A Deep Learning Framework to Identify Remedial Action Schemes Against False Data Injection Cyberattacks Targeting Smart Power Systems
IEEE TRANSACTIONS ON INDUSTRIAL INFORMATICS(2023)
摘要
This article proposes a remedial action scheme (RAS) based on the concept of deep learning to mitigate the impacts of false data injection (FDI) cyberattacks on smart power systems. As a prerequisite of such a RAS, power system operator is being in attacker's shoe to scrutinize different scenarios of cyberattacks. In design of the RAS, long short-term memory (LSTM) cells have been integrated into a deep recurrent neural network (DRNN) to effectively process the data of an intelligent archive framework (IAF), identifying the proper reaction mechanisms. Power flow analysis has been considered to examine the interdependence between transmission/distribution sectors to react to the cyberattacks for which similar pre-investigated remedial actions have not been saved in the IAF. Effectiveness of the proposed RAS is validated on two IEEE transmission/distribution systems, where consequences of FDI cyberattacks are reduced by 30% in case of experiencing attacks, which are not pre-investigated by system operator.
更多查看译文
关键词
Archive,congestion,flexible AC transmission system (FACTS),deep learning,false data injection (FDI),remedial action scheme (RAS),voltage violation
AI 理解论文
溯源树
样例
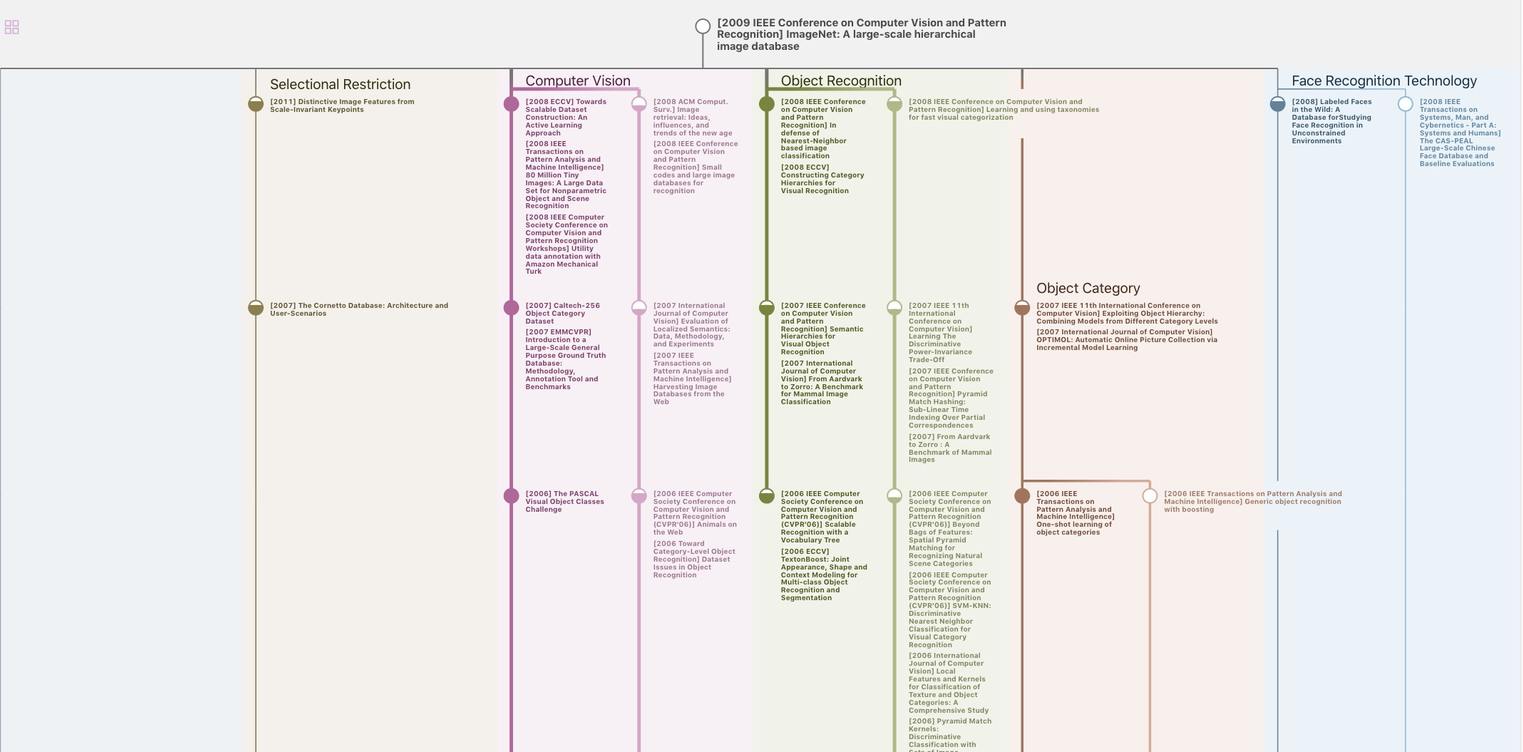
生成溯源树,研究论文发展脉络
Chat Paper
正在生成论文摘要