Deep-learning Based Pathological Assessment of Frozen Procurement Kidney Biopsies Predicts Graft Loss and Guides Organ Utilization: A Large-scale Retrospective Study
bioRxiv (Cold Spring Harbor Laboratory)(2023)
摘要
Abstract Background Lesion scores on procurement donor biopsies are commonly used to guide organ utilization. However, frozen sections present challenges for histological scoring, leading to inter- and intra-observer variability and inappropriate discard. Methods We constructed deep-learning based models to recognize kidney tissue compartments in H&E stained sections from procurement biopsies performed at 583 hospitals nationwide in year 2011-2020. The models were trained and tested respectively on 11473 and 3986 images sliced from 100 slides. We then extracted whole-slide abnormality features from 2431 kidneys, and correlated with pathologists’ scores and transplant outcomes. Finally, a Kidney Donor Quality Score (KDQS) incorporating digital features and the Kidney Donor Profile Index (KDPI) was derived and used in combination with recipient demographic and peri-transplant characteristics to predict graft loss or assist organ utilization. Results Our model accurately identified 96% and 91% of normal/sclerotic glomeruli respectively; 94% of arteries/arterial intimal fibrosis regions; 90% of tubules. Three whole-slide features (Sclerotic Glomeruli%, Arterial Intimal Fibrosis%, and Interstitial Fibrosis%) demonstrated strong correlations with corresponding pathologists’ scores (n=2431), but had superior associations with post-transplant eGFR (n=2033) and graft loss (n=1560). The combination of KDQS and other factors predicted 1- and 4-year graft loss (discovery: n=520, validation: n=1040). Finally, by matching 398 discarded kidneys due to “biopsy findings” to transplanted population, the matched transplants from discarded KDQS<4 group (110/398, 27.6%) showed similar graft survival rate to unmatched transplanted kidneys (2-, 5-year survival rate: 97%, 86%). KDQS ≥ 7 (37/398, 9.3%) and 1-year survival model score ≥ 0.55 were determined to identify possible discards (PPV=0.92). Conclusion This deep-learning based approach provides automatic and reliable pathological assessment of procurement kidney biopsies, which could facilitate graft loss risk stratification and organ utilization. Translational Statement This deep-learning based approach provides rapid but more objective, sensitive and reliable assessment of deceased-donor kidneys before transplantation, and improves the prognostic value of procurement biopsies, thus could potentially reduce inappropriate discard and stratify patients needing monitoring or preventative measures after transplantation. The pipeline can be integrated into various types of scanners and conveniently generates report after slide scanning. Such report can be used in conjunction with pathologists’ report or independently for centers lacking renal pathologists.
更多查看译文
关键词
kidney,guides organ utilization,deep-learning deep-learning,graft,large-scale
AI 理解论文
溯源树
样例
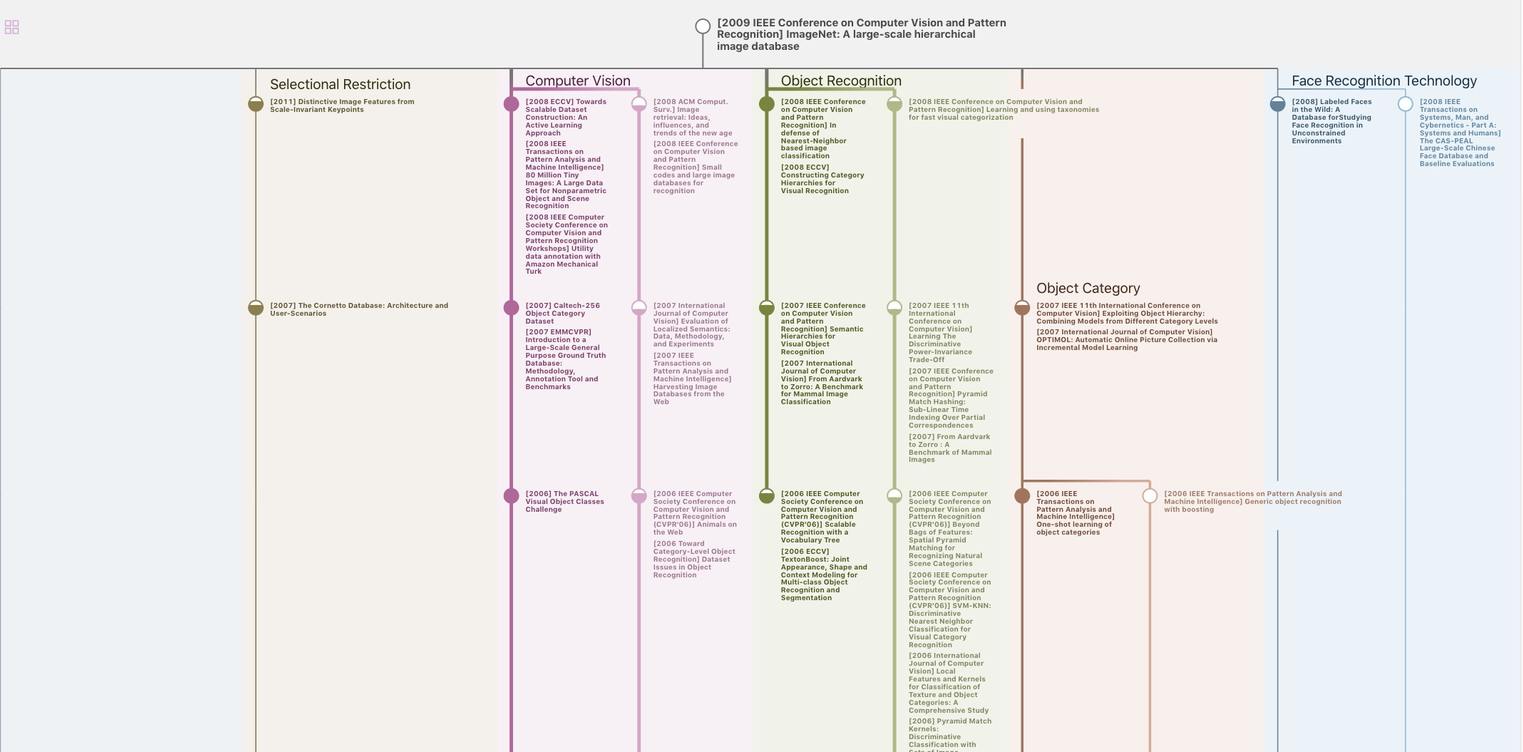
生成溯源树,研究论文发展脉络
Chat Paper
正在生成论文摘要