Machine-learning-based Detection for Quantum Hacking Attacks on Continuous-Variable Quantum-Key-distribution Systems
PHYSICAL REVIEW A(2023)
Abstract
Continuous-variable quantum key distribution (CVQKD) is a mature technology that can theoretically provide an unconditional security guarantee. However, a practical CVQKD system may be vulnerable to various quantum hacking attacks due to imperfect devices and insufficient assumptions. In this paper, we propose a universal defense strategy called a machine-learning-based attack detection scheme (MADS). Leveraging the combined advantages of density-based spatial clustering of applications with noise (DBSCAN) and multiclass support vector machines (MCSVMs), MADS demonstrates remarkable effectiveness in detecting quantum hacking attacks. Specifically, we first establish a set of attack-related features to extract feature vectors. These vectors are then utilized as input data for DBSCAN to identify and remove any noise or outliers. Finally, the trained MCSVMs are employed to classify and predict the processed data. The predicted results can immediately determine whether or not to generate a final secret key. Simulation results show that the proposed MADS can efficiently detect most quantum hacking attacks and revise the overestimated secret key rates caused by a CVQKD system without any defense strategy to obtain a tighter security bound.
MoreTranslated text
AI Read Science
Must-Reading Tree
Example
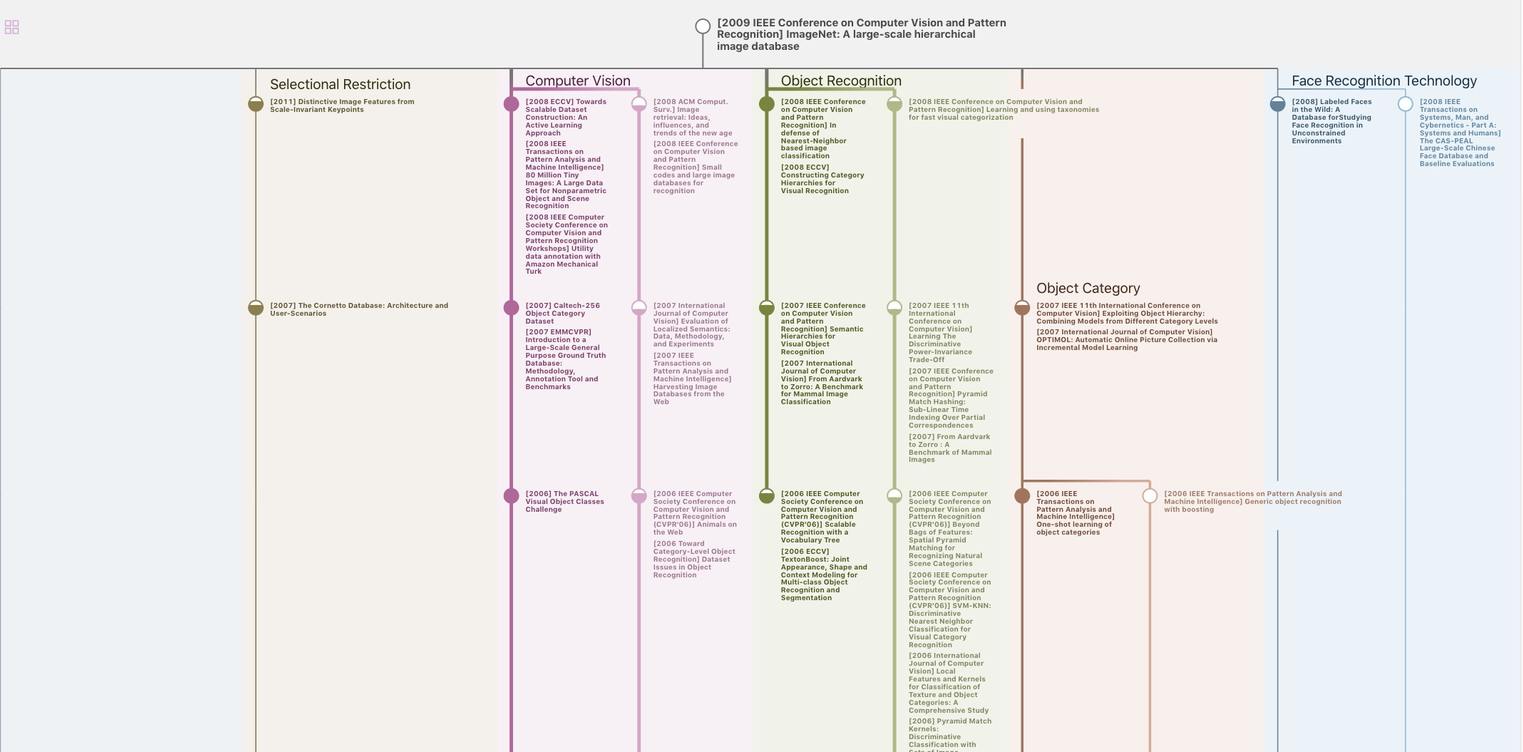
Generate MRT to find the research sequence of this paper
Chat Paper
Summary is being generated by the instructions you defined