A Probabilistic Crowd-AI Framework for Reducing Uncertainty in Postdisaster Building Damage Assessment
JOURNAL OF ENGINEERING MECHANICS(2023)
摘要
Damage assessment of the built infrastructure forms a critical step in post-disaster response as it is necessary for estimating the severity and extent of the disaster impact, thereby ensuring effective and adequate recovery strategies. Contrary to traditional expert-driven approaches, recent trends show growing popularity in exploring more advanced alternate solutions, such as artificial intelligence (AI) and citizen science. One major current limitation, however, is the potential lack of reliability of these approaches. While recent efforts in the disaster research domain have successfully developed and demonstrated the use of AI and crowdsourcing-based solutions for large-scale post-disaster damage assessment, the inherent uncertainty associated with the adoption of such techniques for complicated subjective and expert-reliant tasks still hampers their practical implementation. This study aims to address this issue by reducing the uncertainty and increasing the consistency in post-disaster damage assessment by developing a novel crowd-AI framework that leverages the collective power of AI with citizen science. The framework comprises two modules: (1) an uncertainty-aware AI-assisted building damage classification module; and (2) a crowd-based probabilistic module for participatory damage assessment. Mainly, the framework uses AI predictions and the underlying uncertainty as prior knowledge in a Bayesian setting to achieve an enhanced crowd-based damage assessment. This paper presents a case study and validates that this innovative crowd-AI approach can reduce the uncertainty by as much as 83%, depending on the end-user's uncertainty tolerance setting.
更多查看译文
关键词
Damage assessment,Citizen science,Uncertainty quantification,Artificial intelligence (AI),Human-AI partnership
AI 理解论文
溯源树
样例
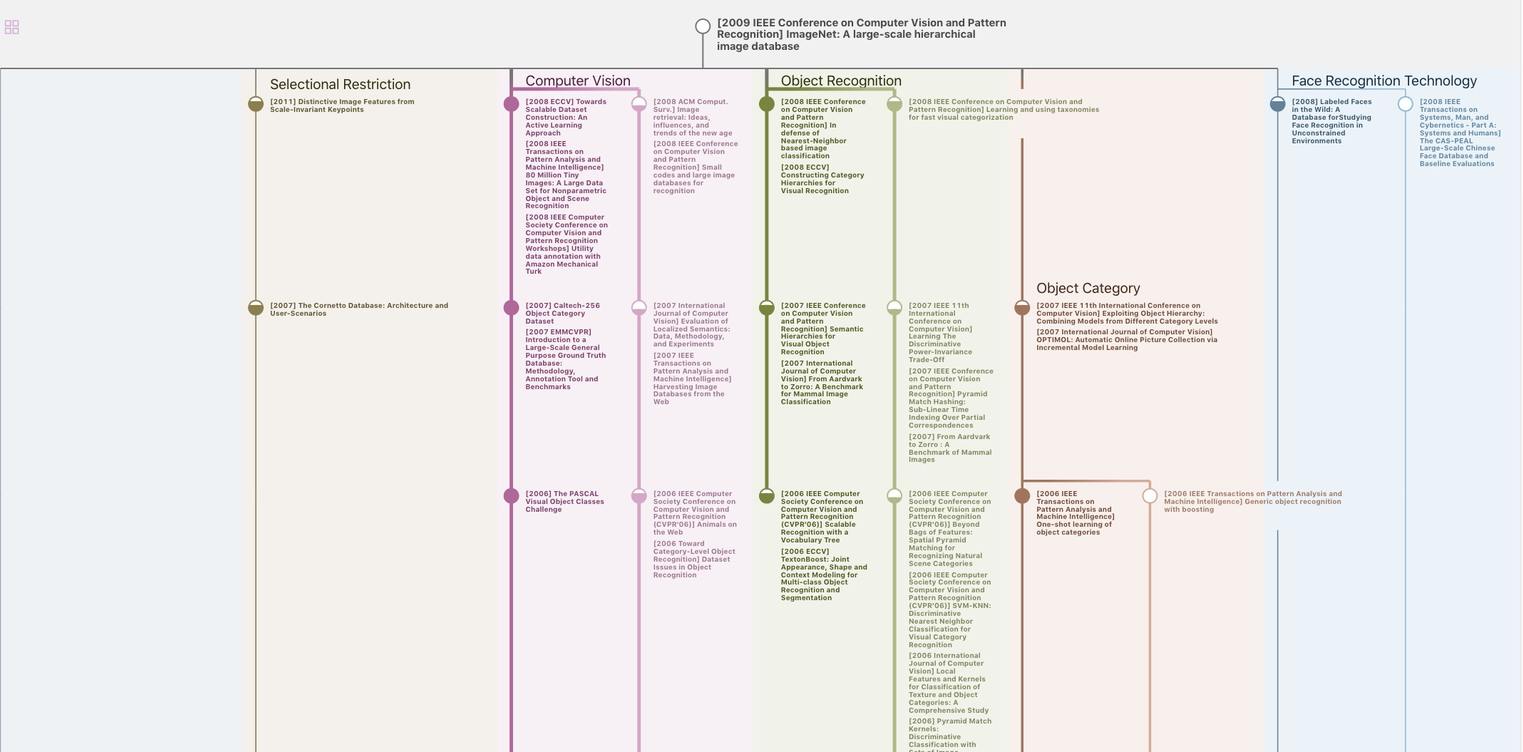
生成溯源树,研究论文发展脉络
Chat Paper
正在生成论文摘要