Calibration-Aware Margin Loss: Pushing the Accuracy-Calibration Consistency Pareto Frontier for Deep Metric Learning
arXiv (Cornell University)(2023)
摘要
The ability to use the same distance threshold across different test classes / distributions is highly desired for a frictionless deployment of commercial image retrieval systems. However, state-of-the-art deep metric learning losses often result in highly varied intra-class and inter-class embedding structures, making threshold calibration a non-trivial process in practice. In this paper, we propose a novel metric named Operating-Point-Incosistency-Score (OPIS) that measures the variance in the operating characteristics across different classes in a target calibration range, and demonstrate that high accuracy of a metric learning embedding model does not guarantee calibration consistency for both seen and unseen classes. We find that, in the high-accuracy regime, there exists a Pareto frontier where accuracy improvement comes at the cost of calibration consistency. To address this, we develop a novel regularization, named Calibration-Aware Margin (CAM) loss, to encourage uniformity in the representation structures across classes during training. Extensive experiments demonstrate CAM's effectiveness in improving calibration-consistency while retaining or even enhancing accuracy, outperforming state-of-the-art deep metric learning methods.
更多查看译文
关键词
metric learning,consistency pareto frontier,calibration-aware,accuracy-calibration
AI 理解论文
溯源树
样例
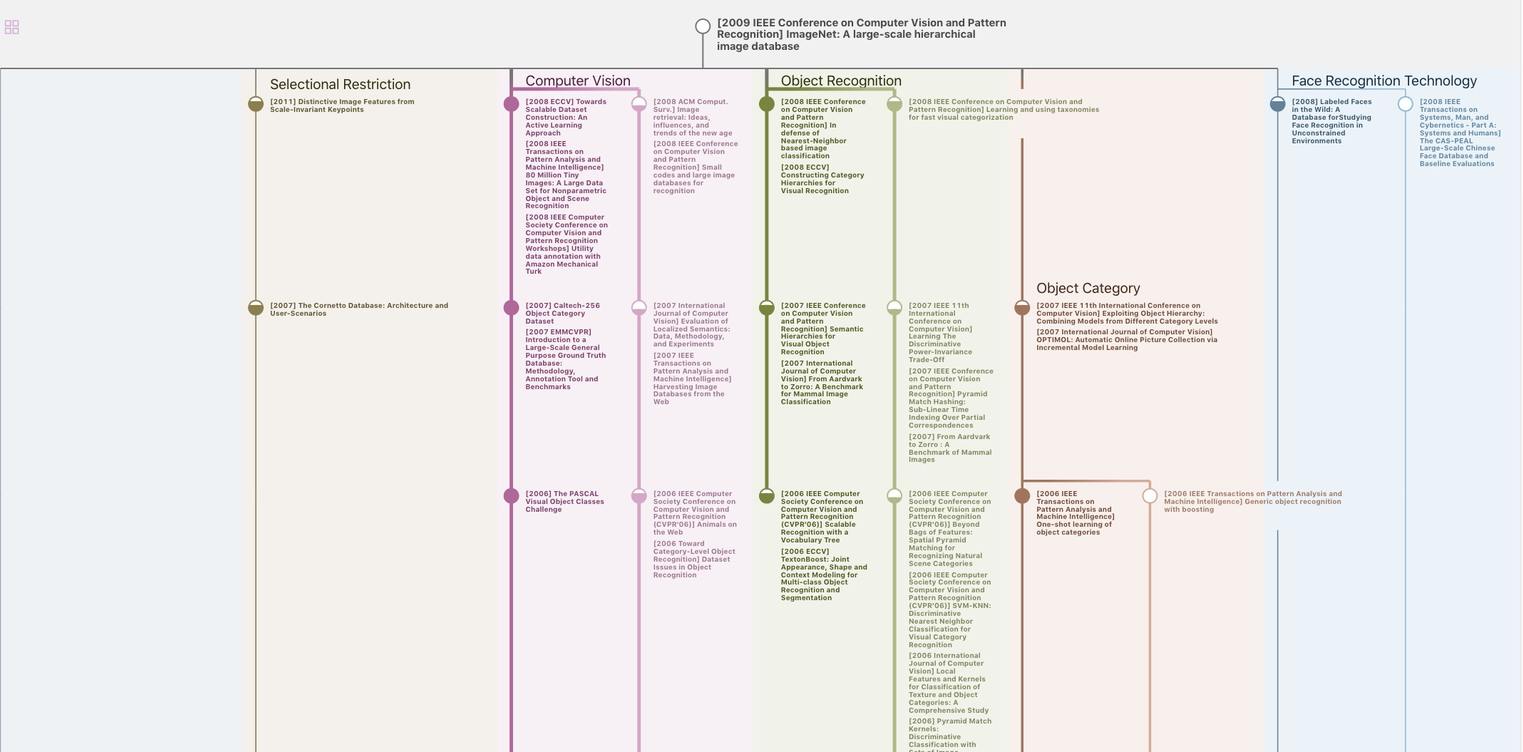
生成溯源树,研究论文发展脉络
Chat Paper
正在生成论文摘要