Centralized data sources and QSAR methods for the prediction of idiosyncratic adverse drug reaction
Elsevier eBooks(2023)
摘要
With the increase of new regimens and medications, adverse drug reaction has become an important factor that can increase the risk of drug use. Better understanding of its molecular mechanisms and clinical relevance can be beneficial for preventing the occurrence of adverse events. However, several obstacles still block the way of using models to facilitate the prevention of ADRs. For instance, some ADRs happen rarely because of the lack of treatment experience and evidence. It is hard to apply comprehensive investigation due to the lack of centralized data sources. Moreover, with numerous models, generating appropriate descriptors based on structure–activity information and choosing a compatible model are delicate procedures. The potential risk could not be effectively assessed unless a valid model was utilized. Hence, it is hard to carry out an early detection and intervention of ADRs. Thus, in this chapter, aiming to provide assistance in wiping out some obstacles, a constriction of centralized data sources of ADRs was demonstrated, and a strategy of selecting proper quantitative structure–activity relationship models and machine learning models was proposed. Two projects with two different ADRs, namely drug-induced rhabdomyolysis and drug-induced teratogenesis during pregnancy, with their corresponding drugs were described in this chapter. The selected models from the projects can well predict the most-concerned drug based on chemical structures. Furthermore, the strategy can be complementary for early detection of ADRs and risk assessment in drug development.
更多查看译文
关键词
idiosyncratic adverse drug reaction,qsar methods,centralized data sources
AI 理解论文
溯源树
样例
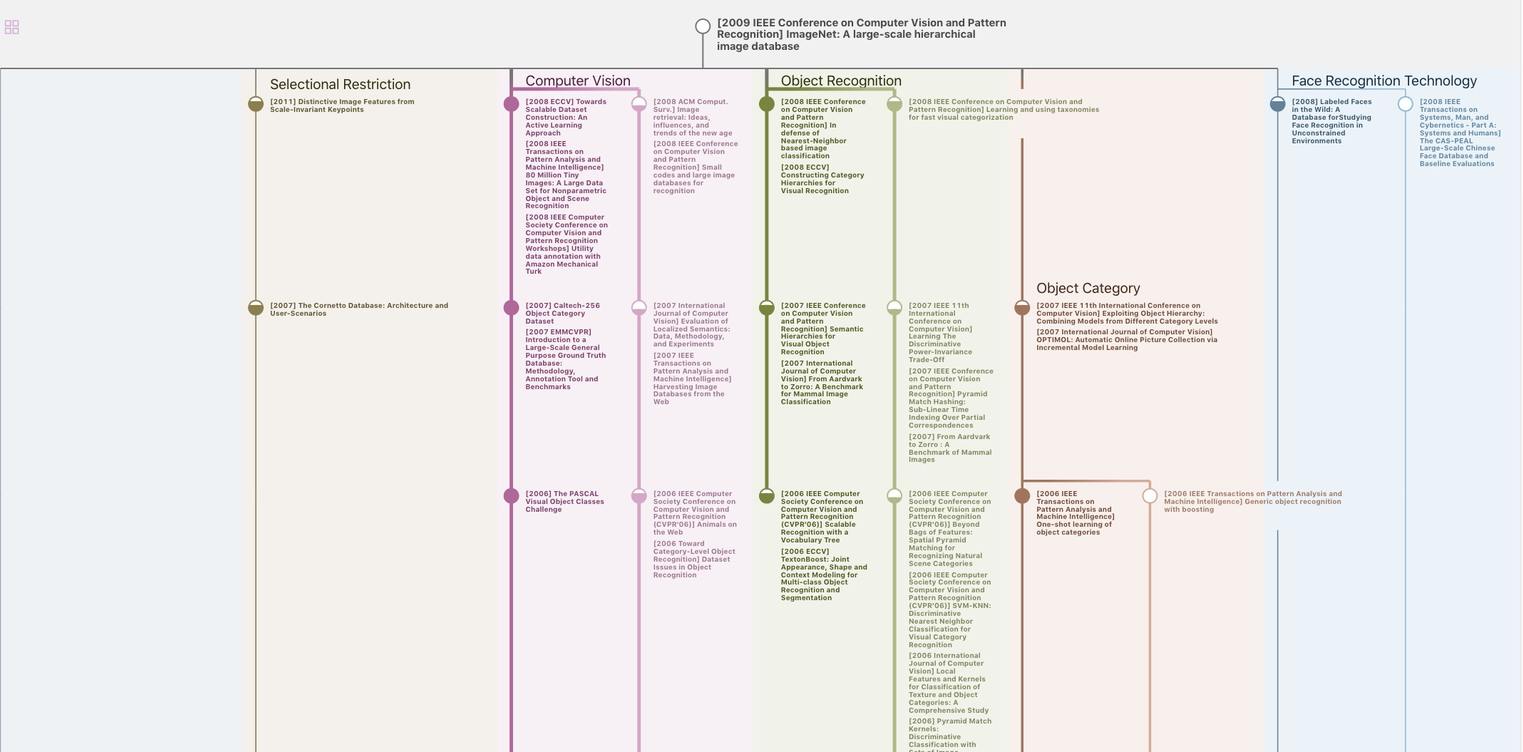
生成溯源树,研究论文发展脉络
Chat Paper
正在生成论文摘要