Individual patterns of functional connectivity in neonates as revealed by surfaced-based Bayesian modeling
bioRxiv (Cold Spring Harbor Laboratory)(2023)
摘要
Abstract Resting-state functional connectivity is a widely used approach to study the functional brain network organization during early brain development. However, the estimation of functional connectivity networks in individual infants has been rather elusive due to the unique challenges involved with functional magnetic resonance imaging (fMRI) data from young populations. Here, we use fMRI data from the developing Human Connectome Project (dHCP) database to characterize individual variability in a large cohort of term-born infants (N = 289) using a novel data-driven Bayesian framework. To enhance alignment across individuals, the analysis was conducted exclusively on the cortical surface, employing surface-based registration guided by age-matched neonatal atlases. Using 10 minutes of resting-state fMRI data, we successfully estimated subject-level maps for fourteen brain networks/subnetworks along with individual functional parcellation maps that revealed differences between subjects. We also found a significant relationship between age and mean connectivity strength in nearly all brain regions, including previously unreported findings in higher-order networks. These results illustrate the potential advantages of surface-based methods and statistical approaches in uncovering individual variability within very young populations.
更多查看译文
关键词
functional connectivity,bayesian modeling,neonates,individual patterns,surfaced-based
AI 理解论文
溯源树
样例
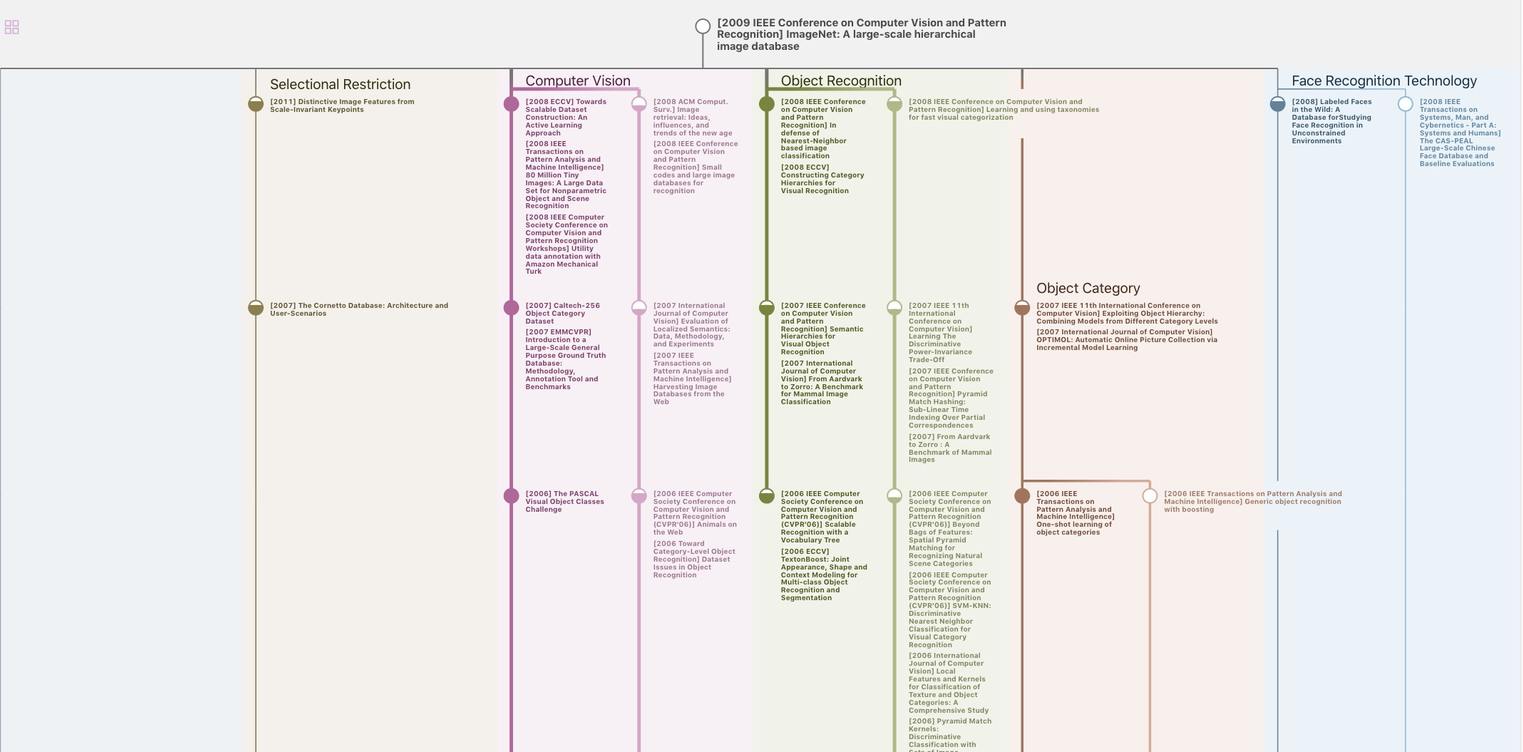
生成溯源树,研究论文发展脉络
Chat Paper
正在生成论文摘要