The AIRI Plug-and-play Algorithm for Image Reconstruction in Radio-Interferometry: Variations and Robustness
arxiv(2023)
摘要
Plug-and-Play (PnP) algorithms are appealing alternatives to proximal algorithms when solving inverse imaging problems. By learning a Deep Neural Network (DNN) denoiser behaving as a proximal operator, one waives the computational complexity of optimisation algorithms induced by sophisticated image priors, and the sub-optimality of handcrafted priors compared to DNNs. Such features are highly desirable in radio-interferometric (RI) imaging, where precision and scalability of the image reconstruction process are key. In previous work, we introduced AIRI, PnP counterpart to the unconstrained variant of the SARA optimisation algorithm, relying on a forward-backward algorithmic backbone. Here, we introduce variations of AIRI towards a more general and robust PnP paradigm in RI imaging. Firstly, we show that the AIRI denoisers can be used without any alteration to instantiate a PnP counterpart to the constrained SARA optimisation algorithm itself, relying on a primal-dual forward-backward algorithmic backbone, thus extending the remit of the AIRI paradigm. Secondly, we show that AIRI algorithms are robust to strong variations in the nature of the training dataset, with denoisers trained on medical images yielding similar reconstruction quality to those trained on astronomical images. Thirdly, we develop a functionality to quantify the model uncertainty introduced by the randomness in the training process. We validate the image reconstruction and uncertainty quantification functionality of AIRI algorithms against the SARA family and CLEAN, both in simulation and on real data of the ESO 137-006 galaxy acquired with the MeerKAT telescope. AIRI code is available in the BASPLib code library on GitHub.
更多查看译文
AI 理解论文
溯源树
样例
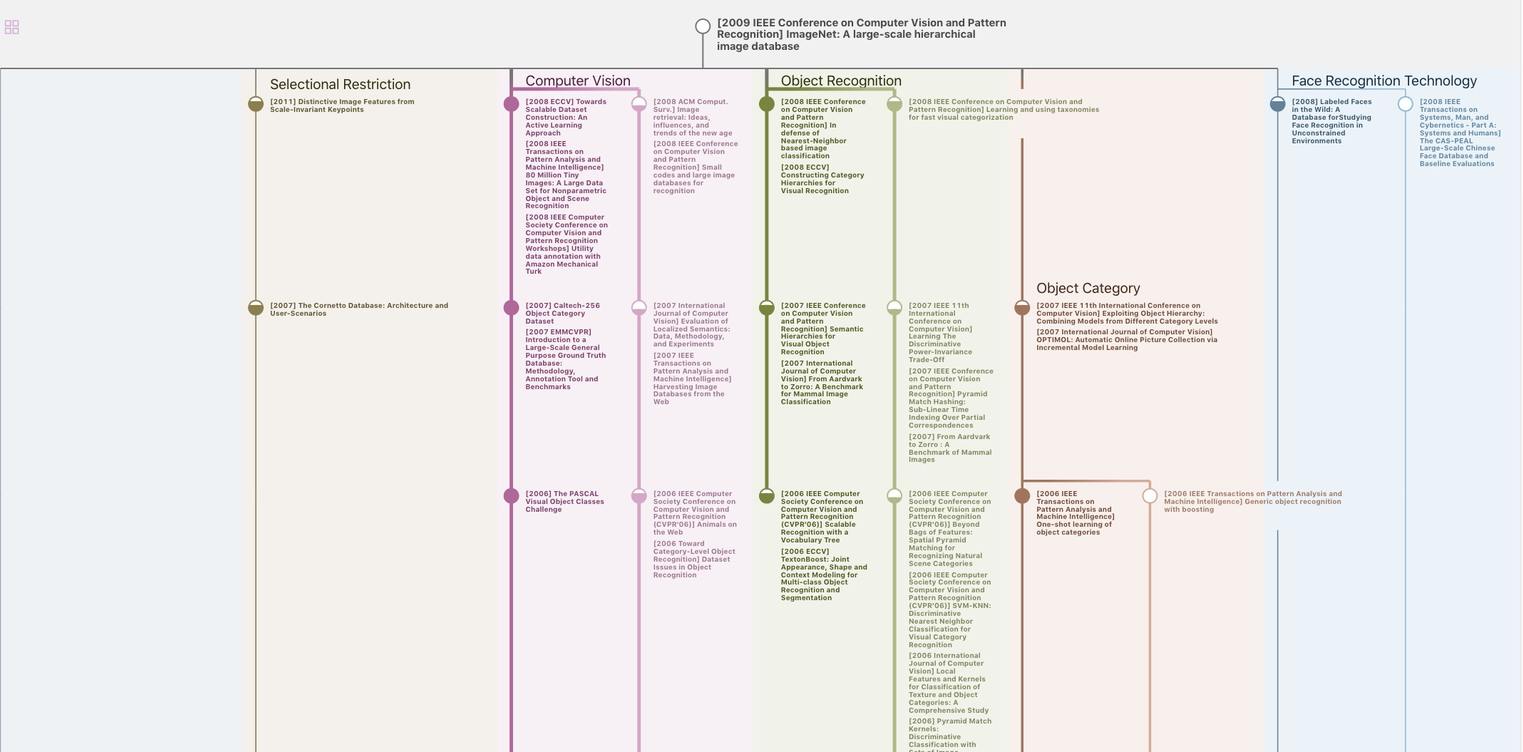
生成溯源树,研究论文发展脉络
Chat Paper
正在生成论文摘要