Abstract LB181: Infer cancer cell gene dependency in multiple myeloma using causal AI in-silico patient model
Cancer Research(2023)
摘要
Abstract Recent advances in artificial intelligence (AI) and availability of multimodal patient datasets have enabled the construction of complex network models to derive disease molecular mechanisms and predict the impact of therapeutic intervention. However, observational datasets are commonly affected by confounding factors making causal interpretation challenging. Causal inference network methods are particularly suited to facilitate therapeutic intervention studies by inferring the causal structure from sufficiently detailed multi-omic molecular data. The learned models enable in-silico loss-of-function screening experiments on patient data by using counterfactual simulation to reveal the impact of a gene loss in a disease model. These models enhance gene dependency characterization and the design of advanced therapeutic interventions. In this study, we developed an in-silico multiple myeloma (MM) patient causal model of overall survival (OS) based on transcriptomic expression, clinical, and genomic alteration data from Multiple Myeloma Research Foundation (MMRF) CoMMpass dataset (IA19). After filtering for data quality and availability, we included 516 patients, with 60% being hyperdiploid. We sampled Bayesian networks using Markov Chain Monte Carlo simulation to infer probabilistic causal relationships between network components that influence overall survival. We then simulated synthetic knock downs of those genes where a path exists to overall survival with posterior probability of at least 0.25. Next, we compared CRISPR-SpCas9 cancer dependency data from DepMap (version 22Q2) for multiple myeloma cell lines against genes predicted to be causal (causal genes) for overall survival. Last, we examined the causal genes that are non-dependent in MM cell lines for upstream genomic alterations to determine if specific patient genomic contexts are affecting the results. We identified 102 causal genes, including non-coding RNA genes (n=23), driving overall survival. Among them, 70% (56/79, p=2.2e-16, OR=9.5) of the coding genes were found to be MM-dependent in DepMap, with 44 common essential, 7 strongly selective and 5 weakly selective genes. From 23 genes identified as causal and not known to be MM-dependent, 20 (87%) were selective in other cancer lineages and all of them (23/23) had consistent upstream genomic alterations driving their expression. Causal genes identified from AI-driven in-silico experiments to predict overall survival were strongly enriched for known dependent genes from DepMap. Furthermore, we identified causal genes that may be dependent in unique patient genomic contexts. This demonstrates in-silico AI causal modelling is a powerful tool for exploring cancer cell vulnerability directly from patient data to advance target discovery. Citation Format: Brandon Nathasingh, Derek Walkama, Laurel Mayhew, Kendall Loh, Jeanne Latourelle, Bruce W. Church, Yaoyu E. Wang. Infer cancer cell gene dependency in multiple myeloma using causal AI in-silico patient model [abstract]. In: Proceedings of the American Association for Cancer Research Annual Meeting 2023; Part 2 (Clinical Trials and Late-Breaking Research); 2023 Apr 14-19; Orlando, FL. Philadelphia (PA): AACR; Cancer Res 2023;83(8_Suppl):Abstract nr LB181.
更多查看译文
关键词
multiple myeloma,cancer,gene,causal ai,in-silico
AI 理解论文
溯源树
样例
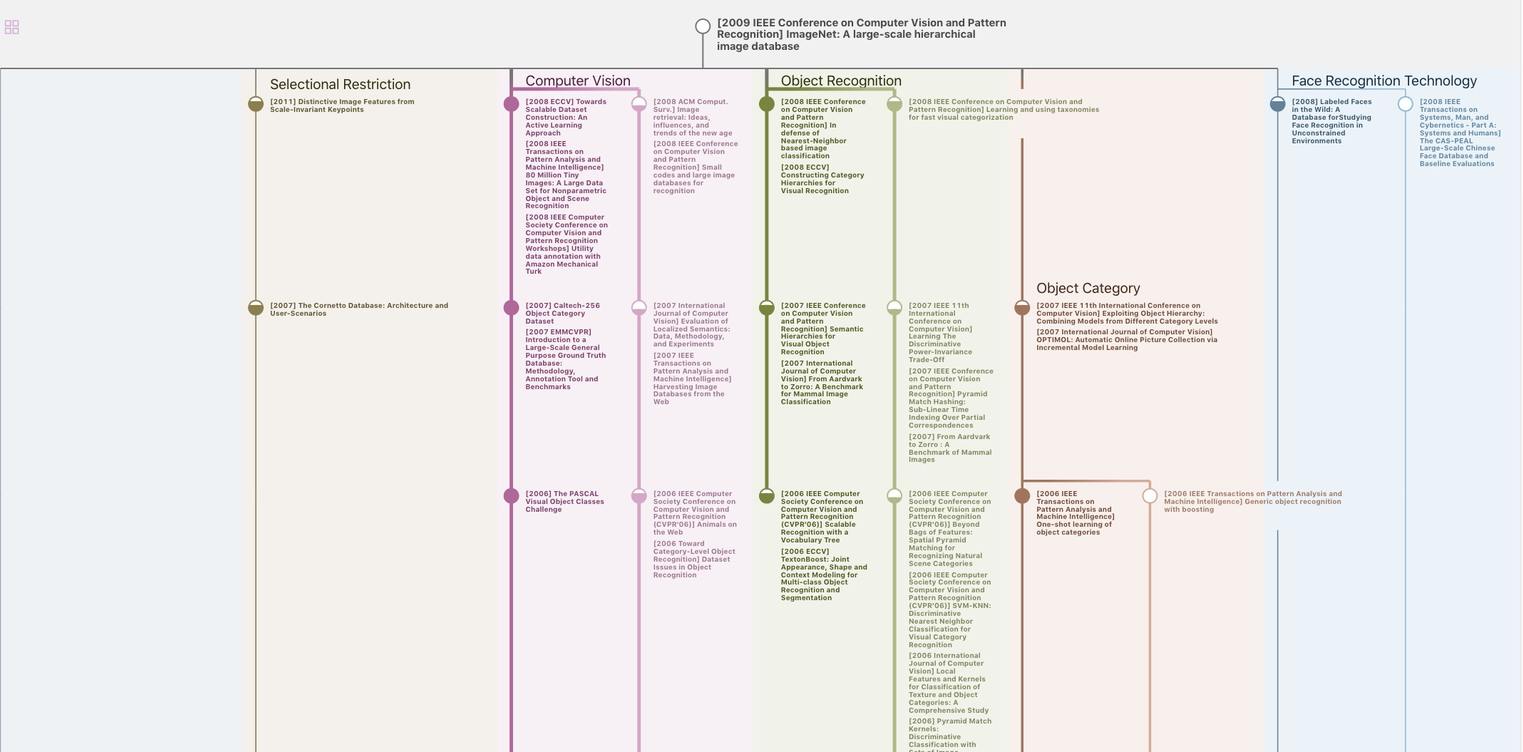
生成溯源树,研究论文发展脉络
Chat Paper
正在生成论文摘要