ImageNet Classification Using WordNet Hierarchy.
IEEE Trans. Artif. Intell.(2024)
摘要
Convolutional neural networks (ConvNets) have be-come increasingly popular for image classification tasks. All contemporary computer vision problems are being dominated by ConvNets. Conventional training methods using cross-entropy loss for training have constantly outperformed the state-of-the-art technique to set a new standard in the ImageNet classification challenge. However, growing accuracy come at the cost of enor-mous number of parameters and computations. Further, classical learning algorithms do not utilise the semantic relationship between the classes present in the dataset. Thus, interpreting the behaviour of the model become difficult even though the results may be desirable. Hence, we demonstrate a classification method by leveraging the WordNet hierarchy on the ImageNet dataset to establish class relationships and label embedding. The model is trained using cross entropy with soft labels based on the semantic similarity between the generated output and the ground truth. Unlike categorical cross entropy, it does not treat every predicted label as equally erroneous. The method generates meaningful neighbouring classes in the feature space of the true label.
更多查看译文
关键词
WordNet,ImageNet,Convolutional neural net-works,soft labels
AI 理解论文
溯源树
样例
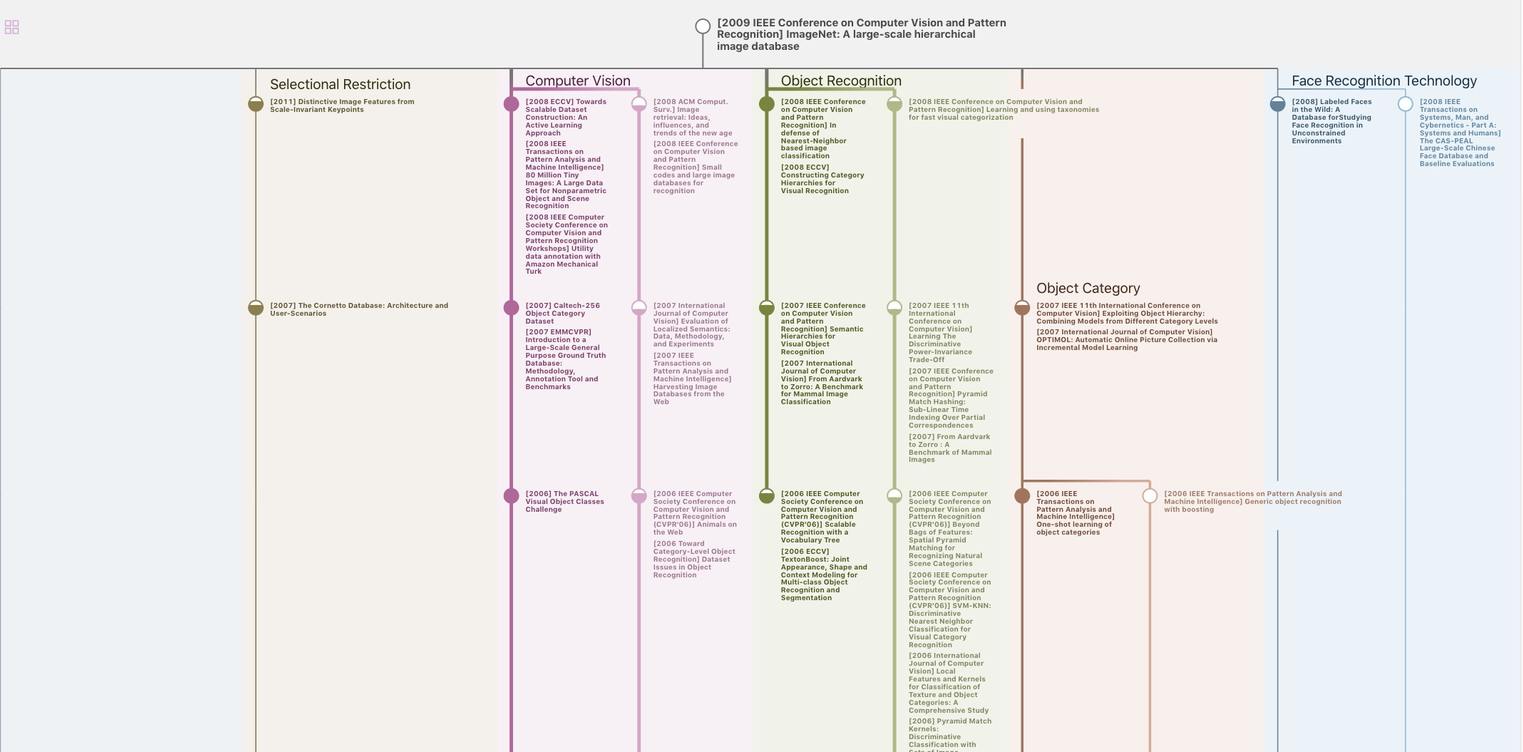
生成溯源树,研究论文发展脉络
Chat Paper
正在生成论文摘要