Bad data identification for power systems state estimation based on data-driven and interval analysis
Electric Power Systems Research(2023)
摘要
A bad data identification method based on data-driven and interval analysis is proposed to address the identification problem when state estimator malfunction happens. The proposed method combines the industry allowable error standard with affine arithmetic-based interval power flow to generate interval samples (INTSs) and then searches for an INTS with the maximum normal measurements to identify bad data. Compared with the identification method based on state estimation, the method avoids the impact of residual smearing effect on threshold setting and identification accuracy. Compared with the data-driven identification methods based on Monte Carlo samples, this method transforms a large number of nonlinear power flow calculations into a small number of interval linear programming problems and resolves the tradeoff of sample granularity between identification accuracy and identification speed. The numerical tests on IEEE-14, 30, 69, 118, and 300 cases demonstrated that this method has higher accuracy and speed. The bad data identification of a 110 kV distribution network snapshot in a Chinese city proves the feasibility of the method on the actual data.
更多查看译文
关键词
power systems state estimation,bad data identification,data-driven
AI 理解论文
溯源树
样例
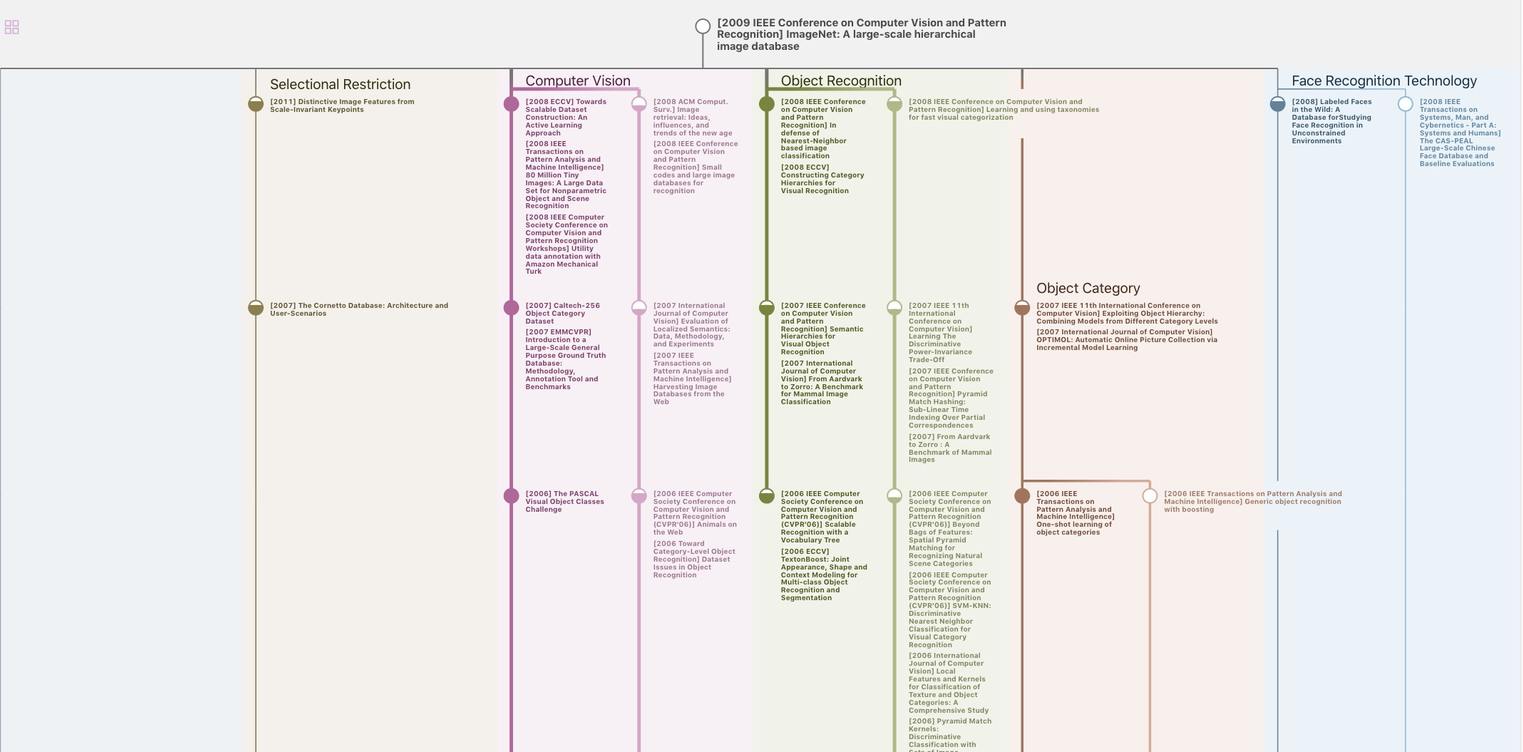
生成溯源树,研究论文发展脉络
Chat Paper
正在生成论文摘要