Clothes-Changing Person Re-identification Via Universal Framework with Association and Forgetting Learning
IEEE transactions on multimedia(2024)
摘要
Clothes-changing person re-identification (Re-ID) aims at learning identity-relevant feature representations among clothing-changed persons. Currently, the state-of-the-art methods accomplish this task by using additional assistance (e.g., silhouettes, sketches, clothes labels, etc.) to explore identity-relevant information. However, humans do not require redundant assistance information to retrieve clothing-changed persons. It is commonly known that humans can recall targets they have seen before with a simple reminder. Inspired by human perception, we propose an association and forgetting learning (AFL) framework for clothes-changing person re-identification. Specifically, on the one hand, during the association learning process, the AFL framework constructs association factors for each identity to simulate the reminders found in human perception. Then, the original instances and the explored hardest positive instances are cross-correlated by the association factors to learn identity-relevant features. On the other hand, the model is forced to forget the identity-irrelevant features by the proposed forgetting learning module, which improves the intra-class compactness. Finally, we further propose a clustering relationship exploration (CRE) module to optimize the cluster distribution of clothes-changing instances, which enables AFL to also be effectively applied in unsupervised settings, improving the universal applicability of the model. Extensive experiment results obtained on clothes-changing person Re-ID datasets under supervised and unsupervised settings demonstrate the superiority of the proposed method over the existing state-of-the-art methods.
更多查看译文
关键词
Clothing,Task analysis,Image color analysis,Germanium,Cameras,Robustness,Interference,Person re-identification (Re-ID),clothes-changing,association learning,forgetting learning
AI 理解论文
溯源树
样例
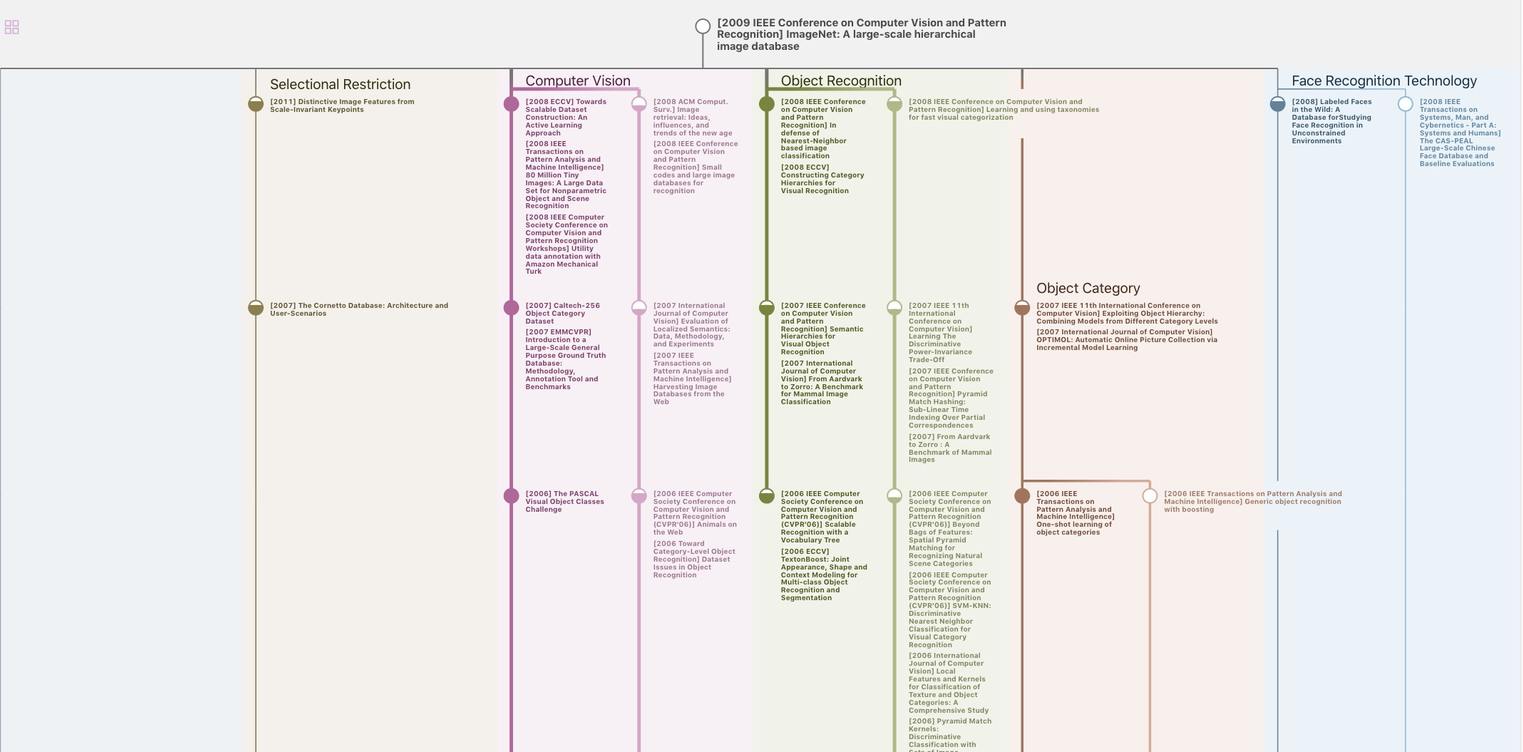
生成溯源树,研究论文发展脉络
Chat Paper
正在生成论文摘要