Using Unsupervised Natural Language Processing to Automatically Identify Colonic Dysplasia in Pathology Reports From Patients With Chronic Colitis
The American Journal of Gastroenterology(2023)
摘要
Introduction: Identifying dysplasia in the setting of chronic colitis requires manual review of unstructured pathology reports, which may vary in terminology or description of dysplasia. Natural Language Processing (NLP) technologies are used to extract data from free text format in the electronic medical record (EMR). We aimed to develop and validate an NLP based algorithm to identify presence of dysplasia in the setting of chronic colitis from pathology reports within an integrated EMR system. Methods: We developed an unsupervised, rule-based regular expression NLP algorithm to identify “dysplasia” with “chronic colitis” and their corresponding location alongside a list of negation terms within pathology reports derived from the EMR (EPIC) at a large quaternary care medical center in Houston, Texas. The algorithm’s performance was evaluated in comparison to authors NSL, SB, and MK's interpretation of the contents of the same pathology reports. A portion of the pathology reports were reviewed by multiple authors to ensure adequate intra-observer agreement. The algorithm's performance was calculated as accuracy, sensitivity, precision and F- measure. Results: We queried 9508 pathology reports and identified 480 patients with chronic colitis, of whom 48 had dysplasia on colonic biopsies. The NLP algorithm identified dysplasia with 97.5% accuracy, 89.5% sensitivity, 86% precision and an F-measure of 93.7% when compared with manual review. The NLP algorithm was able to identify the location of dysplasia with 93% accuracy, 87.9% precision and an F-measure of 78%. Conclusion: Unsupervised NLP approach identified the presence and location of dysplasia in the setting of chronic colitis with high degree of accuracy from pathology reports. We expect our algorithm’s performance to improve with the utilization of training sets. Application of this algorithm has the potential to improve patient identification to enhance research and clinical care across large EMRs (Table 1). Table 1. - Performance characteristics of the NLP algorithm for detection of colonic dysplasia and dysplasia location in pathology reports Measure Accuracy Sensitivity Specificity Precision F-Measure Dysplasia 97.5 89.5 98.4 86.0 93.7 Location 93.1 64.5 98.3 87.9 77.9
更多查看译文
关键词
chronic colitis,natural language processing,unsupervised natural language processing,colonic dysplasia
AI 理解论文
溯源树
样例
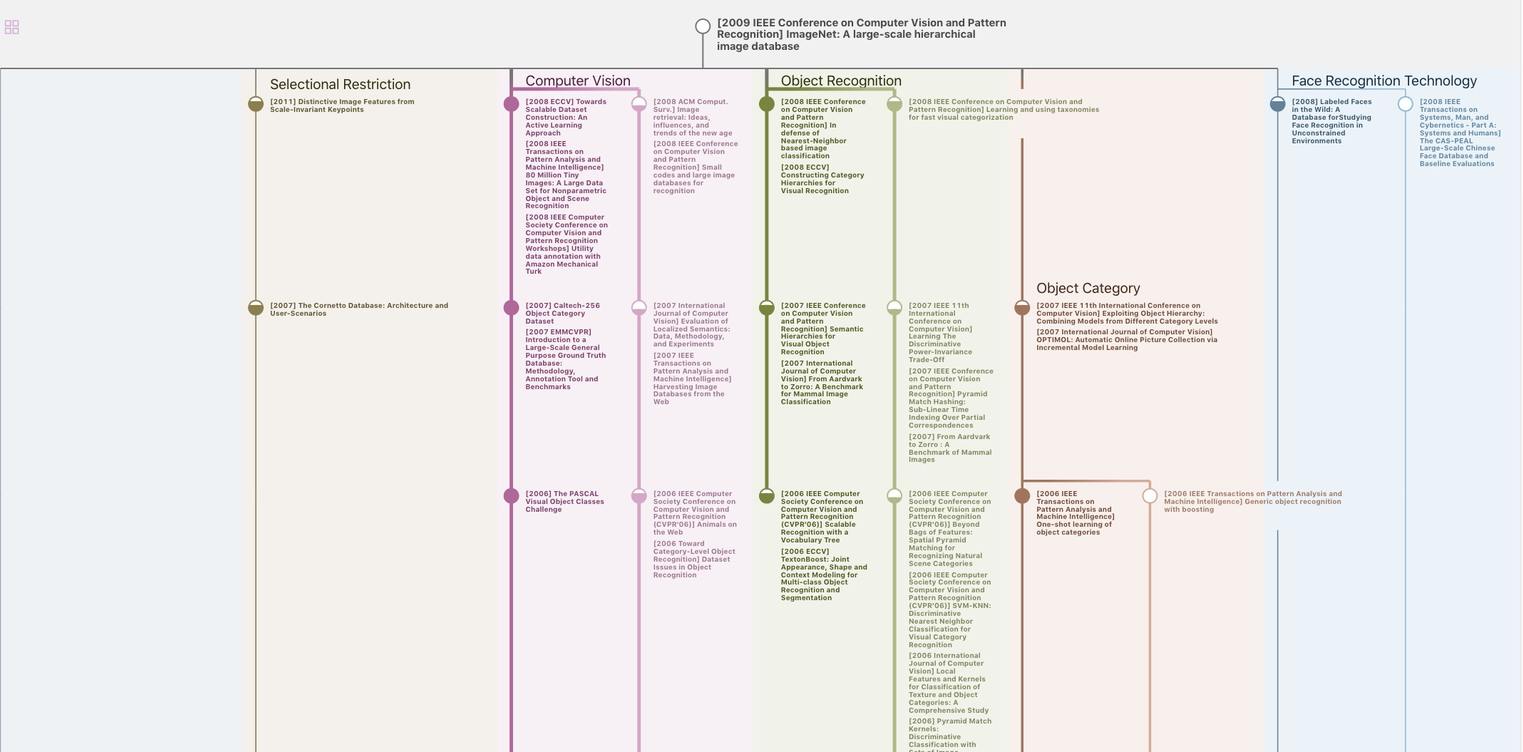
生成溯源树,研究论文发展脉络
Chat Paper
正在生成论文摘要