Application of QSAR models based on machine learning methods in chemical risk assessment and drug discovery
Elsevier eBooks(2023)
摘要
Quantitative structure–activity relationship (QSAR) modeling has found broad utility in predicting the toxicological or pharmacological activities of chemicals based on their structural information. In this chapter, we will describe a complete QSAR model development and application process using combinations of different machine learning methods and molecular fingerprints, which includes data preprocessing, followed by strategies to evaluate and optimize model performance, such as feature selection and data rebalancing, and finally virtual screening and experimental validation of predictions. Examples of successful applications of QSAR models in chemical risk assessment (i.e., the prediction of in vivo or in vitro toxicity of environmental compounds) and drug discovery (i.e., the prediction of cytochrome P450 3A7/3A4 inhibitors and substrates, anti-SARS-CoV-2 compounds, acetylcholinesterase (AChE), and butyrylcholinesterase (BChE) inhibitors, and opioid receptor agonists and antagonists) will be discussed. In conclusion, robust QSAR models based on machine learning methods can be applied to efficiently predict the bioactivity of compounds.
更多查看译文
关键词
qsar models,chemical risk assessment,machine learning methods,machine learning
AI 理解论文
溯源树
样例
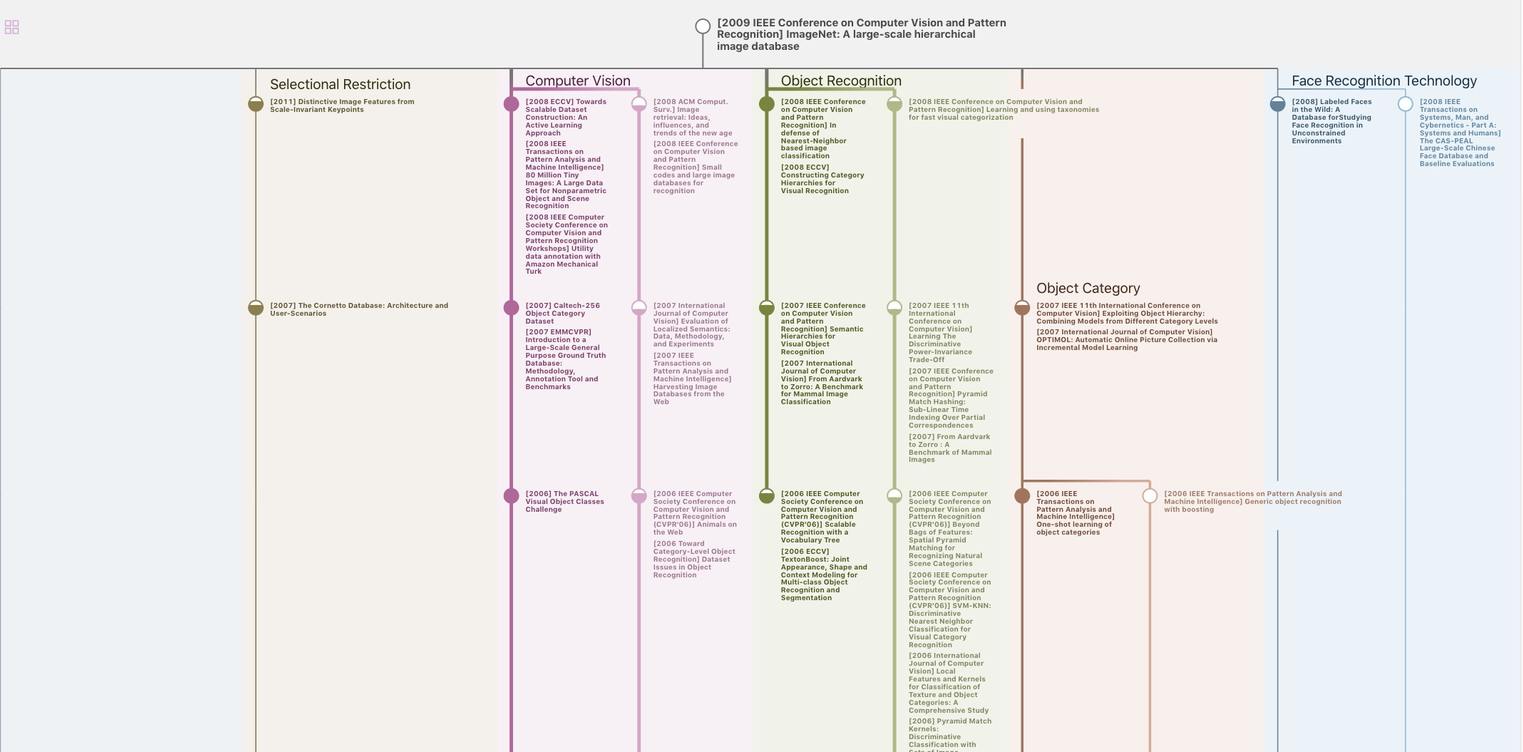
生成溯源树,研究论文发展脉络
Chat Paper
正在生成论文摘要