���械学習と結晶方位解析を用いたオーステナイト鋼の塑性ひずみ量の推定
Nihon Kikai Gakkai Kanto Shibu Sokai Koenkai koen ronbunshu(2023)
摘要
In this study, we development a novel technique to estimate plastic strain by image discrimination using ResNet, one of the convolutional neural networks. The input images to ResNet were prepared from the crystal misorientation data obtained by the electron backscatter diffraction method corresponding to the degree of plastic deformation. Since the logarithmic values of the crystal misorientation in each region followed a normal distribution, machine learning was performed using images normalized by the mean and standard deviation of the logarithmic values of the crystal misorientation. The results revealed that the standardized images indicated the local strain distribution due to plastic deformation and became smoother according to the increasing plastic strain. The standardized images also clearly showed the difference in damage distribution between unloaded and loaded conditions. The developed machine learning system identified five different levels of plastic strain applied to austenitic steel with an accuracy of 68%. It is expected that the plastic strain can be predicted from the average value of the estimated plastic strain calculated from the machine learning results if a sufficient evaluation area is available.
更多查看译文
AI 理解论文
溯源树
样例
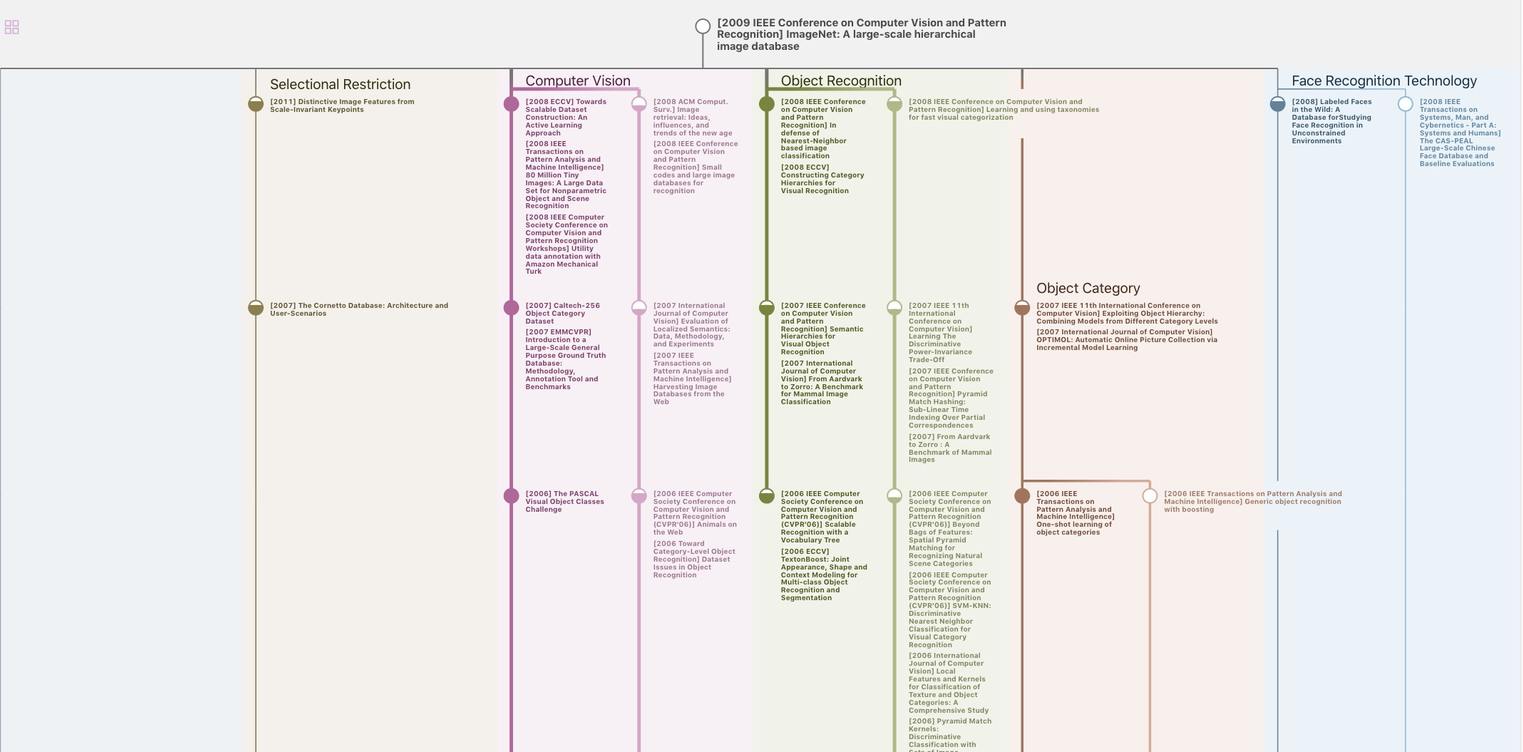
生成溯源树,研究论文发展脉络
Chat Paper
正在生成论文摘要