Semi-supervised attack detection in industrial control systems with deviation networks and feature selection
The Journal of Supercomputing(2024)
摘要
With the rapid development of Industry 4.0, the importance of cyber security for industrial control systems has become increasingly prominent. The complexity and diversity of industrial control systems result in data with high dimensionality and strong correlation, posing significant challenges in obtaining labeled data. However, current intrusion detection methods often demand large amounts of labeled data for effective training. To address this limitation, this paper proposes a semi-supervised anomaly detection framework, called SFSD, which leverages feature selection and deviation networks to detect anomalies in industrial control systems. Specifically, we introduce a feature selection algorithm (IG-PCA) that utilizes information gain and principal component analysis to reduce the dimensionality of features in industrial control data by eliminating redundant features. Then, we propose a semi-supervised learning method based on an improved deviation network, which utilizes an anomaly scoring network to learn end-to-end anomaly scores for the training data, thus assigning anomaly scores to each training data. Finally, using a limited amount of anomaly-labeled data, we design a specific deviation loss function to optimize the anomaly scoring network, enabling a significant score bias between positive and negative samples. Experimental results demonstrate that the proposed SFSD outperforms existing semi-supervised anomaly detection frameworks by improving the accuracy and detection rate by an average of 1–2
更多查看译文
关键词
Industrial control systems,Intrusion detection,Feature selection,Semi-supervised learning,PCA
AI 理解论文
溯源树
样例
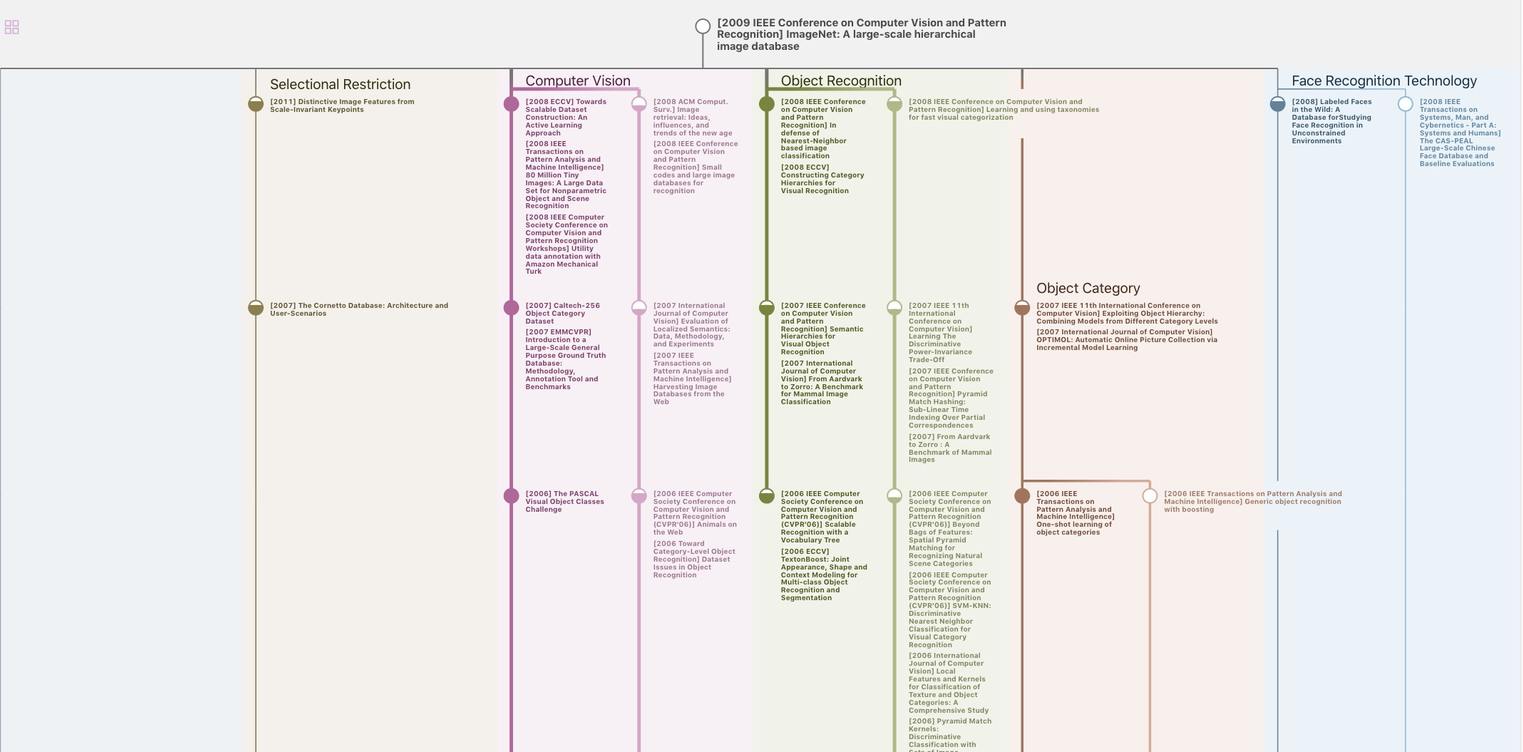
生成溯源树,研究论文发展脉络
Chat Paper
正在生成论文摘要