Accelerating antibiotic discovery by leveraging machine learning models: application to identify novel inorganic complexes
Research Square (Research Square)(2023)
摘要
Abstract The expanded prevalence of resistant bacteria and the inherent challenges of complicated infections highlight the urgent need to develop alternative antibiotic options. Through conventional screening approaches, the discovery of new antibiotics has proven to be challenging. Anti-infective drugs, including antibacterials, antivirals, antifungals, and antiparasitics, have become less effective due to the spread of drug resistance. In this work, we helped define the design of next-generation antibiotic analogs based on metal complexes. For this purpose, we used artificial intelligence (AI) methods, demonstrating superior ability to tackle resistance in Gram-positive and Gram-negative bacteria, including multidrug-resistant strains. The existing AI approaches' bottleneck relies on the current antibiotics' structural similarities. Herein, we developed a machine learning approach that predicts the minimum inhibitory concentration (MIC) of Re-complexes towards two S. aureus strains (ATCC 43300 - MRSA and ATCC 25923 - MSSA). A Multi-layer Perceptron (MLP) was tailored with the structural features of the Re-complexes to develop the prediction model. Although our approach is demonstrated with a specific example of rhenium carbonyl complexes, the predictive model can be readily adjusted to other candidate metal complexes. The work shows the application of the developed approach in the de novo design of a metal-based antibiotic with targeted activity against a challenging pathogen.
更多查看译文
关键词
antibiotic discovery,complexes,machine learning,machine learning models
AI 理解论文
溯源树
样例
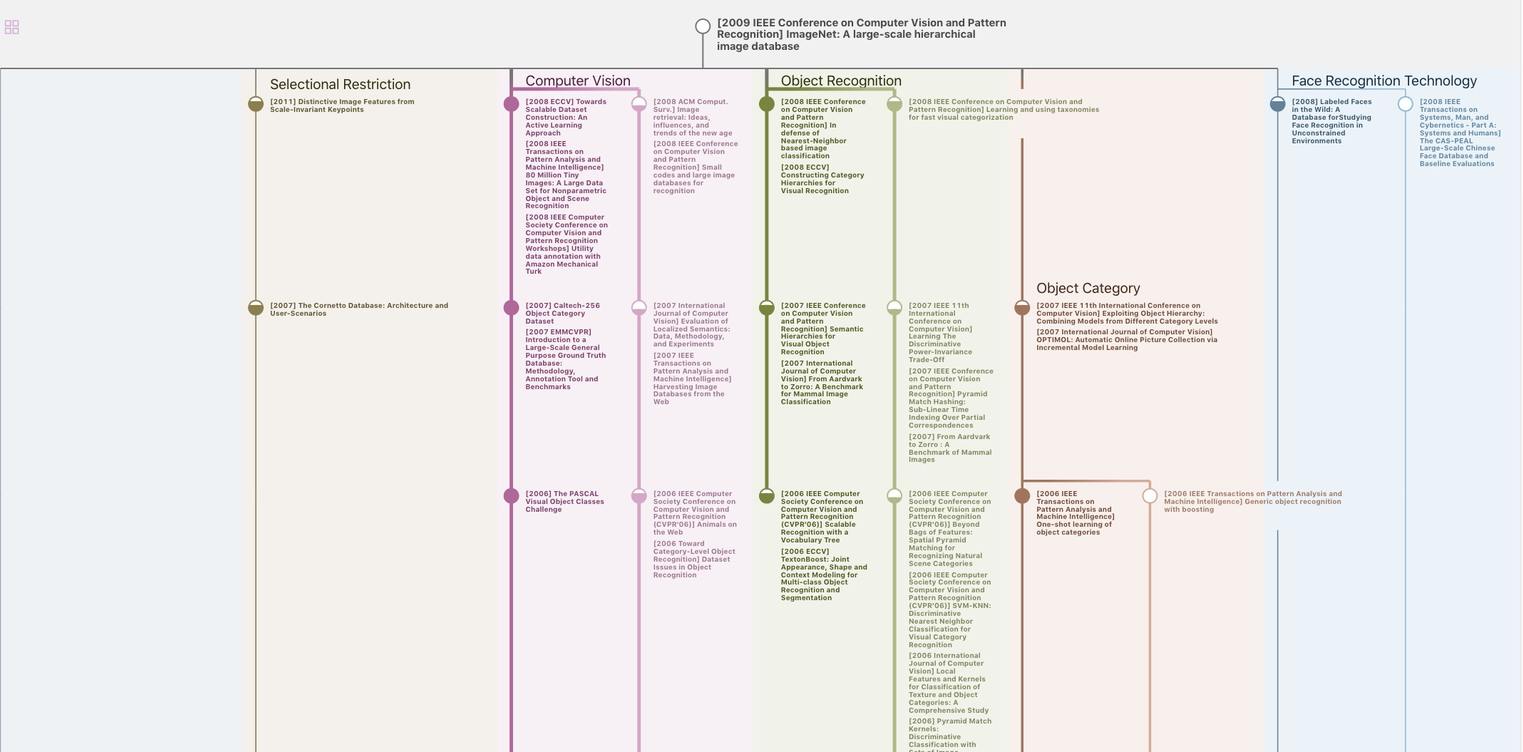
生成溯源树,研究论文发展脉络
Chat Paper
正在生成论文摘要