Online Learning under Budget and ROI Constraints and Applications to Bidding in Non-Truthful Auctions
arXiv (Cornell University)(2023)
摘要
We study online learning problems in which a decision maker has to make a sequence of costly decisions, with the goal of maximizing their expected reward while adhering to budget and return-on-investment (ROI) constraints. Previous work requires the decision maker to know beforehand some specific parameters related to the degree of strict feasibility of the offline problem. Moreover, when inputs are adversarial, it requires the existence of a strictly feasible solution to the offline optimization problem at each round. Both requirements are unrealistic for practical applications such as bidding in online ad auctions. We propose a best-of-both-worlds primal-dual framework which circumvents both assumptions by exploiting the notion of interval regret, providing guarantees under both stochastic and adversarial inputs. Our proof techniques can be applied to both input models with minimal modifications, thereby providing a unified perspective on the two problems. Finally, we show how to instantiate the framework to optimally bid in various mechanisms of practical relevance, such as first- and second-price auctions.
更多查看译文
关键词
bidding,learning,roi constraints,budget,non-truthful
AI 理解论文
溯源树
样例
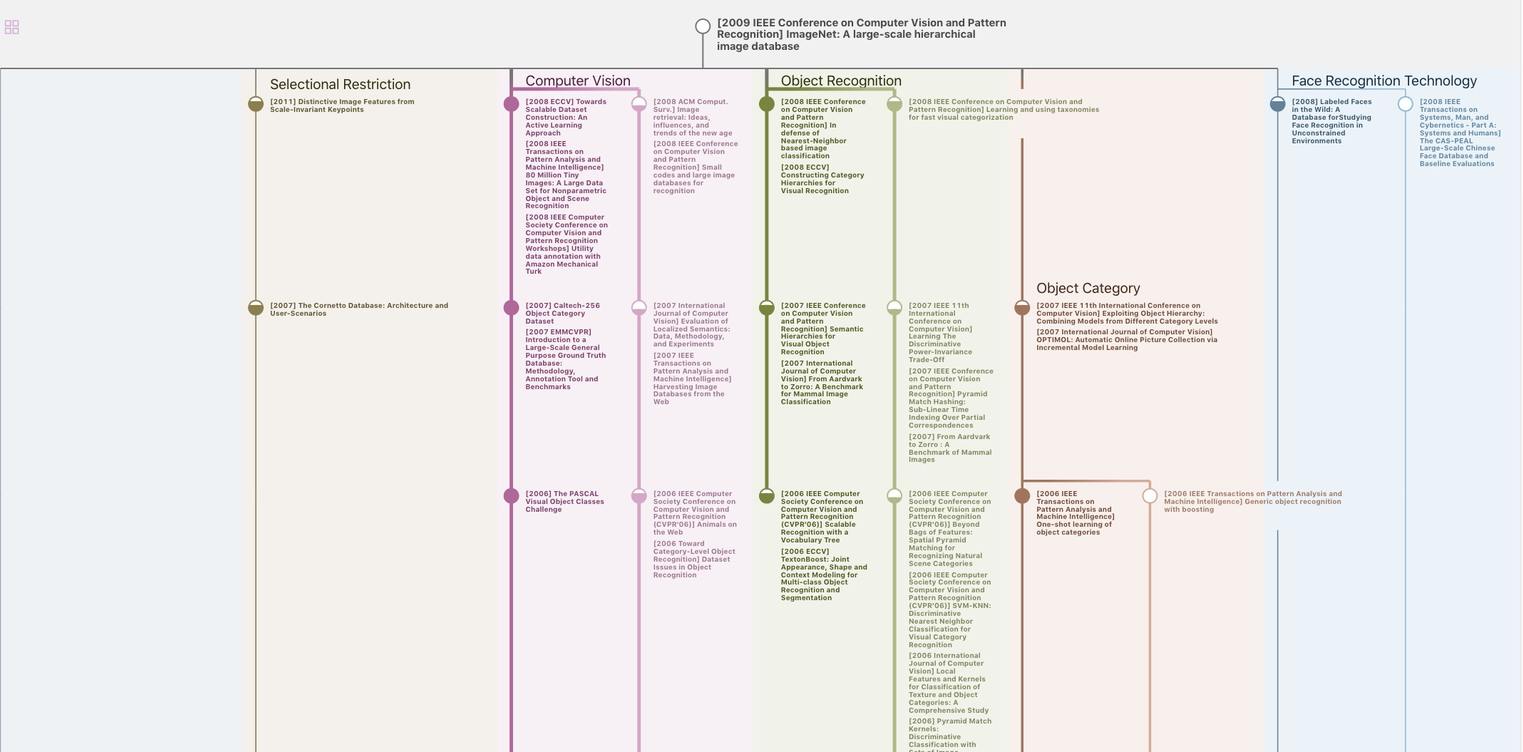
生成溯源树,研究论文发展脉络
Chat Paper
正在生成论文摘要