Personalized identification of Autism-related bacteria in the gut microbiome using eXplainable Artificial Intelligence
Research Square (Research Square)(2023)
摘要
Abstract Background Autism spectrum disorder (ASD) constitutes a pervasive developmental condition impacting social interaction and communication proficiency. Emerging evidence underscores a plausible association between ASD and alterations within the gut microbiome—an intricate assembly of microorganisms inhabiting the gastrointestinal tract. While machine learning (ML) techniques have emerged as a valuable tool for unraveling the intricate interactions between the gut microbiome and host physiology, their application faces limitations in assessing the individual contributions of microbial species for each subject. Addressing this constraint, explainable artificial intelligence (XAI) emerges as a solution. This paper delves into the potential of the Shapley Method Additive Explanations (SHAP) algorithm for personalized identification of microbiome biomarkers in the context of ASD. Results The study demonstrates the efficacy of the SHAP algorithm in overcoming conventional ML limitations. SHAP enables a personalized assessment of microbiome contributions, facilitating the identification of specific bacteria associated with ASD. Moreover, leveraging local explanation embeddings and an unsupervised clustering method successfully clusters ASD subjects into subgroups. Notably, a cluster with lower ASD probability is identified, uncovering false negatives in ASD classification. The recognition of false negatives holds clinical significance, prompting an exploration of contributing factors and insights for refining ASD classification accuracy. Conclusions In conclusion, XAI provides personalized insights into ASD-associated microbiome biomarkers. Its ability to address ML limitations enhances understanding of individualized microbial environment in ASD. The identification of ASD subgroups through clustering analysis emphasizes disorder heterogeneity. Additionally, recognizing false negatives within ASD classification introduces complexity to patient care considerations. These findings imply potential for tailored interventions based on individual microbiome profiles, advancing precision in ASD management and classification.
更多查看译文
关键词
gut microbiome,explainable artificial intelligence,bacteria,artificial intelligence,autism-related
AI 理解论文
溯源树
样例
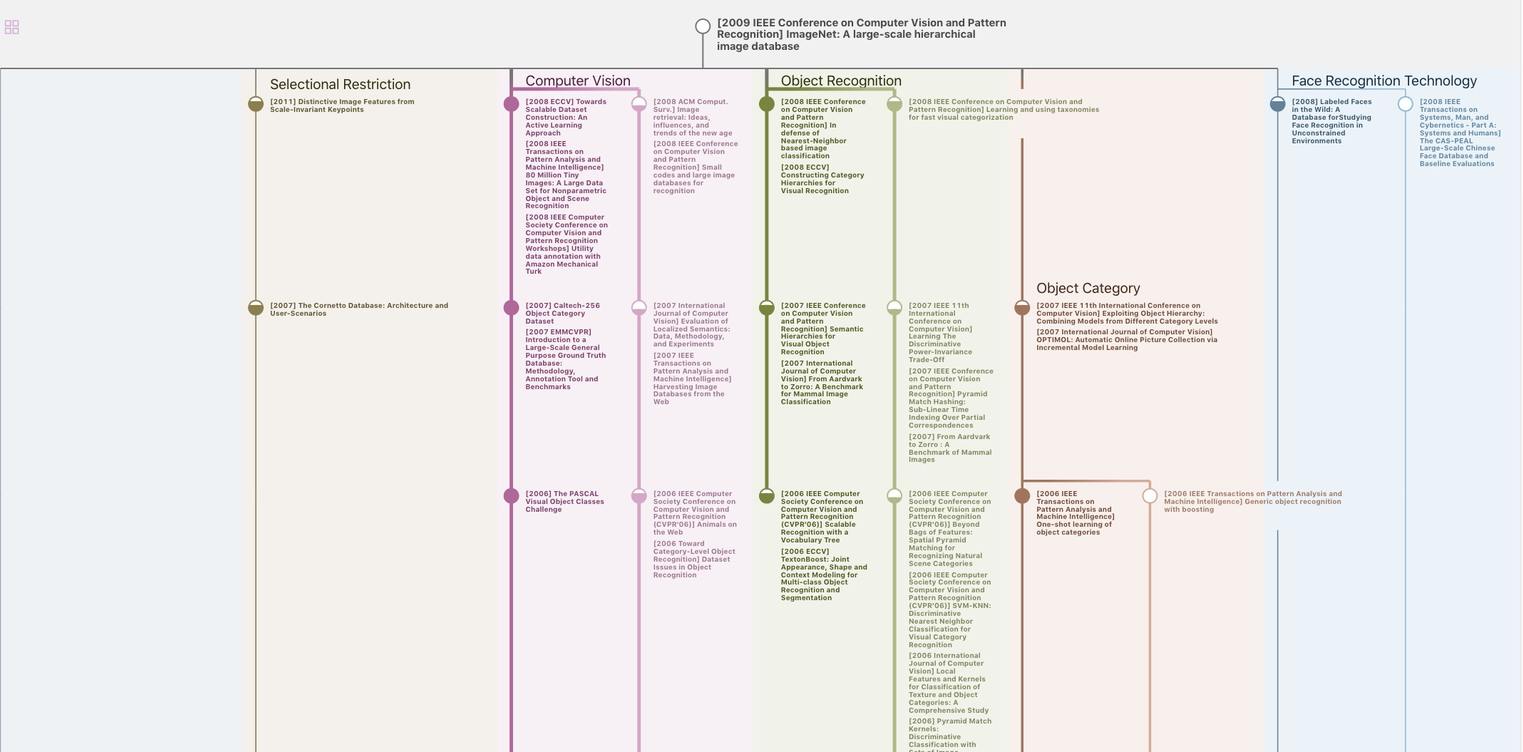
生成溯源树,研究论文发展脉络
Chat Paper
正在生成论文摘要