Operational Status Evaluation of Smart Electricity Meters Using Gaussian Process Regression With Optimized-ARD Kernel
IEEE TRANSACTIONS ON INDUSTRIAL INFORMATICS(2024)
摘要
Operational Status Evaluation (OSE) is essential for the health management of Smart Electricity Meters (SEM). This paper develops a machine learning-enabled OSE method for SEM. Specifically, the Gaussian Process Regression (GPR) is developed for modeling analysis, where an Optimized Automatic Relevance Determination (OARD) kernel is first used. Though the conventional ARD kernel structure can capture the potential mapping relationship between running time, temperature, humidity, and Measurement Error (ME), it cannot extract the influence degree of different stresses on the ME. To address this problem, an OARD kernel structure is proposed to identify the contribution of each part in ARD structure to the target data. Furthermore, the quartiles line instead of 95% confidence interval is exploited to enhance the performance of GPR for long-term prediction. Combining the two above improvements, a novel OSE model is established for the future-oriented long-term operation of the SEM. It is the first-known data-driven application that utilizes the GPR with OARD kernel to perform OSE for SEM. Actual SEM datasets collected from both dry and hot region are used for model validation and prediction. The results demonstrate that the proposed GPR model with OARD Matern52 kernel outperforms other conventional kernel approaches in the aspect of interpretability. More importantly, the operational status of the SEM in future can be assessed via the proposed OSE framework.
更多查看译文
关键词
Data driven,gaussian process regression,operational status evaluation,OARD kernel structure,smart electricity meter.
AI 理解论文
溯源树
样例
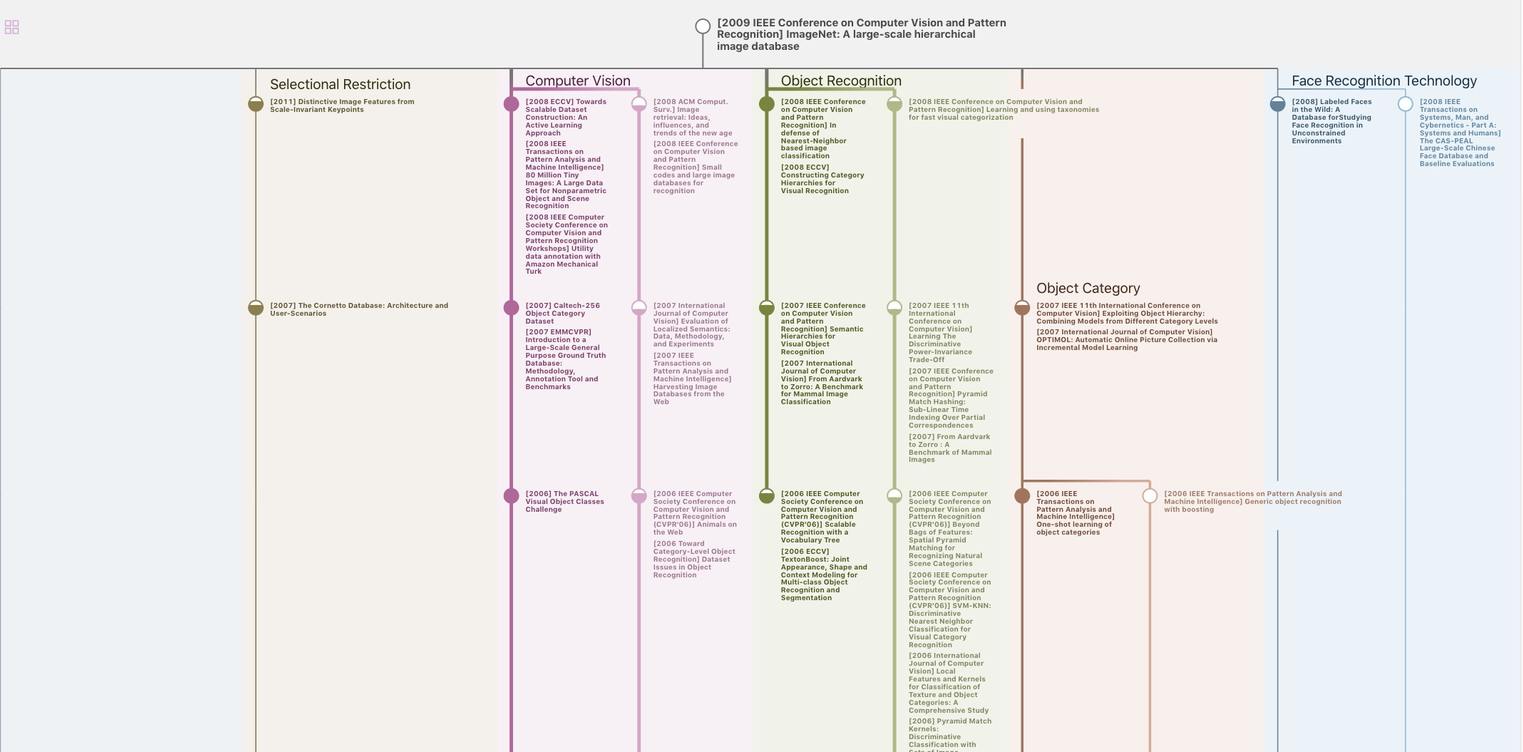
生成溯源树,研究论文发展脉络
Chat Paper
正在生成论文摘要