Less is More: Towards Efficient Few-shot 3D Semantic Segmentation via Training-free Networks
arXiv (Cornell University)(2023)
摘要
To reduce the reliance on large-scale datasets, recent works in 3D segmentation resort to few-shot learning. Current 3D few-shot semantic segmentation methods first pre-train the models on `seen' classes, and then evaluate their generalization performance on `unseen' classes. However, the prior pre-training stage not only introduces excessive time overhead, but also incurs a significant domain gap on `unseen' classes. To tackle these issues, we propose an efficient Training-free Few-shot 3D Segmentation netwrok, TFS3D, and a further training-based variant, TFS3D-T. Without any learnable parameters, TFS3D extracts dense representations by trigonometric positional encodings, and achieves comparable performance to previous training-based methods. Due to the elimination of pre-training, TFS3D can alleviate the domain gap issue and save a substantial amount of time. Building upon TFS3D, TFS3D-T only requires to train a lightweight query-support transferring attention (QUEST), which enhances the interaction between the few-shot query and support data. Experiments demonstrate TFS3D-T improves previous state-of-the-art methods by +6.93% and +17.96% mIoU respectively on S3DIS and ScanNet, while reducing the training time by -90%, indicating superior effectiveness and efficiency.
更多查看译文
关键词
segmentation,3d,networks,few-shot,training-free
AI 理解论文
溯源树
样例
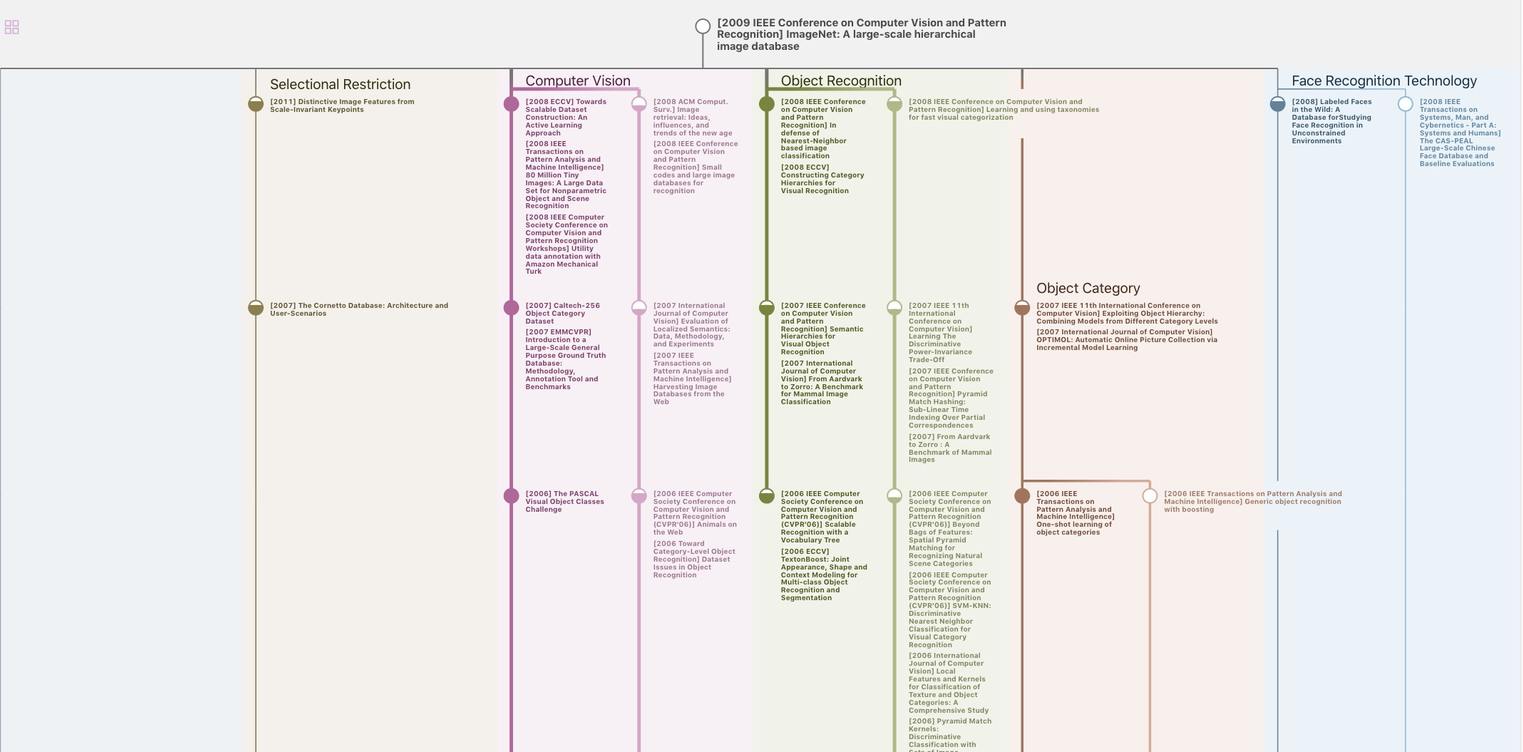
生成溯源树,研究论文发展脉络
Chat Paper
正在生成论文摘要