P995: indeed: a deep learning model for diagnosis and subtypes differentiation of myeloproliferative neoplasm based on intelligence neural network
HemaSphere(2023)
摘要
Topic: 15. Myeloproliferative neoplasms - Biology & Translational Research Background: The classical BCR-ABL1-negative myeloproliferative neoplasms (MPN) include polycythemia vera (PV), essential thrombocythaemia (ET) and primary myelofibrosis (PMF). Artificial intelligence (AI) model has attracted substantial attention for its satisfy performance in hematological malignancies. The application in MPN diagnosis is still rarely reported. Aims: To prospectively develop an intelligence neural network based deep learning model for myeloproliferative neoplasm diagnostics and subtypes differentiation, briefly named INDEED model, which incorporates pathologic whole slide image (WSI). Methods: Training set was composed of totally 819 MPN patients and 232 non-MPN controls from five centers in China, details of composition shown in Figure 1B. Hematoxylin and Eosin (HE) staining WSI of bone marrow biopsy of all the participants were collected and scanned using Pannoramic SCAN 150 (3DHISTECH Ltd., Budapest, Hungary). INDEED modelling process included WSI automatic pre-processing and selection, self-supervised contrastive learning feature extraction, and multiple instance learning and classifying (Figure 1A), which was tuned in the internal testing set. Further, the INDEED model was independently validated in the set additionally collected from the four hospitals (Validation Set 1), and externally validated in the other two hospitals (Validation Set 2 & 3). Area under curve (AUC) and 95% confidence interval of receiver operating characteristic (ROC) analysis was used to evaluate model accuracy, and the optimal cut-off points for subtypes were estimated by Youden index in internal testing set (Figure 1C). Results: INDEED model for MPN diagnostics and subtype differentiation reached AUC values over 0.95 and 0.89 in the training set and internal testing set, respectively. In the validation phase, for MPN diagnostics versus non-MPN controls, INDEED achieved AUC of 0.911(0.890-0.932) in Validation Set 1, and well replicated in the Validation Sets 2 & 3, obtaining AUCs of 0.938(0.922-0.953) and 0.804(0.759-0.842), respectively. For PV subtype differentiation, INDEED achieved AUC of 0.847 (0.821-0.874) in validation Set 1, and further replicated in Validation Sets 2 and 3, respectively, which got AUCs of 0.928(0.910-0.941) and 0.899(0.879-0.915). For ET differentiation, INDEED obtained AUCs of 0.873(0.854-0.890), 0.833(0.805-0.860), and 0.832(0.804-0.864) in Validation Sets 1 to 3, respectively. Similar, for pre-PMF, AUCs of the model were 0.894(0.863-0.922), 0.782(0.732-0.827), 0.847(0.817-0.870), respectively. For overt-PMF, the AUC values were estimated as 0.981(0.972-0.990), 1.000(1.000-1.000), and 1.000(1.000-1.000) in Validation Sets 1 to 3, respectively. Overall, in the combined validation set of the three validation sets, the average AUC of INDEED achieved 0.884 for MPN, and 0.891 for PV, 0.846 for ET,0.841 for pre-PMP, and 0.994 for overt-PMF (Figure 1D). In the combined validation set, 167 of 345 samples (48.4%) had two predicted subtypes; the redefined accuracy reached 77.2%. In addition, there were a small fraction of samples (9.3%) were predicted into three subtypes, which had overall 90.6% accuracy. Overall, the INDEED model reached 83.8% accuracy. Distribution of non-unique predictions may provide key objectives for future model-assisted subtype differentiation. Summary/Conclusion: The proposed INDEED model exhibits well performance in MPN diagnostics and subtypes differentiation. The model without cell-level annotation is convenient to train and fast to apply, and provide a new insight for the AI-assisted MPN diagnosis.Keywords: Myeloproliferative disorder
更多查看译文
关键词
myeloproliferative neoplasm based,deep learning model,deep learning,neural network
AI 理解论文
溯源树
样例
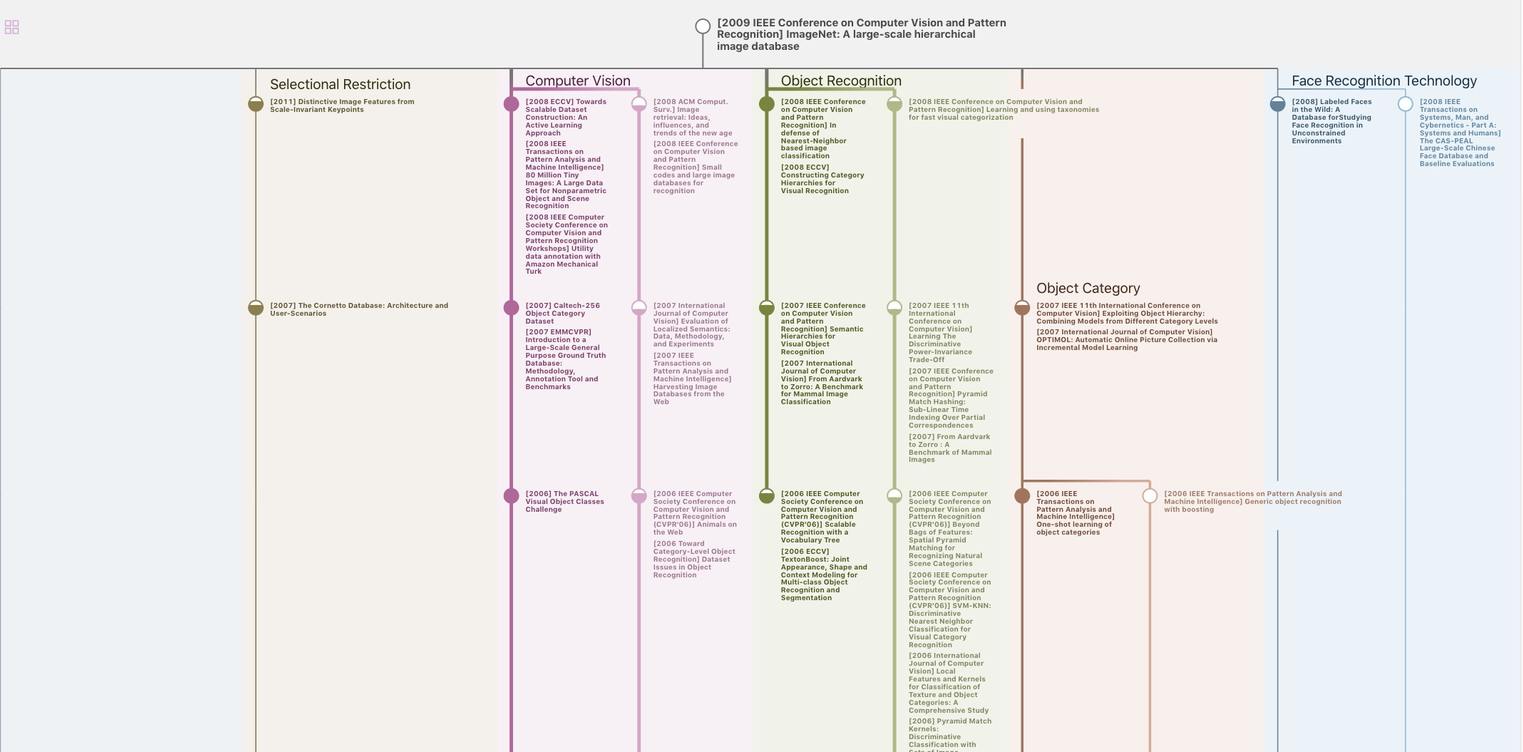
生成溯源树,研究论文发展脉络
Chat Paper
正在生成论文摘要