Comparative Deep Learning Studies for Indirect Tunnel Monitoring with and Without Fourier Pre-Processing.
Integrated computer-aided engineering(2024)
摘要
In the last decades, the majority of the existing infrastructure heritage is approaching the end of its nominal design life mainly due to aging, deterioration, and degradation phenomena, threatening the safety levels of these strategic routes of communications. For civil engineers and researchers devoted to assessing and monitoring the structural health (SHM) of existing structures, the demand for innovative indirect non-destructive testing (NDT) methods aided with artificial intelligence (AI) is progressively spreading. In the present study, the authors analyzed the exertion of various deep learning models in order to increase the productivity of classifying ground penetrating radar (GPR) images for SHM purposes, especially focusing on road tunnel linings evaluations. Specifically, the authors presented a comparative study employing two convolutional models, i.e. the ResNet-50 and the EfficientNet-B0, and a recent transformer model, i.e. the Vision Transformer (ViT). Precisely, the authors evaluated the effects of training the models with or without pre-processed data through the bi-dimensional Fourier transform. Despite the theoretical advantages envisaged by adopting this kind of pre-processing technique on GPR images, the best classification performances have been still manifested by the classifiers trained without the Fourier pre-processing.
更多查看译文
关键词
Convolutional neural networks,transformer,Fourier transforms,ground penetrating radar systems,nondestructive examination
AI 理解论文
溯源树
样例
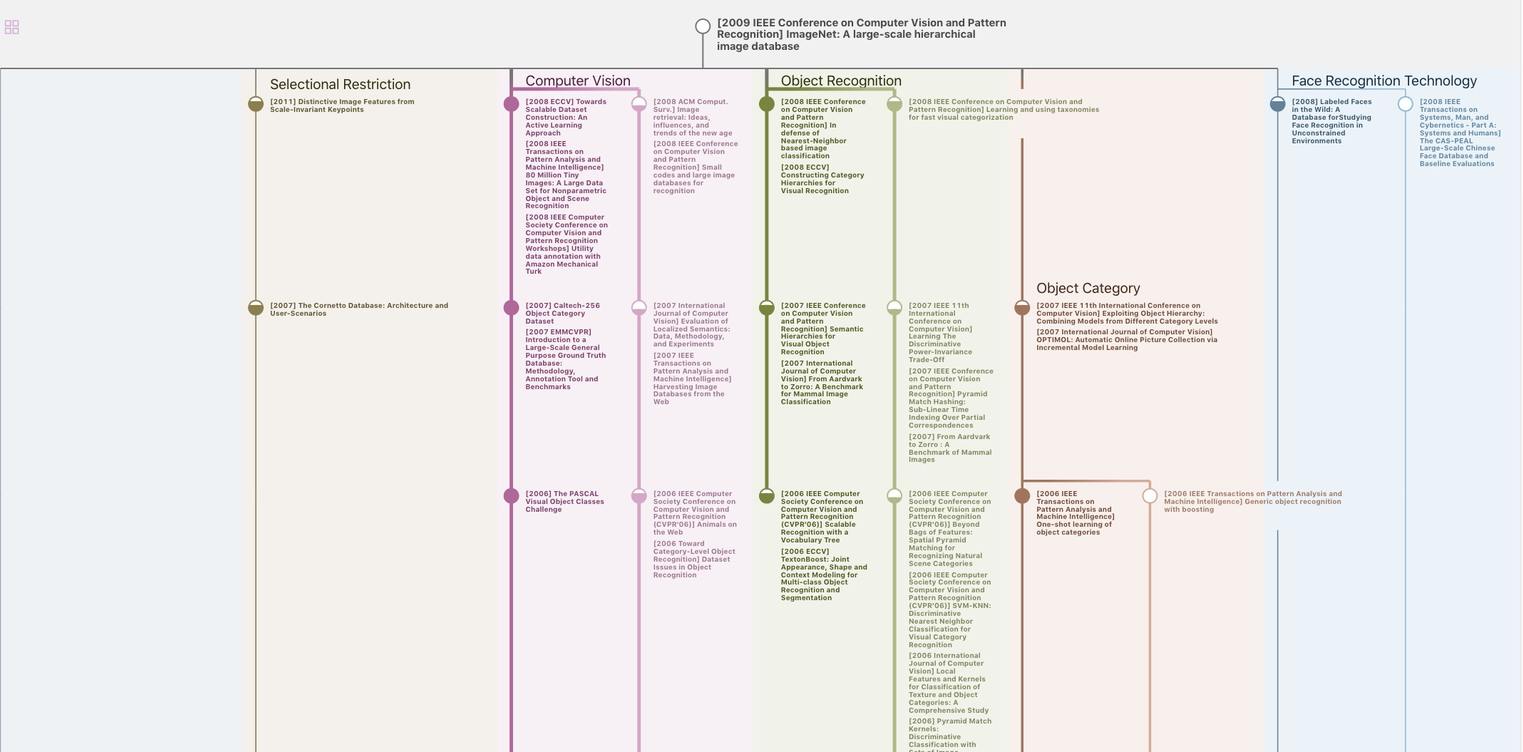
生成溯源树,研究论文发展脉络
Chat Paper
正在生成论文摘要