Probing feature spaces of object categories with a drawing task
Journal of Vision(2023)
摘要
Humans categorize both familiar and novel objects quickly and consistently. Many theories posit that we achieve this by forming category boundaries around the perceived object in a high-dimensional psychological feature space. As of now, these spaces are mostly theoretical constructs without appropriate empirical methods available to probe them. Here, we combined a machine-learning based shape synthesis method with an intuitive drawing task to make detailed measurements of one-shot generalization, and the shifting shape of category boundaries as additional exemplars are provided. We synthesised novel abstract 2D forms with naturalistic shape statistics using a GAN trained on a dataset of >25K animal silhouettes. We then presented 20 participants with one or multiple such objects, and asked them to draw their own new objects belonging to the same category as the exemplar(s) on a tablet computer, resulting in >1600 drawings. This unconstrained task allowed us to assess what observers considered to be legal shape variations within each category. Although the drawings were substantially different from the original exemplar (i.e., not mere copies), they revealed highly systematic and constrained types of variation. To model the resulting perceptual spaces, we used ‘ShapeComp’—an image-computable model of human shape similarity based on >100 shape descriptors. We found that drawings cluster considerably closer around the originating exemplar than around non-related reference shapes (p < .01). Furthermore, this cluster shrank when observers were simultaneously presented with additional samples of the same category (p < .01). Importantly, generalization was anisotropic within shape space, indicating that different features were preserved and varied depending on the exemplar. Together, these findings reveal how people form categorical boundaries in feature space, based on identifying which features are most relevant for that category.
更多查看译文
关键词
object categories,feature spaces
AI 理解论文
溯源树
样例
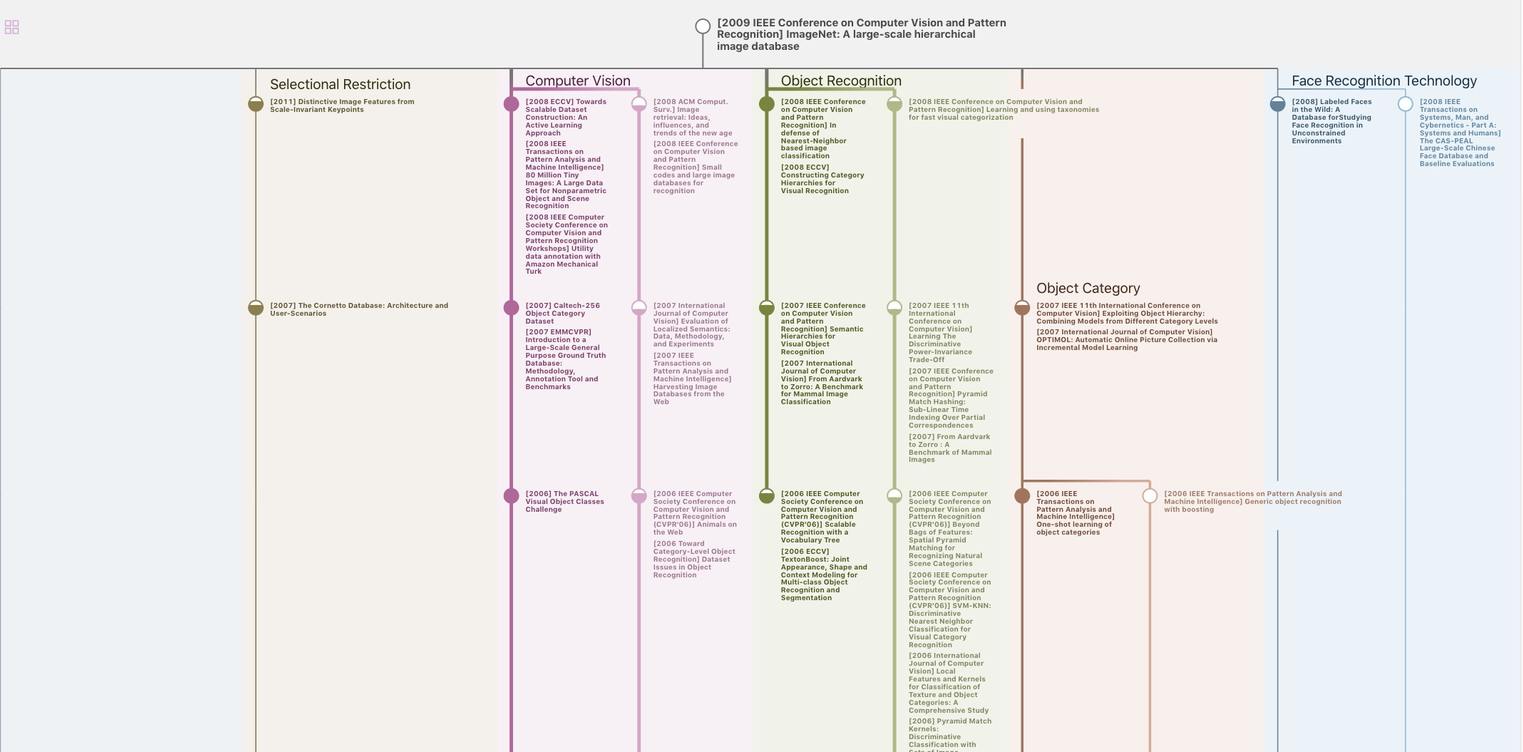
生成溯源树,研究论文发展脉络
Chat Paper
正在生成论文摘要