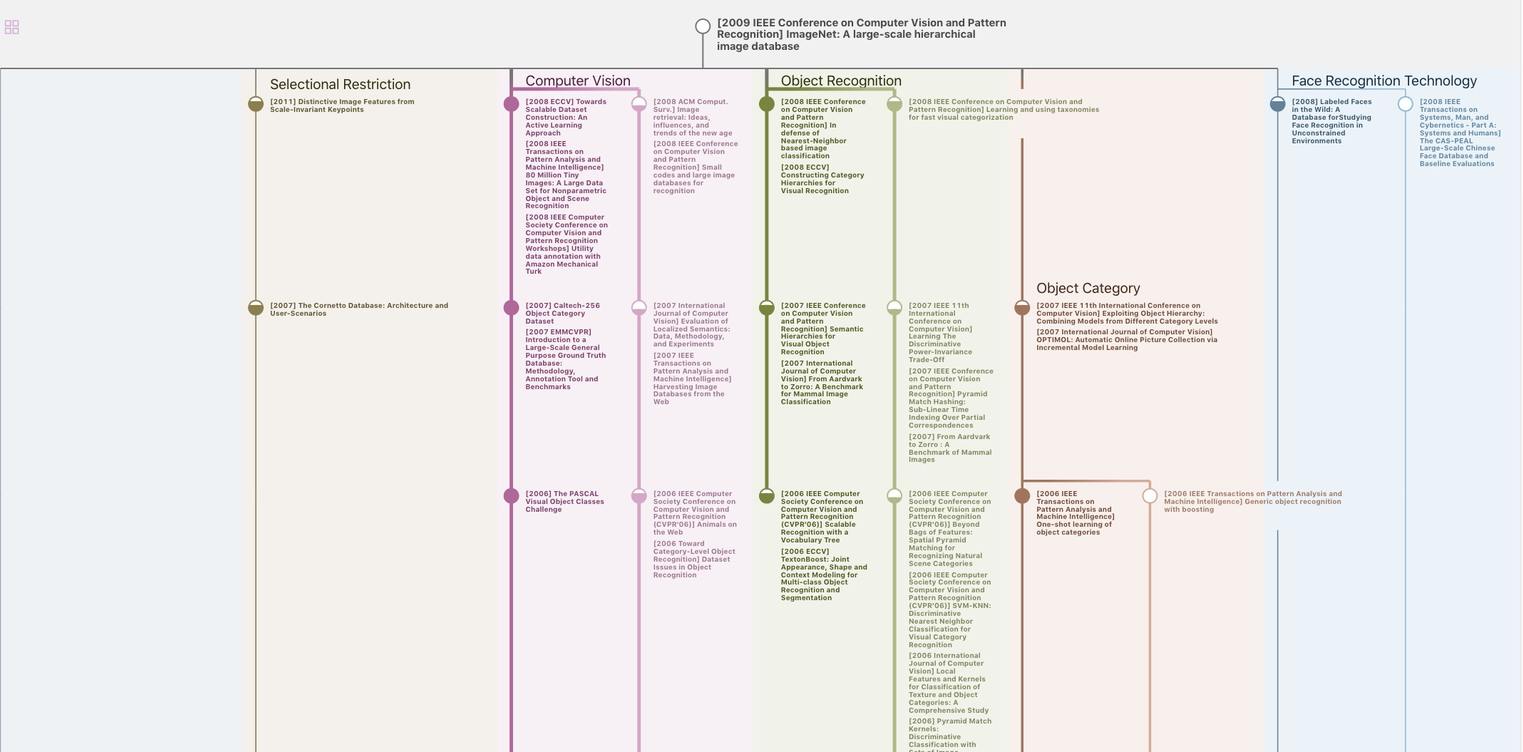
生成溯源树,研究论文发展脉络
Chat Paper
正在生成论文摘要