CoLive: Edge-Assisted Clustered Learning Framework for Viewport Prediction in 360$^\circ$ Live Streaming
IEEE Transactions on Multimedia(2023)
摘要
The exceptionally high bandwidth requirement for delivering high-quality live 360 $^\circ$ video poses a significant challenge to current network capacity. Mitigating such bandwidth starvation necessitates accurate field-of-view (FoV) prediction to focus limited resources on the viewer's area of interest. However, FoV prediction for live 360 $^\circ$ streaming can be complex due to the time-sensitive nature of live content and the limited knowledge available for model training. Our paper introduces a novel framework, CoLive , for predicting the FoV in 360 $^\circ$ live streaming. CoLive accelerates FoV prediction by offloading model training from viewers to the edge and migrating saliency feature detection to the server side. Observations on user clustering of viewing behaviors further motivate us to propose a novel dynamic clustered learning algorithm. The algorithm dynamically groups users according to their model update gradients and enables them to train a shared model that better suits their viewing preferences. We conduct extensive experiments on the public 360 $^\circ$ video datasets and demonstrate that CoLive outperforms state-of-the-art solutions in terms of prediction performance and bandwidth savings.
更多查看译文
关键词
viewport prediction,learning,edge-assisted
AI 理解论文
溯源树
样例
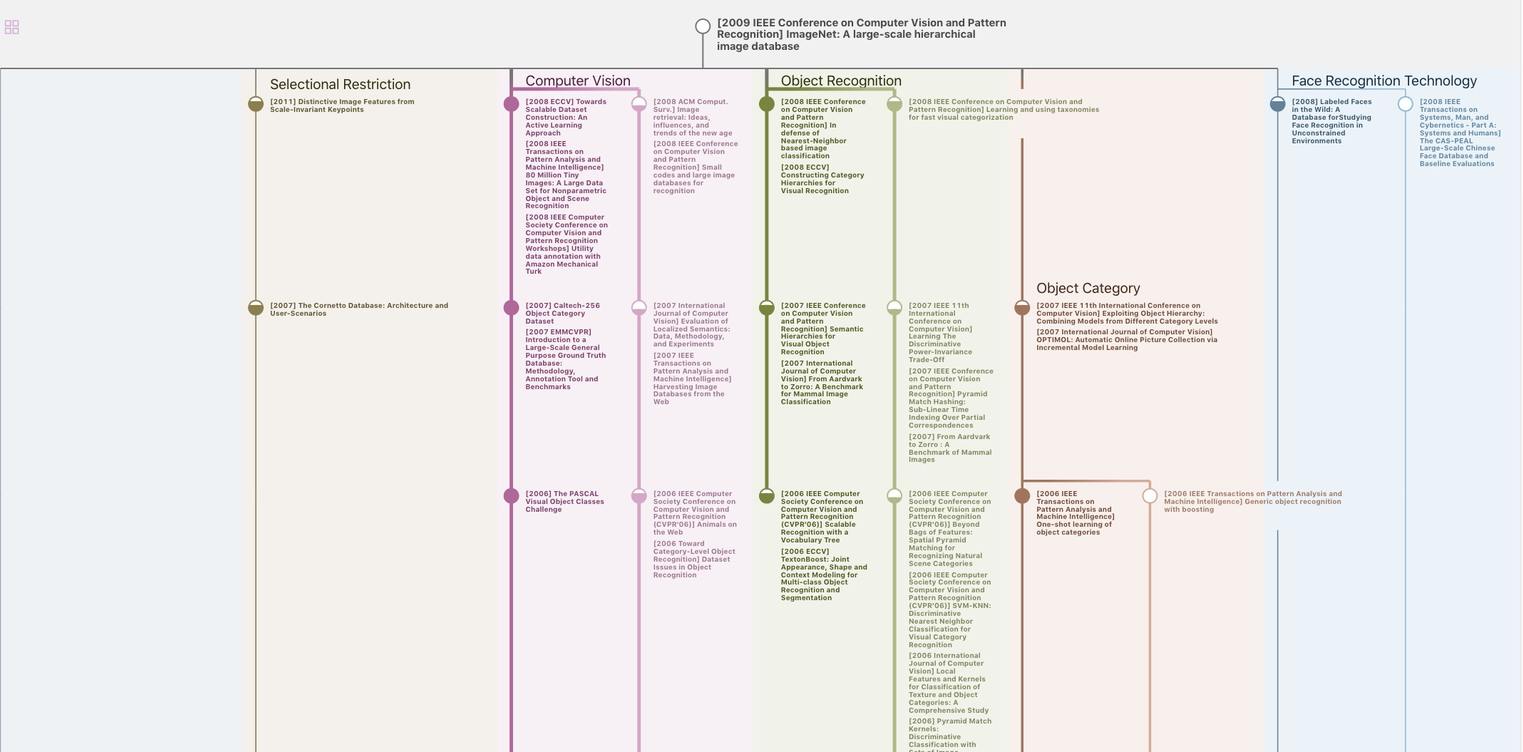
生成溯源树,研究论文发展脉络
Chat Paper
正在生成论文摘要