Towards Hierarchical Clustered Federated Learning with Model Stability on Mobile Devices
IEEE Transactions on Mobile Computing(2023)
摘要
Clustered federated learning (CFL) has proved to be an effective way to alleviate the non-IID (not independently and identically distributed) data challenge, which severely restricts the wider application of federated learning. However, existing approaches either lack adaptability,
i.e.
, they require an additional number of clusters as a guide when clustering, or lack effectiveness in terms of communication. In this paper, we explore the differences in the ability of different layers in a model to represent non-IID data, and propose a hierarchical CFL approach, named
HiCFL
, which considers both adaptivity and communication efficiency. The improvement of communication efficiency is due to our proposed novel concept of model stability, which characterizes the variation of model weights during training. Based on model stability,
HiCFL
can find the proper time to bi-partition the clusters of mobile devices in a hierarchical manner more quickly. We conduct extensive experiments based on popular datasets with various non-IID data settings. The results show that
HiCFL
achieves excellent performance effectiveness and efficiency. Compared to state-of-the-art approaches,
HiCFL
can improve the model accuracy by
$2.0\% \sim 9.0\%$
, while reducing the communication overheads by
$27.3\% \sim 80.6\%$
.
更多查看译文
关键词
Federated learning,clustered federated learning,communication efficiency,hierarchical clustering,model stability
AI 理解论文
溯源树
样例
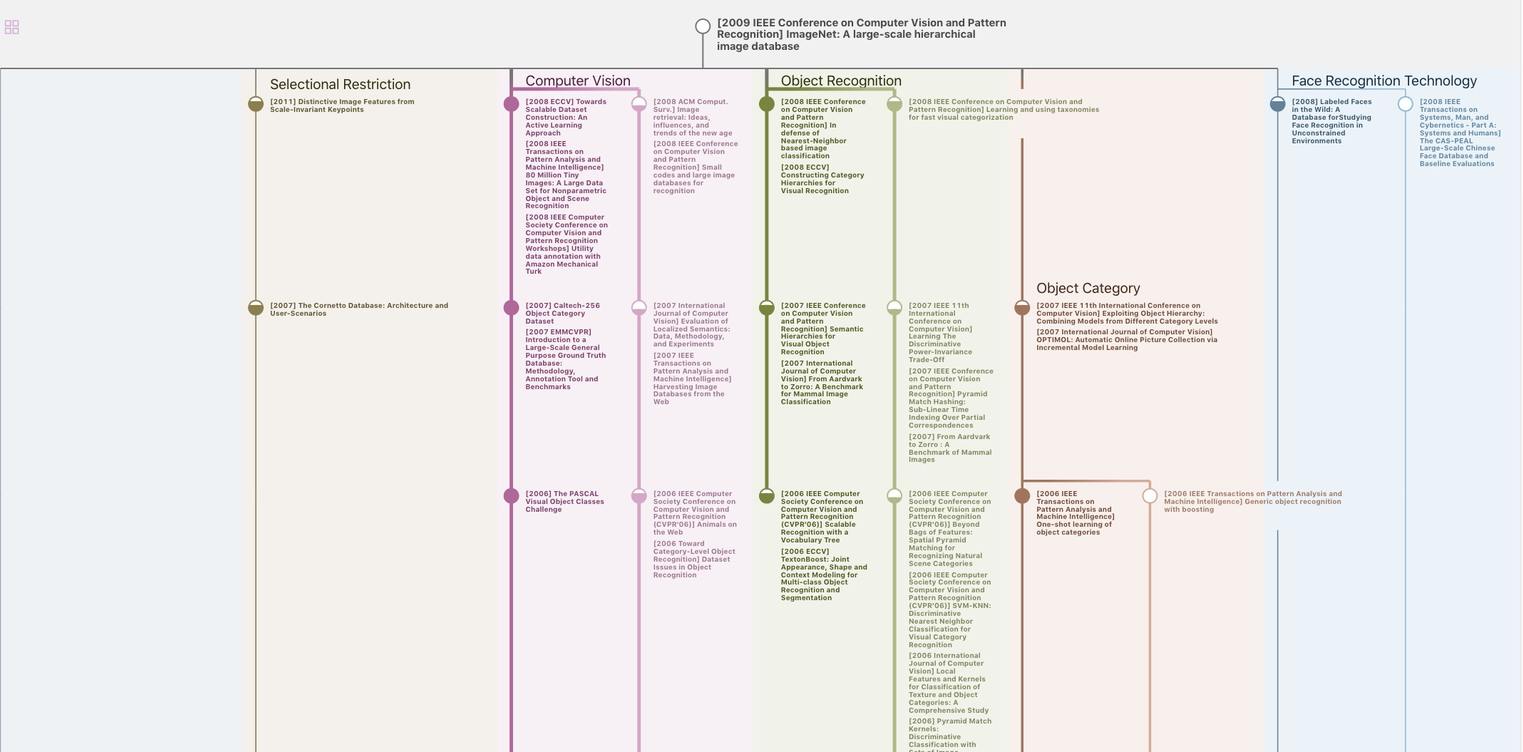
生成溯源树,研究论文发展脉络
Chat Paper
正在生成论文摘要