Appliance Identification via Combinatorial Fusion Analysis-Assisted Bayesian-Optimized Classifier
IEEE TRANSACTIONS ON SMART GRID(2024)
摘要
Non-Intrusive Load Monitoring (NILM) aids in estimating the individual energy consumed by different appliances using aggregate signals measured at point of common connection. This paper explores various unexplored aspects concerning load classification in NILM. Rich feature sets are extracted from load signatures using auto-correlogram, Variational Mode Decomposition (VMD), and Wavelet Packet Tree (WPT) decomposition. Typically, previous works employ a single Feature Selection (FS) technique to earmark the relevant features for improved performance of NILM. However, each FS technique behaves uniquely, and the effects of using different FS techniques or their combinations have yet to be explored for NILM. Hence, Combinatorial Fusion Analysis (CFA), which surpasses the performance of a single FS technique by considering an optimal combination of multiple FS techniques, is used in this paper. The final selected features are fused and provided to a classifier. Rather than manually tuning or performing an exhaustive search for setting the classifier's hyper-parameters, as prevalent in previous works, the present work utilizes Bayesian Optimization (BO) comprising surrogate and acquisition functions. The proposed work's efficacy is validated on publicly available data-sets in a host computer and an embedded device, namely, R-Pi, via comparison with previous works.
更多查看译文
关键词
Feature extraction,Home appliances,Trajectory,Optimization,Tuning,Switches,Signal resolution,Appliance identification,Bayesian optimisation,combinatorial fusion analysis,load signature,hyper-parameter tuning,non-intrusive load monitoring
AI 理解论文
溯源树
样例
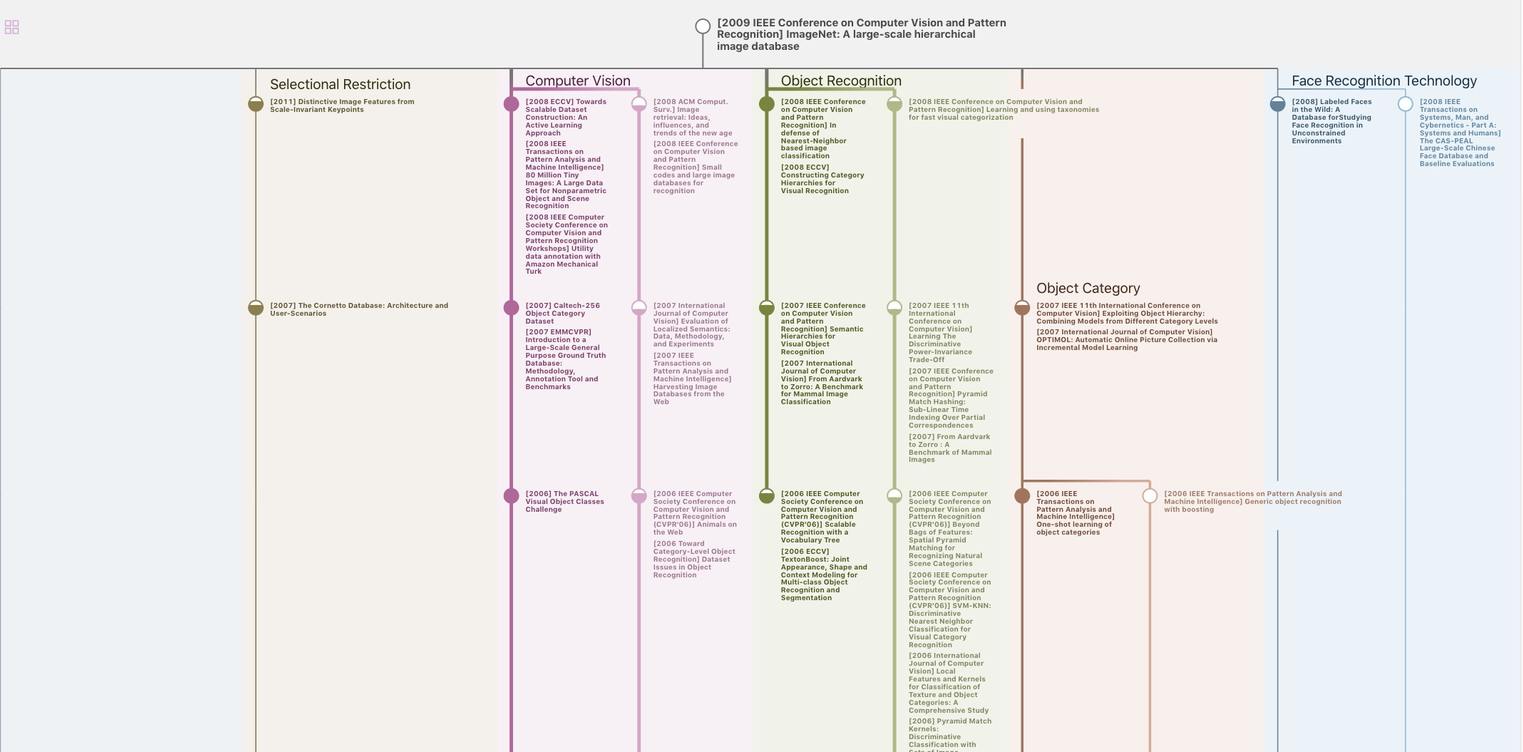
生成溯源树,研究论文发展脉络
Chat Paper
正在生成论文摘要