Understanding Reconstruction Attacks with the Neural Tangent Kernel and Dataset Distillation
arXiv (Cornell University)(2023)
摘要
Modern deep learning requires large volumes of data, which could contain sensitive or private information that cannot be leaked. Recent work has shown for homogeneous neural networks a large portion of this training data could be reconstructed with only access to the trained network parameters. While the attack was shown to work empirically, there exists little formal understanding of its effective regime which datapoints are susceptible to reconstruction. In this work, we first build a stronger version of the dataset reconstruction attack and show how it can provably recover the \emph{entire training set} in the infinite width regime. We then empirically study the characteristics of this attack on two-layer networks and reveal that its success heavily depends on deviations from the frozen infinite-width Neural Tangent Kernel limit. Next, we study the nature of easily-reconstructed images. We show that both theoretically and empirically, reconstructed images tend to "outliers" in the dataset, and that these reconstruction attacks can be used for \textit{dataset distillation}, that is, we can retrain on reconstructed images and obtain high predictive accuracy.
更多查看译文
关键词
Dataset Distillation,Reconstruction Attacks,Neural Tangent Kernel
AI 理解论文
溯源树
样例
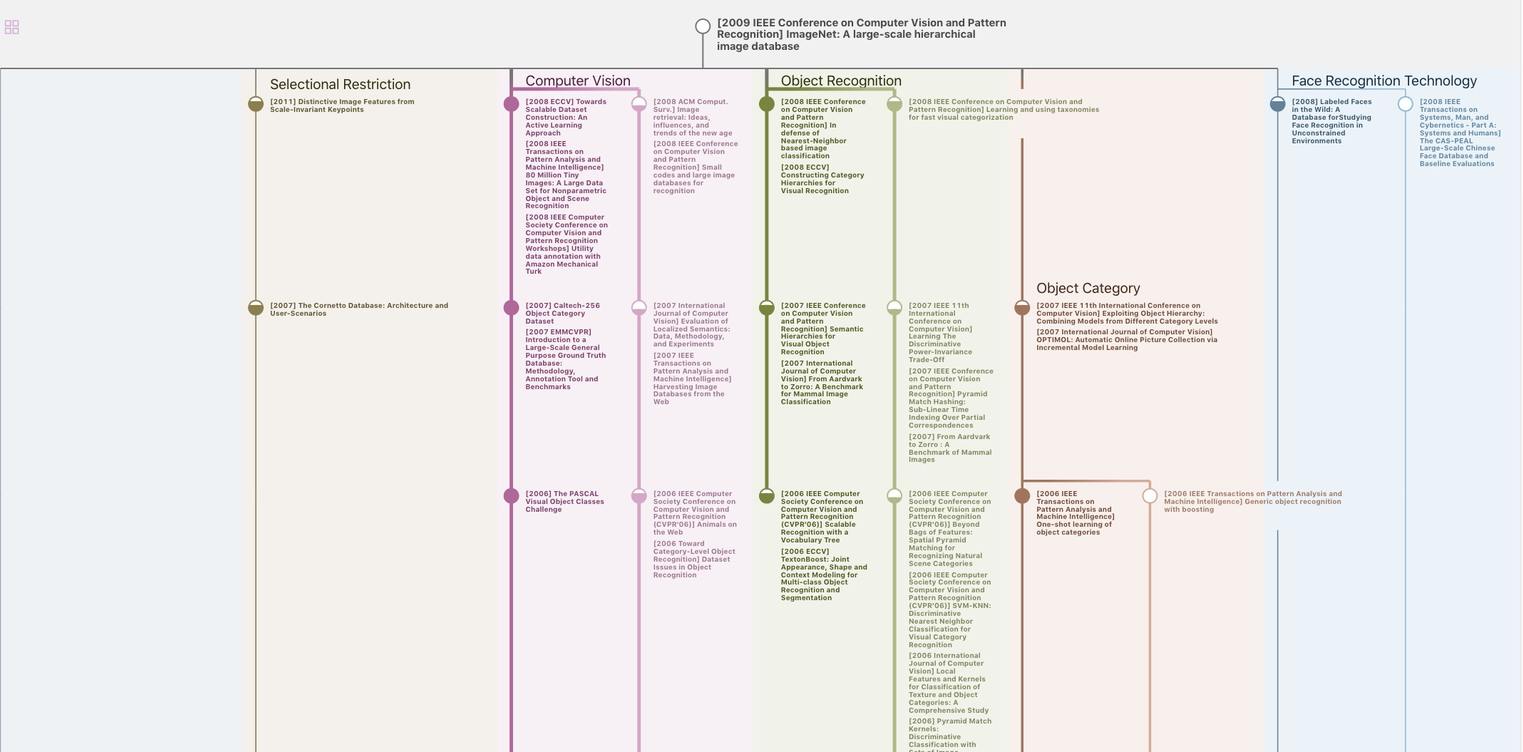
生成溯源树,研究论文发展脉络
Chat Paper
正在生成论文摘要