Observer-Based Event-Triggered Iterative Learning Consensus for Locally Lipschitz Nonlinear MASs
IEEE TRANSACTIONS ON COGNITIVE AND DEVELOPMENTAL SYSTEMS(2024)
摘要
This article aims to realize the robust tracking for nonidentical locally Lipschitz nonlinear multiagent systems (MASs) with unmeasurable states, for which an observer-based distributed event-triggered iterative learning control (ILC) framework is proposed. With this framework, distributed state observers provide the indispensable state information for agents to learn to complete the task. An initial state learning strategy is implemented to relax consistent initial conditions, which can improve the learning accuracy. An event-triggering mechanism is designed to reduce the occupation of communication and computing resources during the iterative learning process of MASs. With locally Lipschitz nonlinearities and iteration-varying uncertainties caused by nonrepetitive initial states, the double-dynamics analysis (DDA) method is adopted to illustrate the convergence of the ILC process. By setting appropriate learning gains and constructing a quasi-globally Lipschitz condition via the DDA method, the robust convergence can be achieved for MASs in the presence of switching topologies. A numerical simulation is used to show the validity of the proposed framework.
更多查看译文
关键词
Topology,Observers,Switches,Uncertainty,Temperature measurement,Laplace equations,Heuristic algorithms,Distributed state observers,event-triggered iterative learning control (ILC),nonidentical locally Lipschitz nonlinear multiagent systems (MASs),switching topologies
AI 理解论文
溯源树
样例
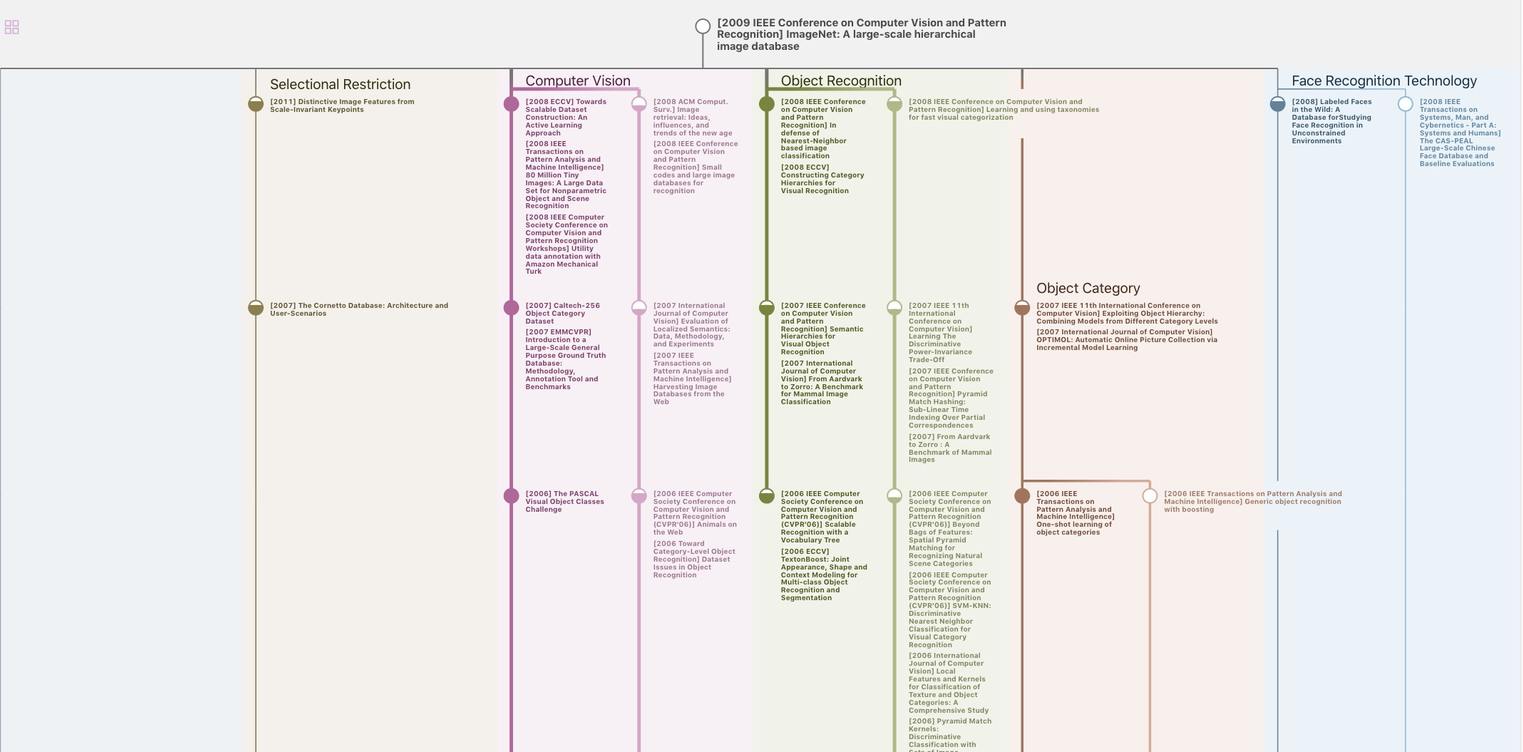
生成溯源树,研究论文发展脉络
Chat Paper
正在生成论文摘要