Quantum Conformal Prediction for Reliable Uncertainty Quantification in Quantum Machine Learning
arXiv (Cornell University)(2023)
摘要
In this work, we aim at augmenting the decisions output by quantum models with "error bars" that provide finite-sample coverage guarantees. Quantum models implement implicit probabilistic predictors that produce multiple random decisions for each input through measurement shots. Randomness arises not only from the inherent stochasticity of quantum measurements, but also from quantum gate noise and quantum measurement noise caused by noisy hardware. Furthermore, quantum noise may be correlated across shots and it may present drifts in time. This paper proposes to leverage such randomness to define prediction sets for both classification and regression that provably capture the uncertainty of the model. The approach builds on probabilistic conformal prediction (PCP), while accounting for the unique features of quantum models. Among the key technical innovations, we introduce a new general class of non-conformity scores that address the presence of quantum noise, including possible drifts. Experimental results, using both simulators and current quantum computers, confirm the theoretical calibration guarantees of the proposed framework.
更多查看译文
关键词
quantum machine learning,reliable uncertainty quantification,prediction
AI 理解论文
溯源树
样例
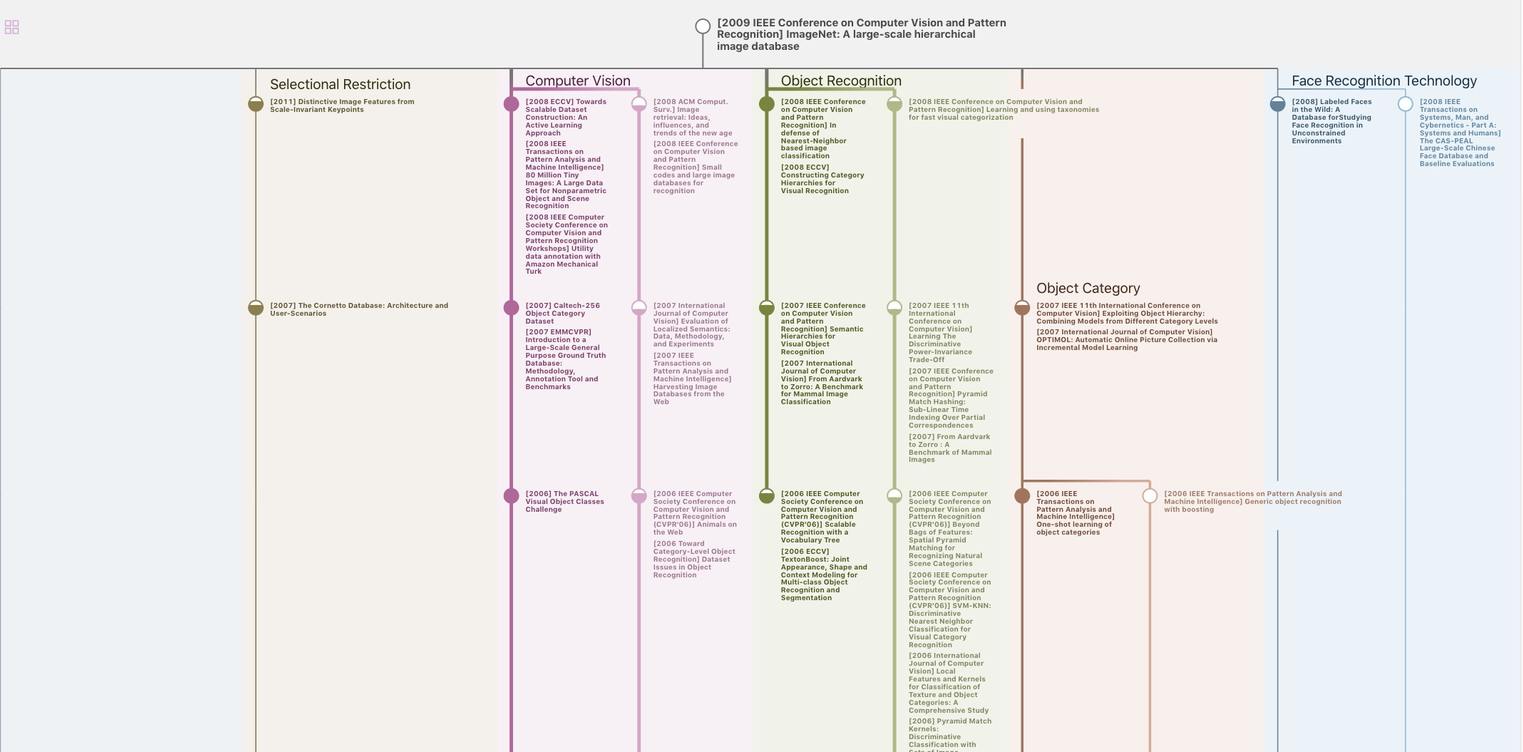
生成溯源树,研究论文发展脉络
Chat Paper
正在生成论文摘要