Design of Contextual Filtered Features for Better Smartphone-User Receptivity Prediction
IEEE INTERNET OF THINGS JOURNAL(2024)
摘要
For the successful engagement of users with incoming interventions, the delivery of just-in-time (JIT) (or opportune moment-OM) interventions is of the utmost importance. This can be accomplished with a machine learning (ML) model that utilizes user context data. Smartwatch and smartphone interactions further open the space for further improvement by considering the user's state and environmental information. In this work, a novel feature engineering approach for predicting smartphone user receptivity to JIT interventions is proposed. Proposed approach utilizes rich information from user-smartphone interactions and smartwatch sensor data to extract contextually filtered features (CFFs). The superiority of the proposed feature engineering method is demonstrated by developing an ML model that predicts a participant's receptivity prior to administering an experience sampling method (ESM) questionnaire. The proposed approach is evaluated using the KEmoPhone data set, which contains 3334 ESM answers collected over the course of one week from 73 participants. To reduce the bias that may result in selecting specific classifier, multiple classifiers are tested and their average ROC-AUC metrics are compared. The results show that the proposed feature engineering method-CFFs improves the prediction performance, achieving an ROC-AUC of 56.5% as opposed to 54.7% obtained using conventional features (statistical moments of time series over an 80 min window). Such superior performance is consistent across multiple ML classification algorithms. Full research code will be released in Jupyter Notebooks for facilitating the research in the domain at https://github.com/Jumabek/receptivity upon the publication of this research work.
更多查看译文
关键词
Feature extraction,Measurement,Predictive models,Data mining,Machine learning,Internet of Things,Data models,Context-aware services,intelligent sensors,machine learning,ubiquitous computing
AI 理解论文
溯源树
样例
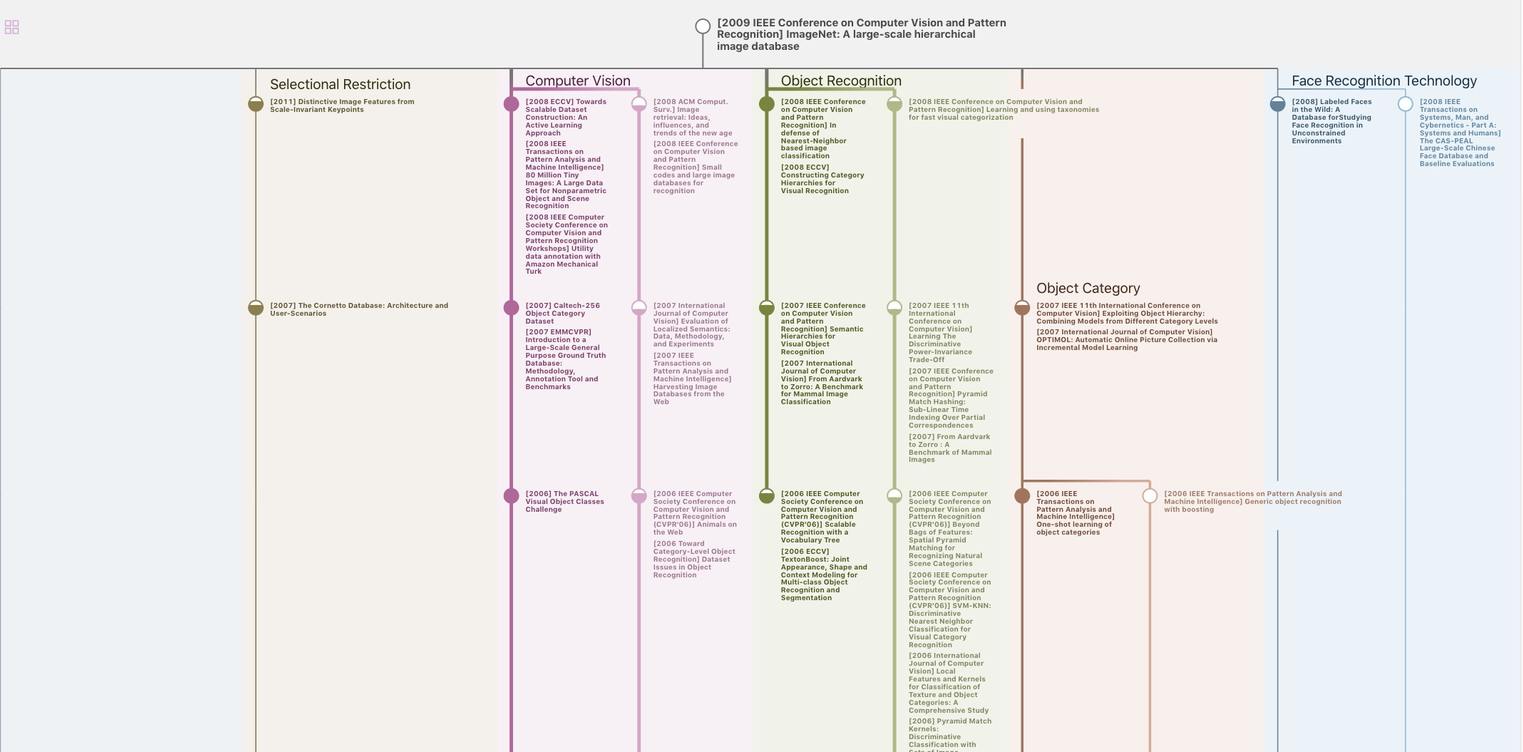
生成溯源树,研究论文发展脉络
Chat Paper
正在生成论文摘要