TRustworthy Uncertainty Propagation for Sequential Time-Series Analysis in RNNs
IEEE TRANSACTIONS ON KNOWLEDGE AND DATA ENGINEERING(2024)
摘要
The massive time-series production through the Internet of Things and digital healthcare requires novel data modeling and prediction. Recurrent neural networks (RNNs) are extensively used for analyzing time-series data. However, these models are unable to assess prediction uncertainty, which is particularly critical in heterogeneous and noisy environments. Bayesian inference allows reasoning about predictive uncertainty by estimating the posterior distribution of the parameters. The challenge remains in propagating the high-dimensional distribution through the sequential, non-linear layers of RNNs, resulting in mode collapse leading to erroneous uncertainty estimation and exacerbating the gradient explosion problem. This paper proposes a TRustworthy Uncertainty propagation for Sequential Time-series analysis (TRUST) in RNNs by introducing a Gaussian prior over network parameters and estimating the first two moments of the Gaussian variational distribution using the evidence lower bound. We propagate the variational moments through the sequential, non-linear layers of RNNs using the first-order Taylor approximation. The propagated covariance of the predictive distribution captures uncertainty in the output decision. The extensive experiments using ECG5000 and PeMS-SF classification and weather and power consumption prediction tasks demonstrate 1) significant robustness of TRUST-RNNs against noise and adversarial attacks and 2) self-assessment through the uncertainty that increases significantly with increasing noise.
更多查看译文
关键词
Gated recurrent units and long short-term memory networks,recurrent neural networks,uncertainty propagation,variational inference
AI 理解论文
溯源树
样例
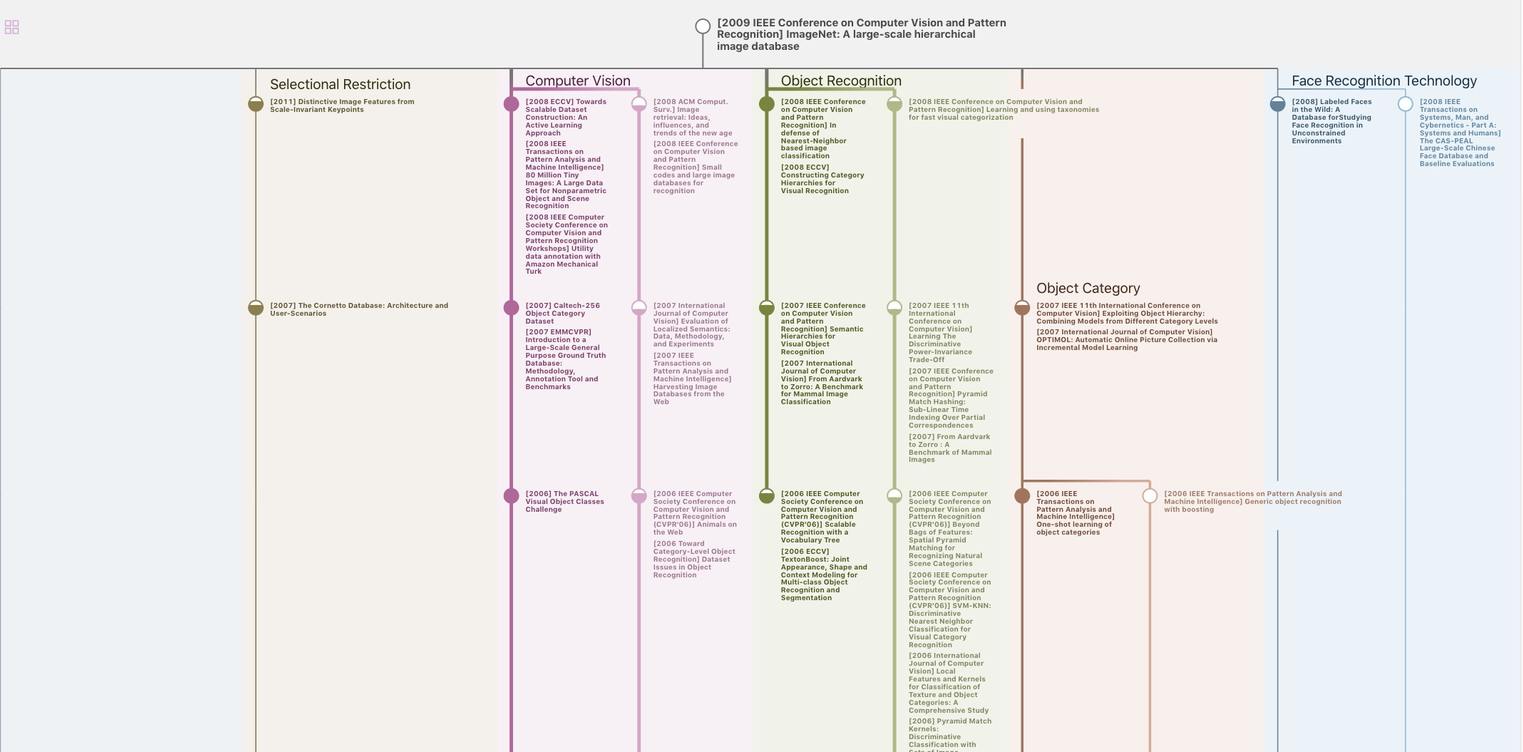
生成溯源树,研究论文发展脉络
Chat Paper
正在生成论文摘要