UAV Trajectory Optimization for Large-Scale and Low-Power Data Collection: An Attention-Reinforced Learning Scheme.
IEEE Trans. Wirel. Commun.(2024)
摘要
Unmanned Aerial Vehicles (UAVs) exhibit great advantages in data collection from ground sensors in vast tracts of fields. Due to their limited power supply, most works assume that the UAV simply traverses each sensor’s fixed transmission range to collect data, thereby shortening the flight path. However, they neglect the quality of collected data, which may deteriorate dramatically as the transmission distance increases. In this paper, by leveraging the physical-layer protocol – LoRa, we propose a Packet Reception Ratio (PRR)-based probabilistic coverage model to evaluate the quality of data transmission, which directly determines the data acquisition efficiency. On this basis, to minimize the energy consumption of UAV and sensors while ensuring high-quality data acquisition, we formulate the UAV trajectory planning as a joint Energy Consumption and data Acquisition Efficiency (ECAE) optimization problem. To tackle the ECAE problem, we propose a Deep Reinforcement Learning (DRL)-based two-stage scheme. First, an attention-based encoder-decoder model is trained to generate an initial trajectory. Then an intuitive optimization algorithm is devised to further explore the optimal trajectory. Evaluation results show that our scheme can reduce the total energy cost of UAV and sensors by 27.1% as compared to the best baseline’s policy while maintaining a promising PRR.
更多查看译文
关键词
Unmanned aerial vehicle,trajectory optimization,data collection,attention model,deep reinforcement learning,LoRa
AI 理解论文
溯源树
样例
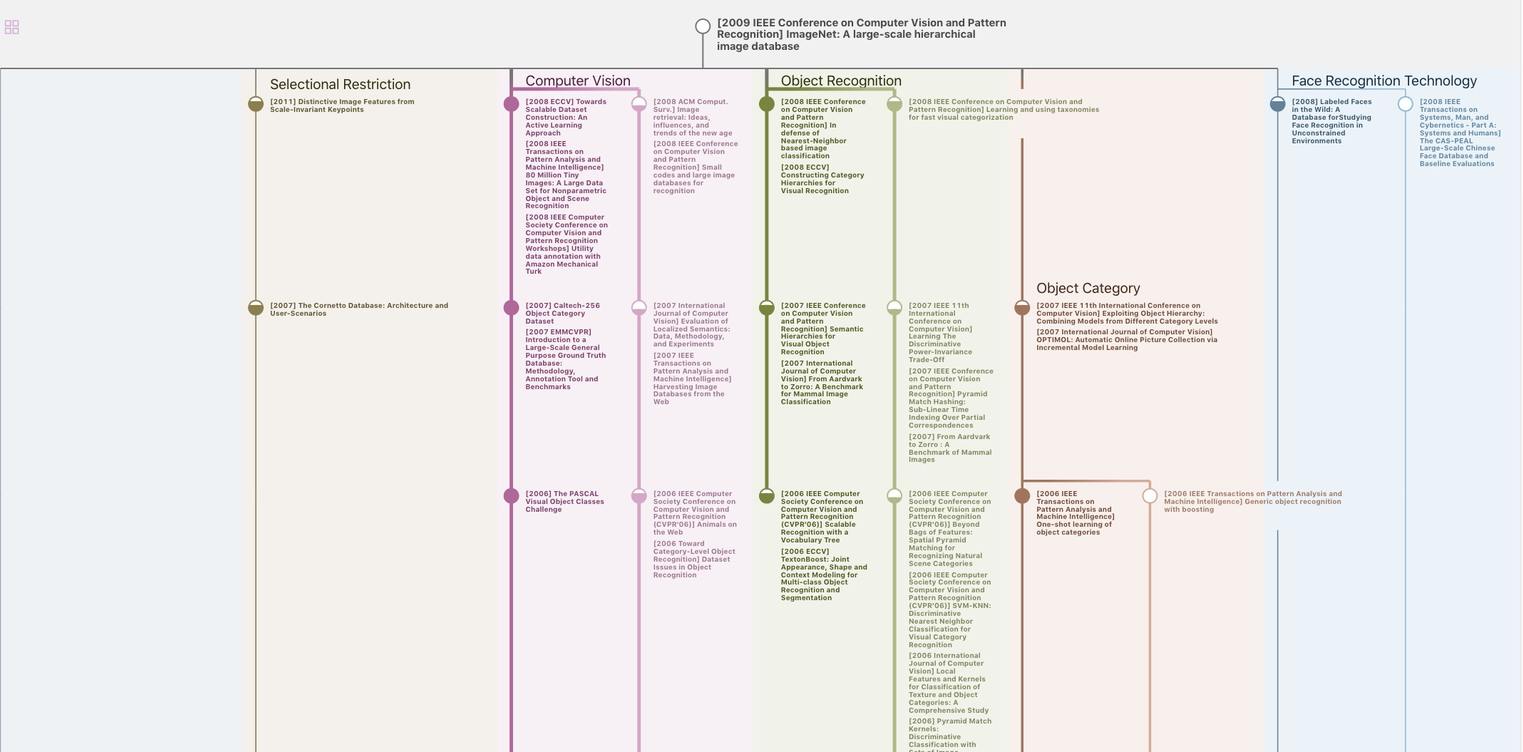
生成溯源树,研究论文发展脉络
Chat Paper
正在生成论文摘要