Abstract 3032: A novel deep learning pipeline for early detection of colorectal cancer and colorectal adenoma using gut microbiome data
Cancer Research(2023)
摘要
Abstract Many studies have shown the associations between colorectal cancer (CRC) and gut microbiome. The deep learning models have the potential to detect CRC earlier than the conventional stool screening test. However, the results are very inconsistent, which impedes the application of prediction using gut microbiomes as biomarkers. Therefore, this study aims to construct a novel deep learning (DL) pipeline to better classify CRC, colorectal adenoma, and healthy groups using microbiome data. Tissue or stool samples with sequence data and/or taxonomy profiles from 16 studies were collected from the NCBI SRA database or supplementary data provided in studies. Tumor-adjacent samples were excluded because of highly related to tumor samples. Sequence data were quality controlled and taxonomy assigned by EasyMAP based on the QIIME2 pipeline. All taxonomy profiles were merged and normalized to relative abundance. In total, 136 CRC-associated genera were collected from 38 different region studies. Among the CRC-associated genera, 98 ones with high prevalence were selected as CRC biomarkers. Taxonomy profiles were feature-selected by the CRC biomarkers. A phylogenetic tree of 98 CRC biomarkers was constructed by ete3 package and NCBI taxonomy database. Features in taxonomy profiles were sorted and converted into a 10x10 array by phylogenetic-based order. Samples labeled as healthy, adenoma, or CRC were randomly split into train, validation, and test datasets by 8:1:1. The 2D convolutional neural network DL model was trained with a feature array of the samples in the training dataset. Model performance was evaluated by the test dataset. Moreover, Saliency map was calculated to identify highly contributed features. Our results showed that, by combining the tissue and stool data, the DL model illustrated excellent performance with 81% AUC and 60% accuracy for the test dataset in classifying health, adenoma, and CRC groups. Two-class DL model performed even higher with 85% AUC and 78% accuracy in classifying health and CRC groups. For a model with only stool samples, the model in classifying health and CRC groups achieved comparable results with 84% AUC and 76% accuracy. Finally, CRC biomarkers that highly contributed to the DL model were identified by saliency map. In summary, we developed a new pipeline for CRC classification using 16s rRNA gut microbiome data and identified CRC-specific gut microbiome genera. The pipeline and biomarkers could be used as a non-invasive tool for the early detection of CRC. Citation Format: Nai-Shun Liao, Yuan-Mao Hung, Yi-Jian Tsai, Nam Nhut Phan, Pei-Chun Chen, Liang-Chuan Lai, Mong-Hsun Tsai, Tzu-Pin Lu, Eric Y. Chuang. A novel deep learning pipeline for early detection of colorectal cancer and colorectal adenoma using gut microbiome data [abstract]. In: Proceedings of the American Association for Cancer Research Annual Meeting 2023; Part 1 (Regular and Invited Abstracts); 2023 Apr 14-19; Orlando, FL. Philadelphia (PA): AACR; Cancer Res 2023;83(7_Suppl):Abstract nr 3032.
更多查看译文
关键词
gut microbiome data,novel deep learning pipeline,colorectal cancer,deep learning
AI 理解论文
溯源树
样例
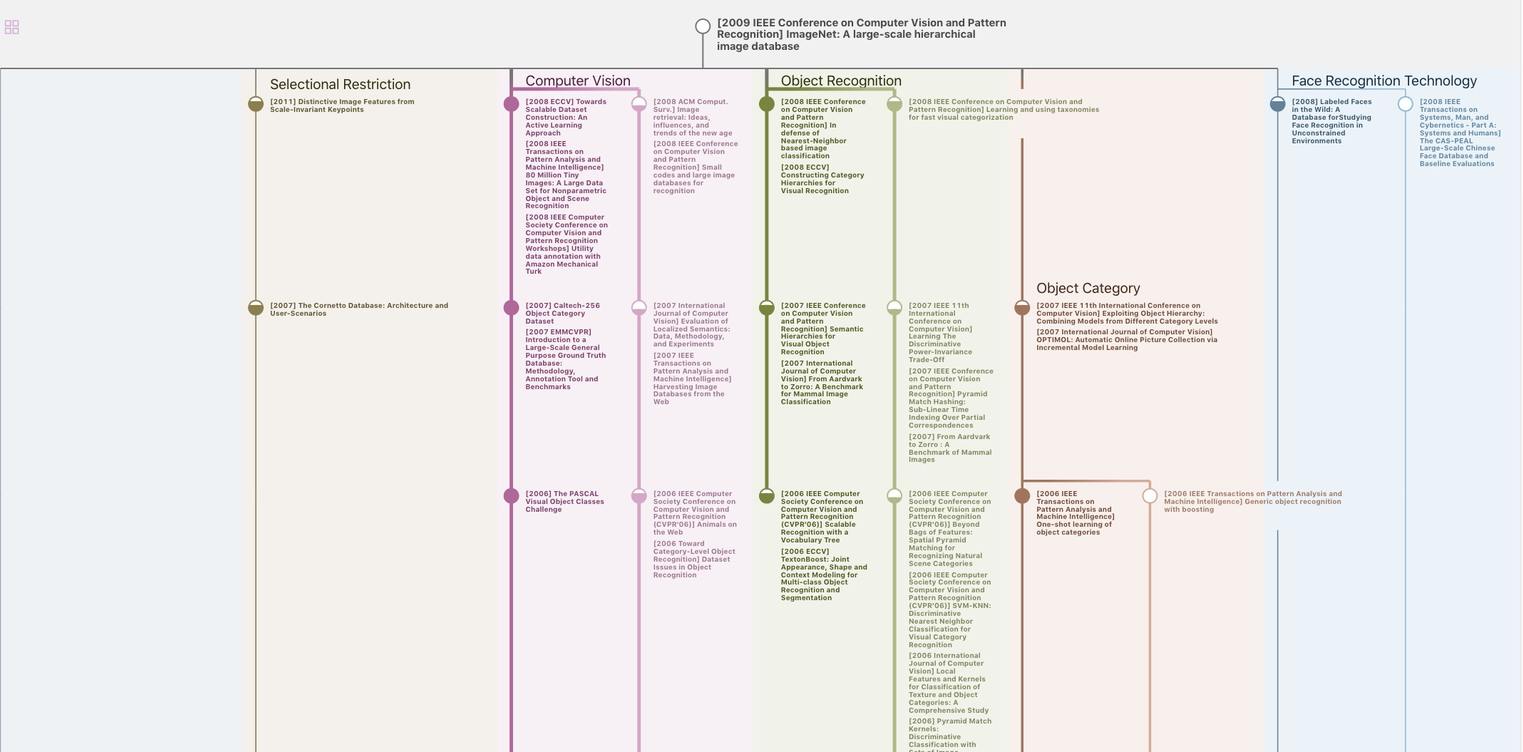
生成溯源树,研究论文发展脉络
Chat Paper
正在生成论文摘要