Personalized Privacy Preservation in Consumer Mobile Trajectories
Information Systems Research(2024)
摘要
The use of mobile technologies to collect and analyze consumer location data has created a multi-billion-dollar ecosystem with various stakeholders. However, this ecosystem also presents privacy risks to consumers. To address this issue, data aggregators can implement a privacy preserving framework that balances privacy risks to consumers with data utilities for advertisers. The proposed framework is personalized and flexible, allowing for quantification of personalized privacy risks and data obfuscation to reduce these risks. It can accommodate a variety of risks, utilities, and trade-offs between the two. The framework was validated on one million consumer location trajectories, revealing potential privacy risks in the absence of data obfuscation. Machine learning methods are used to demonstrate the effectiveness of the proposed framework which outperformed ten baselines from the latest literature, significantly reducing each consumer’s privacy risk while preserving advertiser utility. As the use of location big data continues to grow, this research offers a necessary framework to balance privacy risks and data utilities, sustain a secure and self-governing ecosystem, and ensure the protection of consumers’ personal data. Ubiquitous mobile technologies have been producing massive swaths of consumer location data, giving rise to an elaborate multibillion-dollar ecosystem. In this ecosystem, some consumers share personal data in exchange for economic benefits, including personalized recommendations; data aggregators curate and monetize data by sharing data with advertisers, and advertisers often utilize such data for location-based marketing. While these various entities can benefit from such data sharing, privacy risks can prevail. This creates an opportunity for data aggregators to implement an effective privacy preserving framework to balance potential privacy risks to consumers and data utilities to advertisers before sharing data with advertisers. We hence propose a personalized and flexible framework that quantifies personalized privacy risks, performs personalized data obfuscation, and flexibly accommodates a variety of risks, utilities, and acceptable levels of risk-utility trade-off. Leveraging machine learning methods, we illustrate the power of the framework with two privacy risks and two utilities. Validating the framework on one million consumer trajectories, we demonstrate potential privacy risks in the absence of data obfuscation. Outperforming ten baselines from the latest literature, the proposed framework significantly reduces each consumer’s privacy risk while preserving an advertiser’s utility. As industries increasingly unleash the power of location big data, this research offers an imperatively needed framework to balance privacy risks and data utilities, and to sustain a secure and self-governing multibillion-dollar location ecosystem. History: Olivia Sheng, Senior Editor; Xiaobai Li, Associate Editor. Supplemental Material: The e-companion is available at https://doi.org/10.1287/isre.2023.1227 .
更多查看译文
关键词
consumer privacy,privacy preservation data publishing,mobile location data,machine learning,location-based marketing
AI 理解论文
溯源树
样例
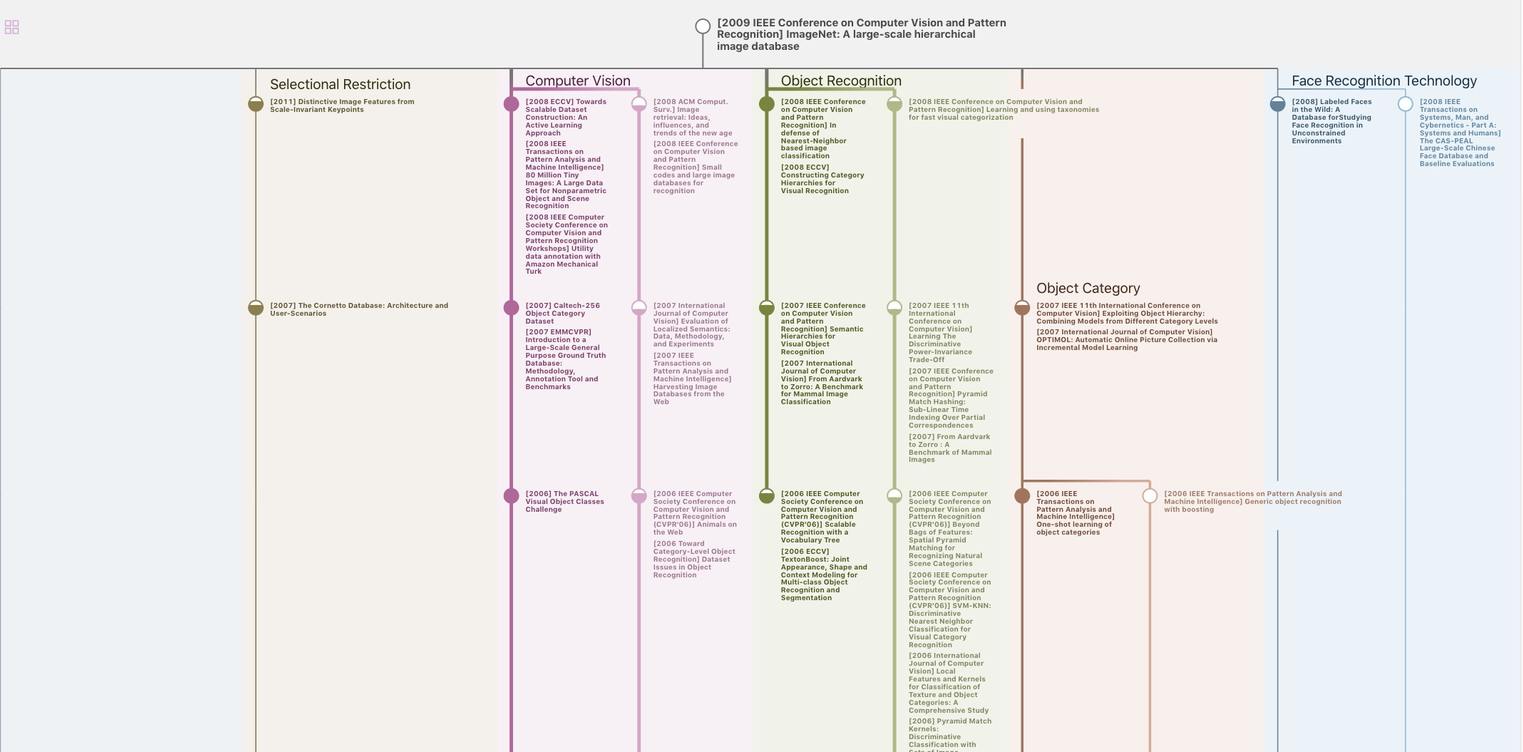
生成溯源树,研究论文发展脉络
Chat Paper
正在生成论文摘要