Abstract 5355: Elraglusib response prediction and mechanistic discovery using iterative machine learning
Cancer Research(2023)
摘要
Abstract Elraglusib is a selective GSK-3 inhibitor that has activity on a broad array of neoplasms as well as their immune microenvironment. However the determinants of tumor response have not been elucidated. Here, we use genomic panels of cancer driver genes to predict patient response to elraglusib therapy as measured by RECIST criteria. Patient response in the Phase I 1801 trial (NCT03678883) was matched to genomic data from patient tumors, with 80 cases used to train Machine Learning models and 26 cases reserved as a test set for model evaluation. When predicting response from mutation status alone our models reached a modest accuracy of 65% in the test set, which we attributed to the sparsity of genomic data. To remedy this, we engineered pathway-based features to provide combinatorial information while ensuring feature stability. To select the optimal combination of features, we designed an iterative design process that first randomized feature combinations and then created refined models from the highest performing pathway features. This resulted in several final models with greater than 88% accuracy. We retained several models of similar accuracy to more comprehensively identify potential biomarkers and combinatorial relationships. These models were then interpreted using SHapley Additive exPlanations (SHAP) where we were able to identify highly predictive features for both the entire pan-cancer cohort and specific histologies. We found the feature constructed from the Reactome pathway “Chromatin Modifying Enzymes” frequently occurred in high performing models with a high-ranking SHAP value. This feature included genes such as SMARCA4, HDAC2, and KDM5A, and was negatively associated with patient response. Another frequently observed high-ranking pathway feature with a negative response association was based on the “Innate Immune System” Reactome pathway. Many of the features highlighted in our models were negatively associated with elraglusib response and thus could be general markers of poor prognosis. To address this, we altered our pipeline to test only positively associated features which substantially reduced our feature pool to only 285 features but still produced models with accuracies of up to 81%. Analysis of key features used by these models identified several positive markers of elraglusib including mutations in the POLE gene, which previously has been linked to DNA-damage response deficiency and anti-tumor immune response. In sum, we forecast elraglusib response for a variety of tumor histologies and simultaneously reveal potential mechanisms of elraglusib sensitivity and biological action. In future work we will investigate the utility of using POLE and other identified biomarker candidates to predict the likelihood of patient response to elraglusib, as well as whether our machine learning models will be an effective tool to guide patient stratification. Citation Format: Joseph McDermott, Taylor Weiskittel, Daniel Billadeau, Benedito Carneiro, Hu Li, Andrew Mazar. Elraglusib response prediction and mechanistic discovery using iterative machine learning. [abstract]. In: Proceedings of the American Association for Cancer Research Annual Meeting 2023; Part 1 (Regular and Invited Abstracts); 2023 Apr 14-19; Orlando, FL. Philadelphia (PA): AACR; Cancer Res 2023;83(7_Suppl):Abstract nr 5355.
更多查看译文
关键词
elraglusib response prediction,mechanistic discovery,machine learning
AI 理解论文
溯源树
样例
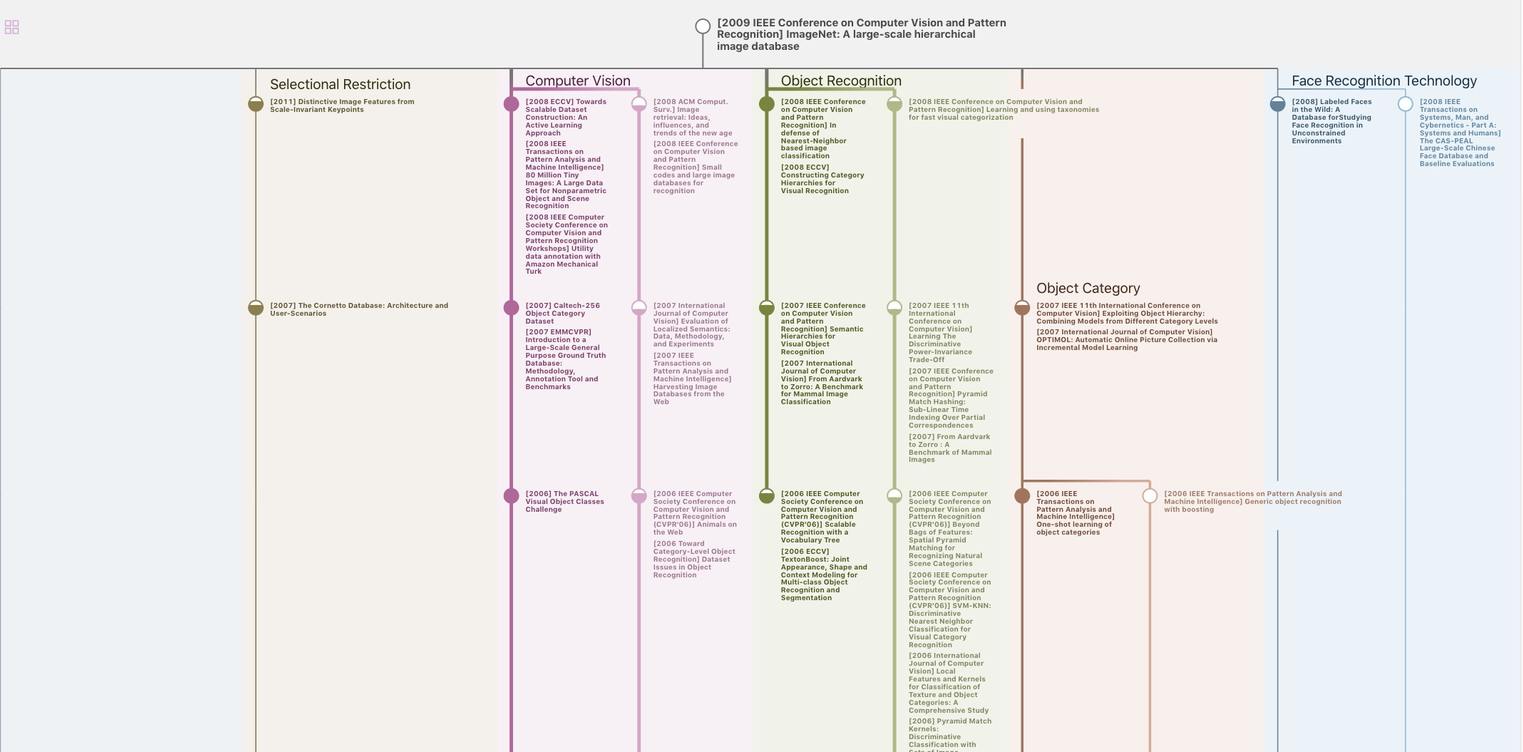
生成溯源树,研究论文发展脉络
Chat Paper
正在生成论文摘要