Differentiable Modeling and Optimization of Battery Electrolyte Mixtures Using Geometric Deep Learning
arXiv (Cornell University)(2023)
摘要
Electrolytes play a critical role in designing next-generation battery systems, by allowing efficient ion transfer, preventing charge transfer, and stabilizing electrode-electrolyte interfaces. In this work, we develop a differentiable geometric deep learning (GDL) model for chemical mixtures, DiffMix, which is applied in guiding robotic experimentation and optimization towards fast-charging battery electrolytes. In particular, we extend mixture thermodynamic and transport laws by creating GDL-learnable physical coefficients. We evaluate our model with mixture thermodynamics and ion transport properties, where we show improved prediction accuracy and model robustness of DiffMix than its purely data-driven variants. Furthermore, with a robotic experimentation setup, Clio, we improve ionic conductivity of electrolytes by over 18.8% within 10 experimental steps, via differentiable optimization built on DiffMix gradients. By combining GDL, mixture physics laws, and robotic experimentation, DiffMix expands the predictive modeling methods for chemical mixtures and enables efficient optimization in large chemical spaces.
更多查看译文
关键词
geometric deep learning,deep learning,property optimization,gradient-based
AI 理解论文
溯源树
样例
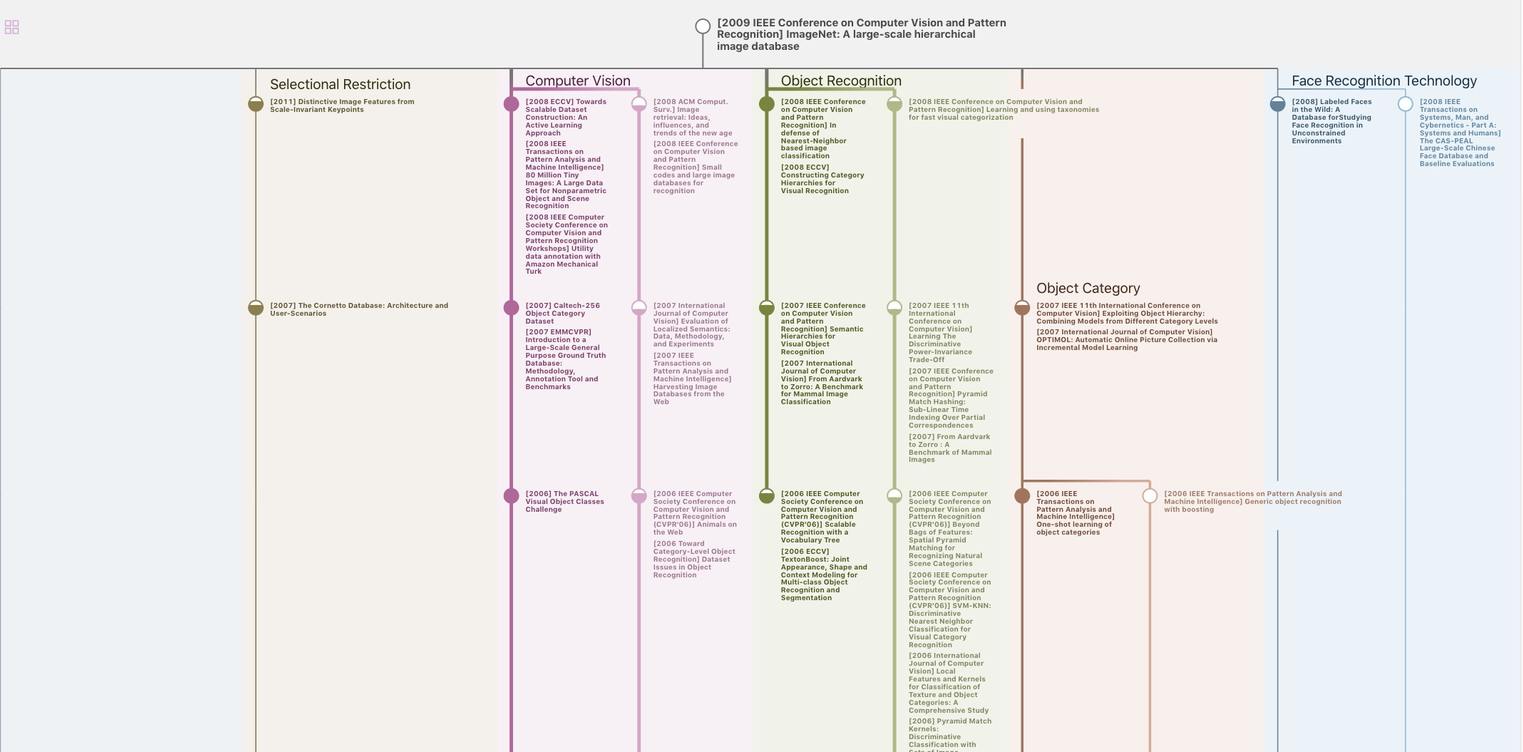
生成溯源树,研究论文发展脉络
Chat Paper
正在生成论文摘要