CT-based AI machine learning model for predicting efficacy of chemoradiotherapy for esophageal squamous cell carcinoma
Annals of Oncology(2023)
摘要
Chemoradiotherapy (CRT) is one of the most effective treatments for esophageal squamous cell carcinoma (ESCC) because CRT is potentially curable and less invasive than surgery. However, there is no useful biomarker for predicting the response and prognosis of CRT for ESCC. Therefore, we developed non-invasive and low-cost CT-based machine learning model for efficacy prediction of CRT in ESCC by radiomics analysis. We enrolled 44 patients with ESCC (stage I - IV) and analyzed the CT images of cancer. The 476 radiomics features were extracted from the CT data of each cancer in training cohort (n=27). We extracted 12 radiomics features significantly associated with the CRT responder from CT images. We then constructed an algorithm to predict response to CRT based on the 12 features and response, employing 5 kinds of machine learning methods (random forest (RF), ridge regression (RR), naïve bayes (NB), support vector machine (SVM), and artificial neural network models (ANN)), Of these, the RF model showed the highest AUC value of 0.99 (p<0.001, accuracy of 77.8%, sensitivity of 100%, specificity of 71.4%). The other classifiers also showed high diagnostic rates with AUC values ranging 0.95 to 0.98. We then evaluated ROC curves in validation cohort (n=17). The optimal cutoff value was set as 0.18 by the Youden index. Similarly, the RF model showed the highest AUC value of 0.92 (p<0.001, accuracy of 82.4%, sensitivity of 83.3%, specificity of 81.8%). NB and RR models also showed high AUC values of more than 0.8. Using the data of all patients, we evaluated whether the RF model is able to predict prognosis as well. The Kaplan-Meier analysis revealed that our model could successfully predict long-term response after CRT such as PFS (30.2 vs 5.9 months, p<0.0001) and OS (100.4 vs 13.0 months, p<0.0001). Thus, we successfully established a non-invasiveness and low cost AI-based radiomics model that can predict CRT response and prognosis of ESCC with very high accuracy.
更多查看译文
关键词
chemoradiotherapy,squamous cell carcinoma,ai machine learning model,machine learning,ct-based
AI 理解论文
溯源树
样例
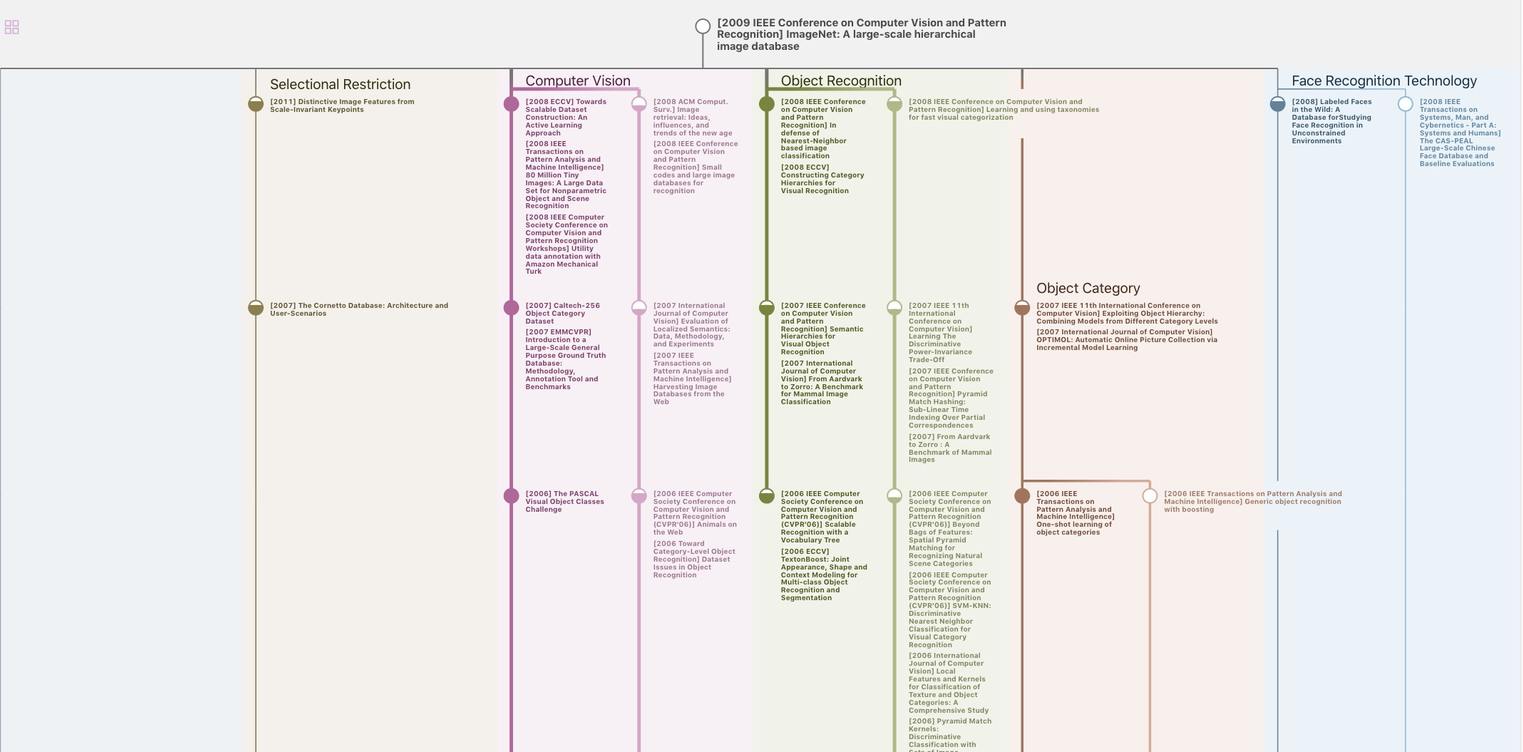
生成溯源树,研究论文发展脉络
Chat Paper
正在生成论文摘要